A multilayered block network model to forecast large dynamic transportation graphs: An application to US air transport
TRANSPORTATION RESEARCH PART C-EMERGING TECHNOLOGIES(2022)
摘要
Dynamic transportation networks have been analyzed for years by means of static graph-based indicators in order to study the temporal evolution of relevant network components, and to reveal complex dependencies that would not be easily detected by a direct inspection of the data. This paper presents a state-of-the-art probabilistic latent network model to forecast multilayer dynamic graphs that are increasingly common in transportation and proposes a community-based extension to reduce the computational burden. Flexible time series analysis is obtained by modeling the probability of edges between vertices through latent Gaussian processes. The models and Bayesian inference are illustrated on a sample of 10-year data from four major airlines within the US air transportation system. Results show how the estimated latent parameters from the models are related to the airlines' connectivity dynamics, and their ability to project the multilayer graph into the future for out-of-sample full network forecasts, while stochastic blockmodeling allows for the identification of relevant communities. Reliable network predictions would allow policy-makers to better understand the dynamics of the transport system, and help in their planning on e.g. route development, or the deployment of new regulations.
更多查看译文
关键词
Transportation networks, Multilayer graphs, Air transport, Machine learning
AI 理解论文
溯源树
样例
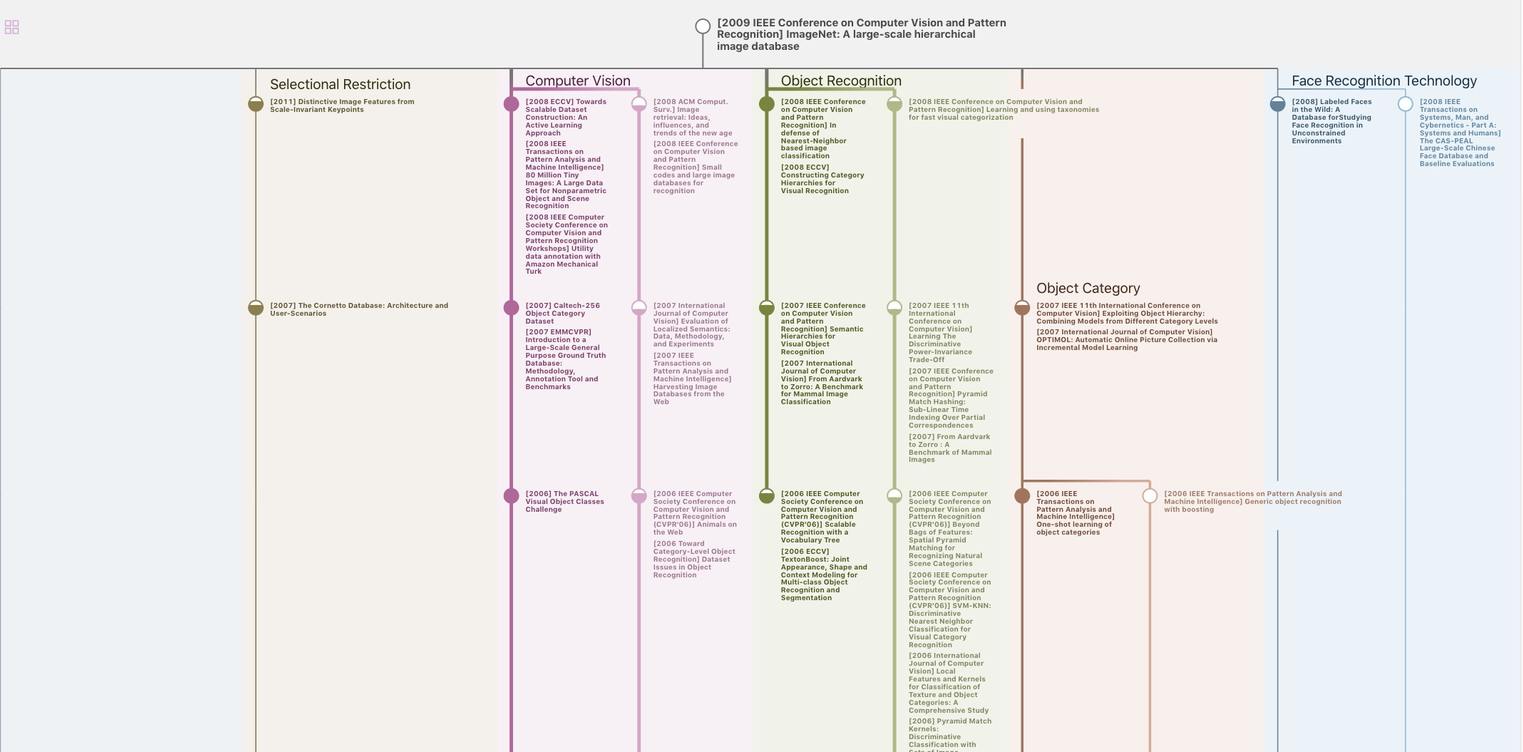
生成溯源树,研究论文发展脉络
Chat Paper
正在生成论文摘要