Selective Domain-Invariant Feature Alignment Network for Face Anti-Spoofing
IEEE TRANSACTIONS ON INFORMATION FORENSICS AND SECURITY(2021)
摘要
One primary challenge in face anti-spoofing refers to suffering a sharp performance drop in cross-domain scenes, where training and testing images are collected from different datasets. Recent methods have achieved promising results by aligning the features of all images among the available source domains. However, due to significant distribution discrepancies among non-face regions of all images, it is challenging to capture domain-invariant features for these regions. In this paper, we propose a novel Selective Domain-invariant Feature Alignment Network (SDFANet) for cross-domain face anti-spoofing, which aims to seek common feature representations by fully exploring the generalization of different regions of images. Different from previous works that align the whole features directly, the proposed SDFANet leverages multiple domain discriminators with the same architecture to balance the generalization of different regions of the all images. Specifically, we firstly design a multi-grained feature alignment network composed of a local-region and global-image alignment subnetworks to learn more generalized feature space for real faces. Besides, the domain adapter module, which aims to alleviate the large domain discrepancy with the help of the domain attention strategy, is adopted to facilitate the learning of our multi-grained feature alignment network. In addition, a multi-scale attention fusion module is designed in our feature generator to refine the different levels of features effectively. Experimental results show that the proposed SDFANet can greatly improve the generalization ability of face anti-spoofing, and that is superior to the existing methods.
更多查看译文
关键词
Faces, Face recognition, Testing, Feature extraction, Generators, Task analysis, Deep learning, Cross-domain face anti-spoofing, feature alignment network, domain adapter, adversarial learning
AI 理解论文
溯源树
样例
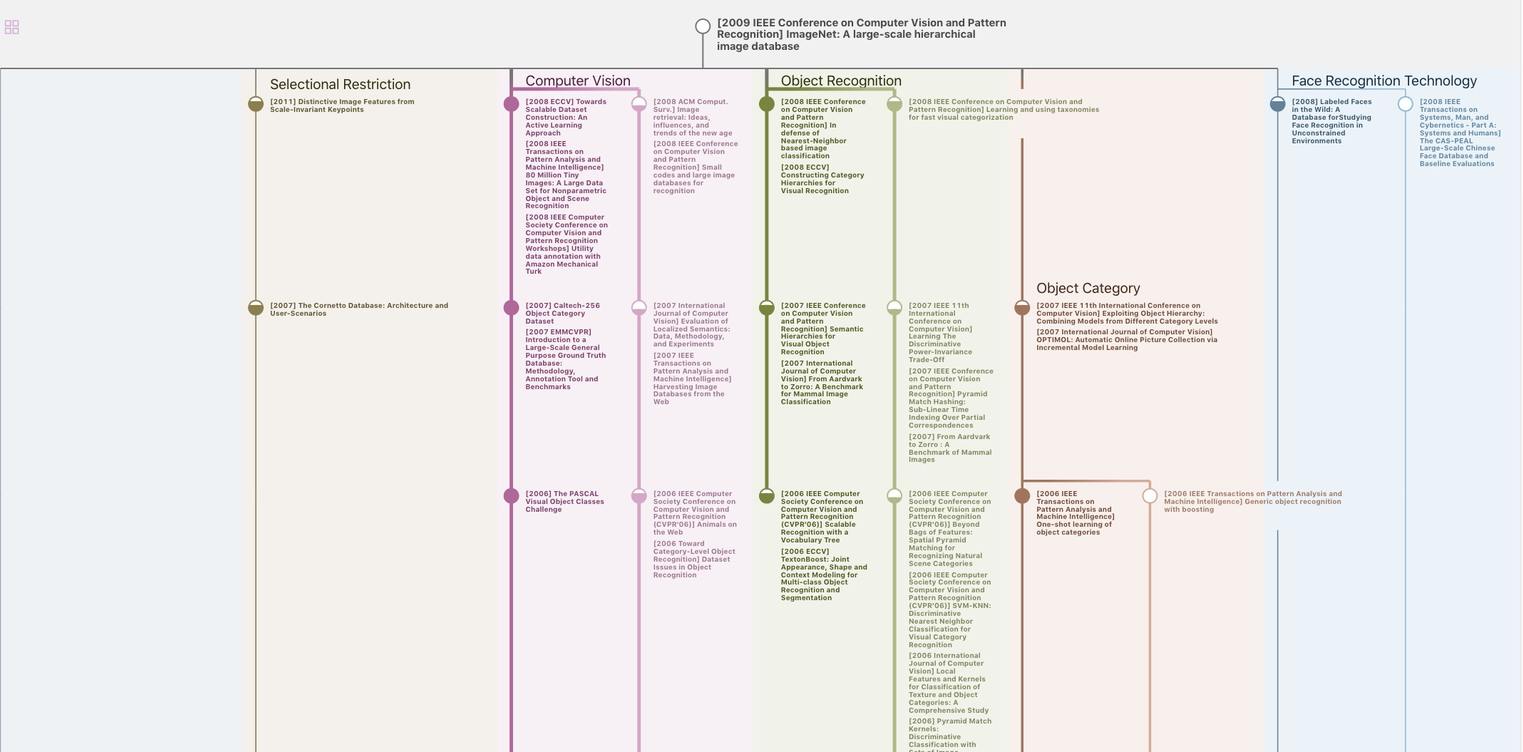
生成溯源树,研究论文发展脉络
Chat Paper
正在生成论文摘要