Comparative Analysis of Machine Learning Methods for Predicting Robotized Incremental Metal Sheet Forming Force
SENSORS(2022)
摘要
This paper proposes a method for extracting information from the parameters of a single point incremental forming (SPIF) process. The measurement of the forming force using this technology helps to avoid failures, identify optimal processes, and to implement routine control. Since forming forces are also dependent on the friction between the tool and the sheet metal, an innovative solution has been proposed to actively control the friction forces by modulating the vibrations that replace the environmentally unfriendly lubrication of contact surfaces. This study focuses on the influence of mechanical properties, process parameters and sheet thickness on the maximum forming force. Artificial Neural Network (ANN) and different machine learning (ML) algorithms have been applied to develop an efficient force prediction model. The predicted forces agreed reasonably well with the experimental results. Assuming that the variability of each input function is characterized by a normal distribution, sampling data were generated. The applicability of the models in an industrial environment is due to their relatively high performance and the ability to balance model bias and variance. The results indicate that ANN and Gaussian process regression (GPR) have been identified as the most efficient methods for developing forming force prediction models.
更多查看译文
关键词
incremental sheet forming,failure prevention,friction force,robotized manufacturing,prediction model
AI 理解论文
溯源树
样例
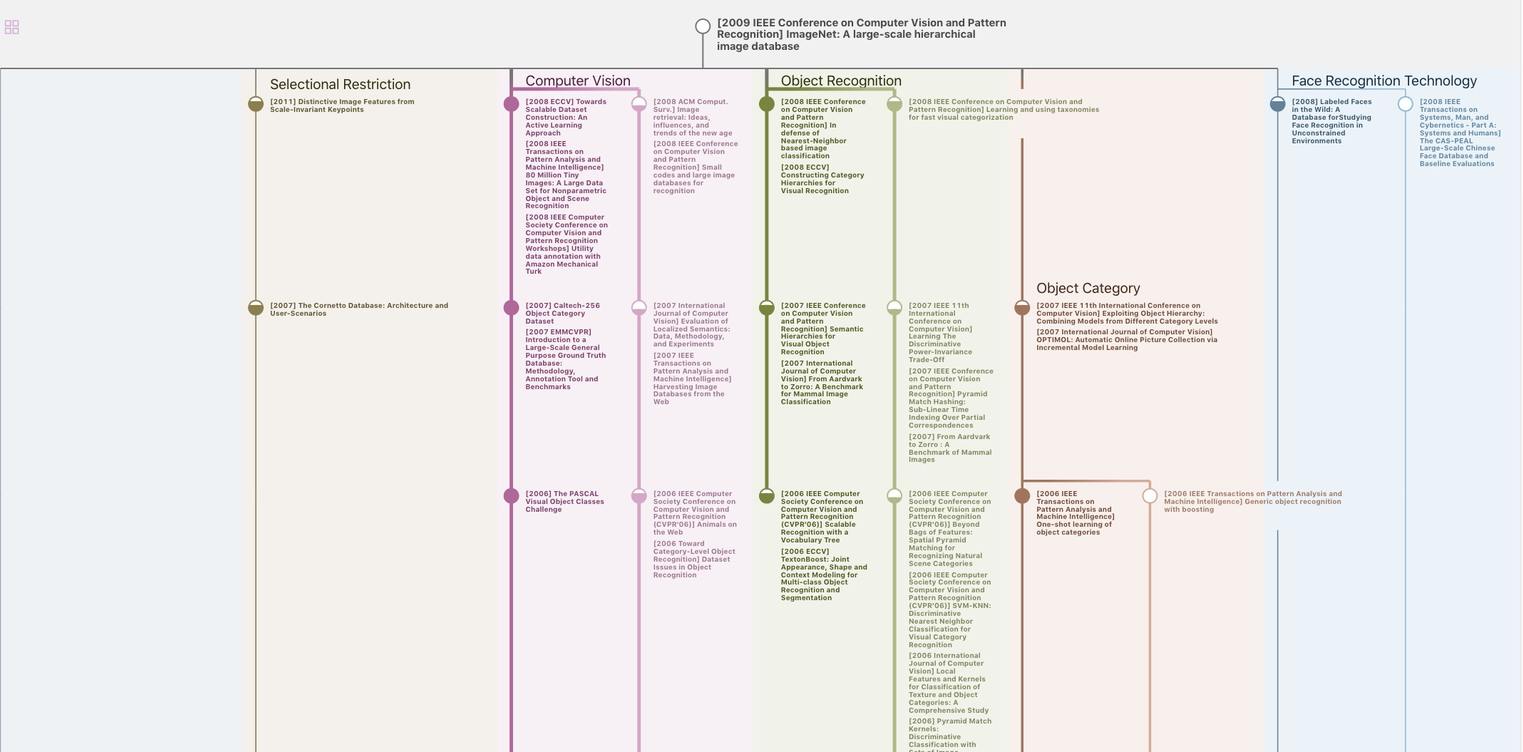
生成溯源树,研究论文发展脉络
Chat Paper
正在生成论文摘要