Anomaly Prediction for Wind Turbines Using an Autoencoder with Vibration Data Supported by Power-Curve Filtering
IEICE TRANSACTIONS ON INFORMATION AND SYSTEMS(2022)
摘要
The prediction of the malfunction timing of wind turbines is essential for maintaining the high profitability of the wind power generation industry. Studies have been conducted on machine learning methods that use condition monitoring system data, such as vibration data, and supervisory control and data acquisition (SCADA) data to detect and predict anomalies in wind turbines automatically. Autoencoder-based techniques that use unsupervised learning where the anomaly pattern is unknown have attracted significant interest in the area of anomaly detection and prediction. In particular, vibration data are considered useful because they include the changes that occur in the early stages of a malfunction. However, when autoencoder-based techniques are applied for prediction purposes, in the training process it is difficult to distinguish the difference between operating and non-operating condition data, which leads to the degradation of the prediction performance. In this letter, we propose a method in which both vibration data and SCADA data are utilized to improve the prediction performance, namely, a method that uses a power curve composed of active power and wind speed. We evaluated the method's performance using vibration and SCADA data obtained from an actual wind farm.
更多查看译文
关键词
anomaly prediction, autoencodet, vibration data, SCADA data, power-curve filtering
AI 理解论文
溯源树
样例
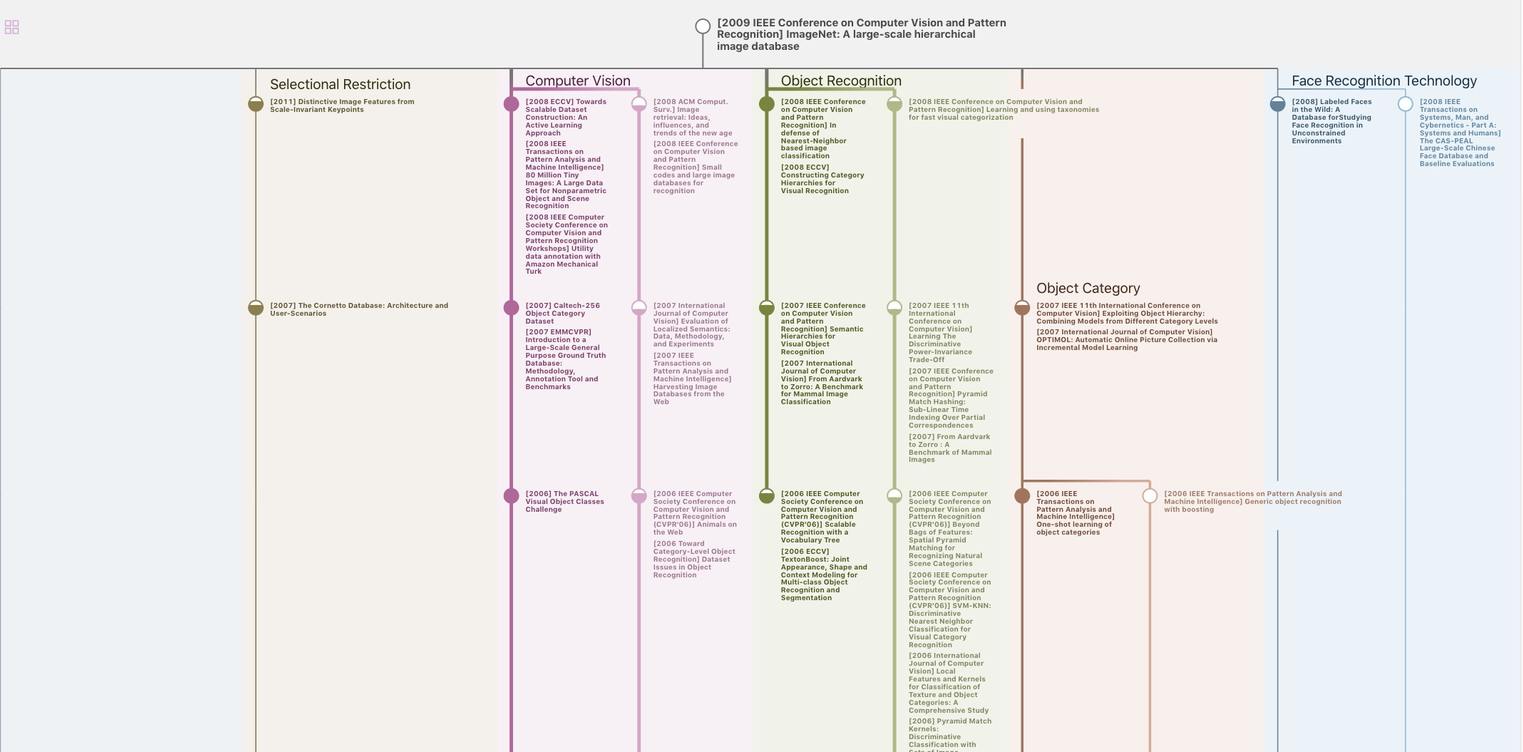
生成溯源树,研究论文发展脉络
Chat Paper
正在生成论文摘要