A novel hybrid model integrating residual structure and bi-directional long short-term memory network for tool wear monitoring
The International Journal of Advanced Manufacturing Technology(2022)
摘要
Tool wear monitoring in machine process is essential for quality assurance, efficiency improvement, and cost reduction. Data-driven methods based on deep learning have become an effective solution for tool wear monitoring, but in actual cutting process, multiple sensors are often used for high-frequency sampling to obtain multi-level information, so that tool wear data has multi-channel spatial structure and time series characteristics, respectively. Therefore, aiming at the distribution of tool wear data, a one-dimensional residual structure is designed and a novel hybrid model integrating bi-directional long short-term memory (BLSTM) network is proposed, which is suitable for tool wear monitoring, named Re-BLSTM. Considering the spatial distribution of tool wear signal, a residual structure is constructed based on one-dimensional convolution neural network (1-D CNN) to realize abstract feature extraction and signal denoising, while gradient vanishing and degradation problem can be solved as the deeper of network. Additionally, batch normalization (BN) layer and dropout operation are introduced to form a pre-activation structure, which can alleviate the over fitting problem caused with the increase of model parameters; Subsequently, in view of the temporal correlation of feature, long-term dependence of concatenated feature is explored by integrating BLSTM and the learning range is also expanded. To verify the performance of the proposed model, experiments are carried out compared with several state-of-the-art method. The results show the effectiveness and generalization of the proposed method.
更多查看译文
关键词
Tool condition monitoring,Deep learning,Residual learning,Long short-term memory network,Tool wear monitoring,RUL prediction
AI 理解论文
溯源树
样例
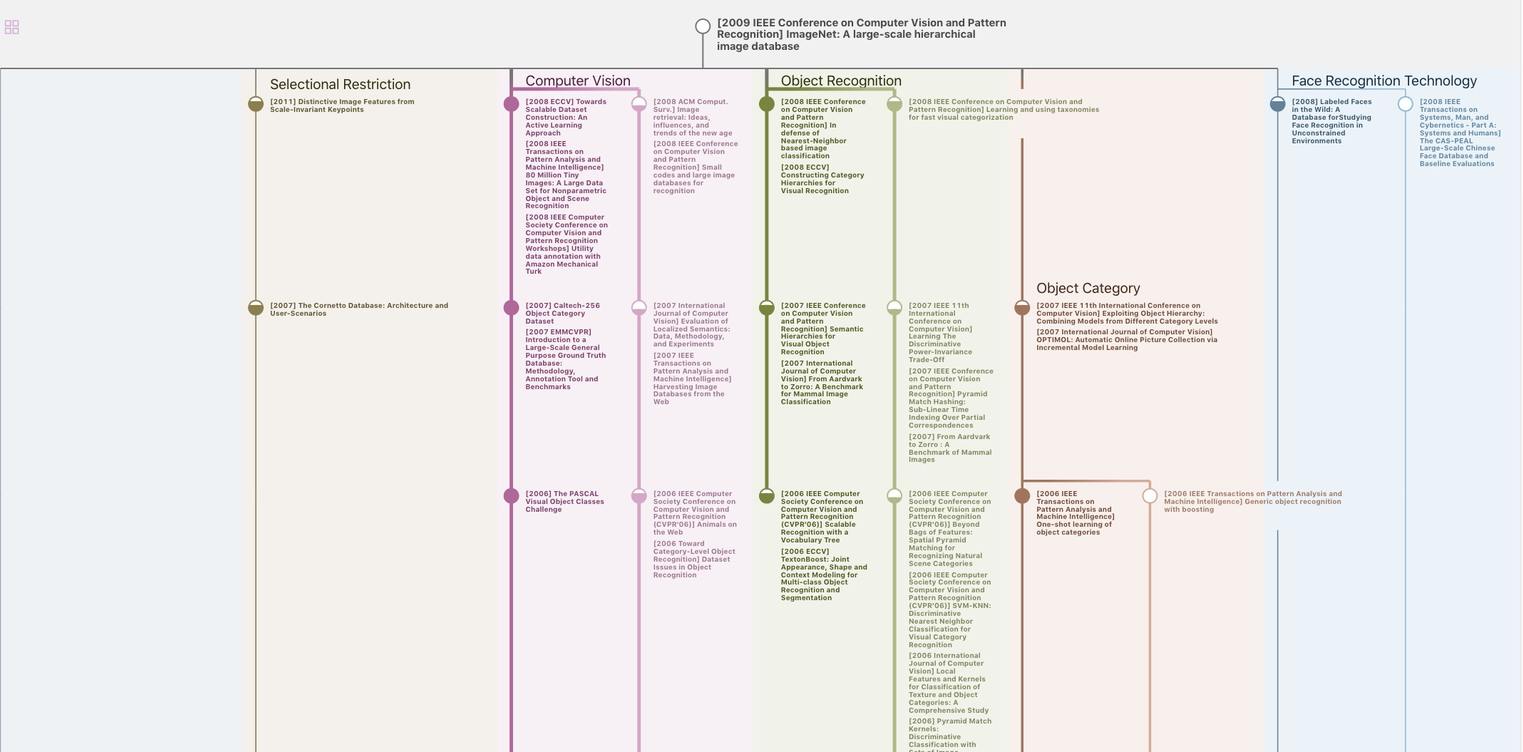
生成溯源树,研究论文发展脉络
Chat Paper
正在生成论文摘要