Brain Tumor Segmentation Based on 2D U-Net Using MRI Multi-modalities Brain Images
SMART TECHNOLOGIES, SYSTEMS AND APPLICATIONS, SMARTTECH-IC 2021(2022)
摘要
Glioblastoma is the most common malignant primary brain tumor, making up approximately 80% of all malignant primary brain tumors and 48.6% of all malignant tumors. Regardless of the diagnosis, the morbidity and mortality associated with a brain tumor are significant. They have a 5-year relative survival rate of around 7.2% and an average survival time of roughly 8 months. It's a difficult but vital endeavor to detect the existence of brain tumors utilizing magnetic resonance images in a quick, precise, and repeatable manner. The delineation of different tumor locations, such as peritumoral edema, enhancing tumor, and tumor core, is a critical aspect in the analysis of gliomas. Various approaches in the literature now use the tumor segmentation methodology to improve diagnosis and treatment plans. The suggested methodology entails using neural networks, specifically U-Net and Attention U-Net, to automate the segmentation of brain tumors. In this study, the BraTS 2020 dataset is used to evaluate the segmentation performance of the proposed approach. The accuracy of 0.9950 was obtained for both models, and a sensitivity of 0.9931 and 0.9891 for the U-Net and Attention U-Net models. A peritumoral edema, enhancing tumor, and tumor core dice similarity coefficient of 0.8453, 0.6950, and 0.7429 respectively has been achieved, for the U-Net model. For the Attention U-Net model, a dice score of 0.8829, 0.7233, and 0.8090 was obtained. Results show that both approaches have considerable potential and can be employed in clinical practice in the segmentation of various sub-regions of brain tumors.
更多查看译文
关键词
Glioma, Brain tumor segmentation, U-net, Multimodal MRI, BraTS dataset
AI 理解论文
溯源树
样例
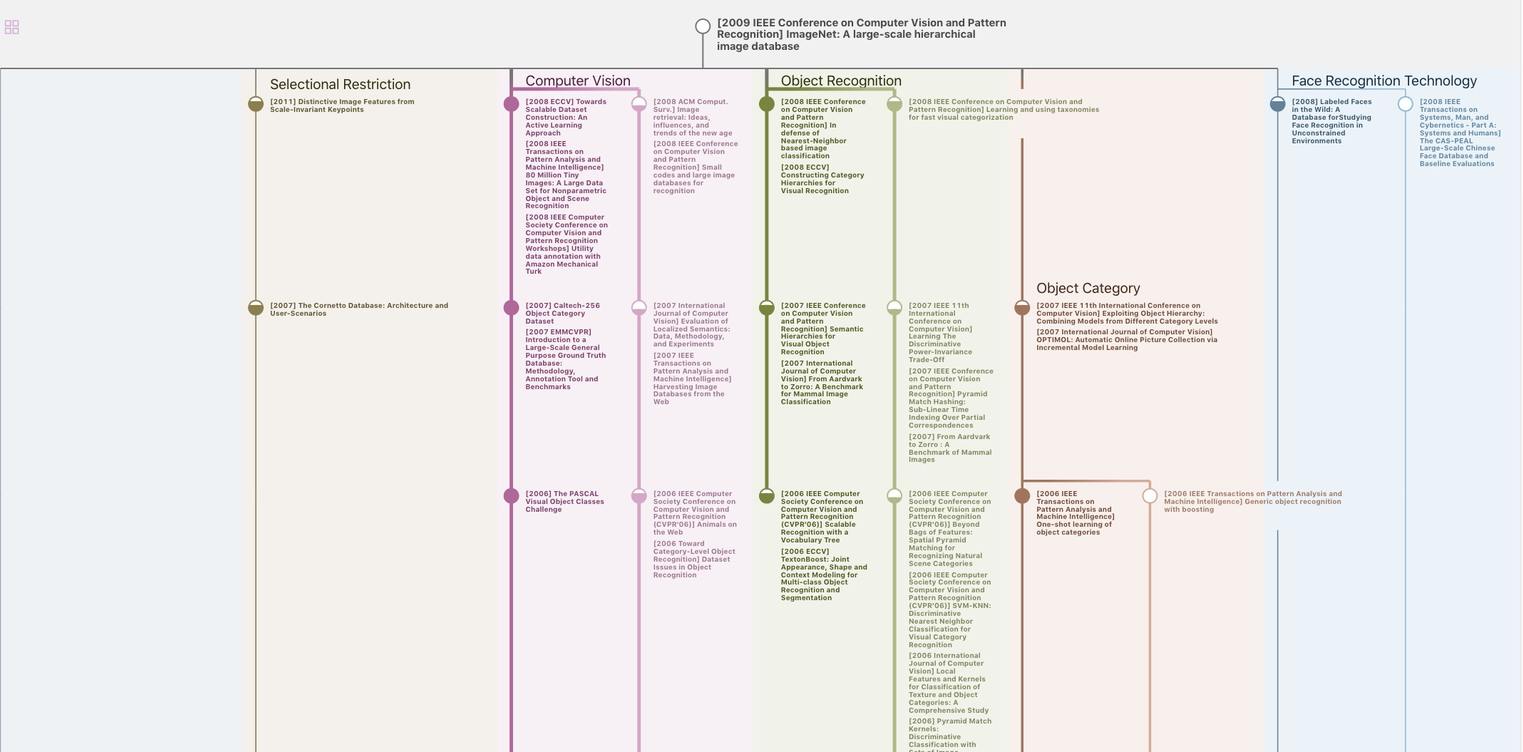
生成溯源树,研究论文发展脉络
Chat Paper
正在生成论文摘要