Suicide Ideation Detection on Social Media During COVID-19 via Adversarial and Multi-task Learning
WEB AND BIG DATA, APWEB-WAIM 2021, PT I(2021)
摘要
Suicide ideation detection on social media is a challenging problem due to its implicitness. In this paper, we present an approach to detect suicide ideation on social media based on a BERT-LSTM model with Adversarial and Multi-task learning (BLAM). More specifically, BLAM combines BERT model with Bi-LSTM model to extract deeper and richer features. Furthermore, emotion classification is utilized as an auxiliary task to perform multi-task learning, which enriches the extracted features with emotion information that enhances the identification of suicide. In addition, BLAM generates adversarial noise by adversarial learning improving the generalization ability of the model. Extensive experiments conducted on our collected Suicide Ideation Detection (SID) dataset demonstrate the competitive superiority of BLAM compared with the state-of-the-art methods.
更多查看译文
关键词
Suicide ideation detection, Adversarial learning, Multi-task learning
AI 理解论文
溯源树
样例
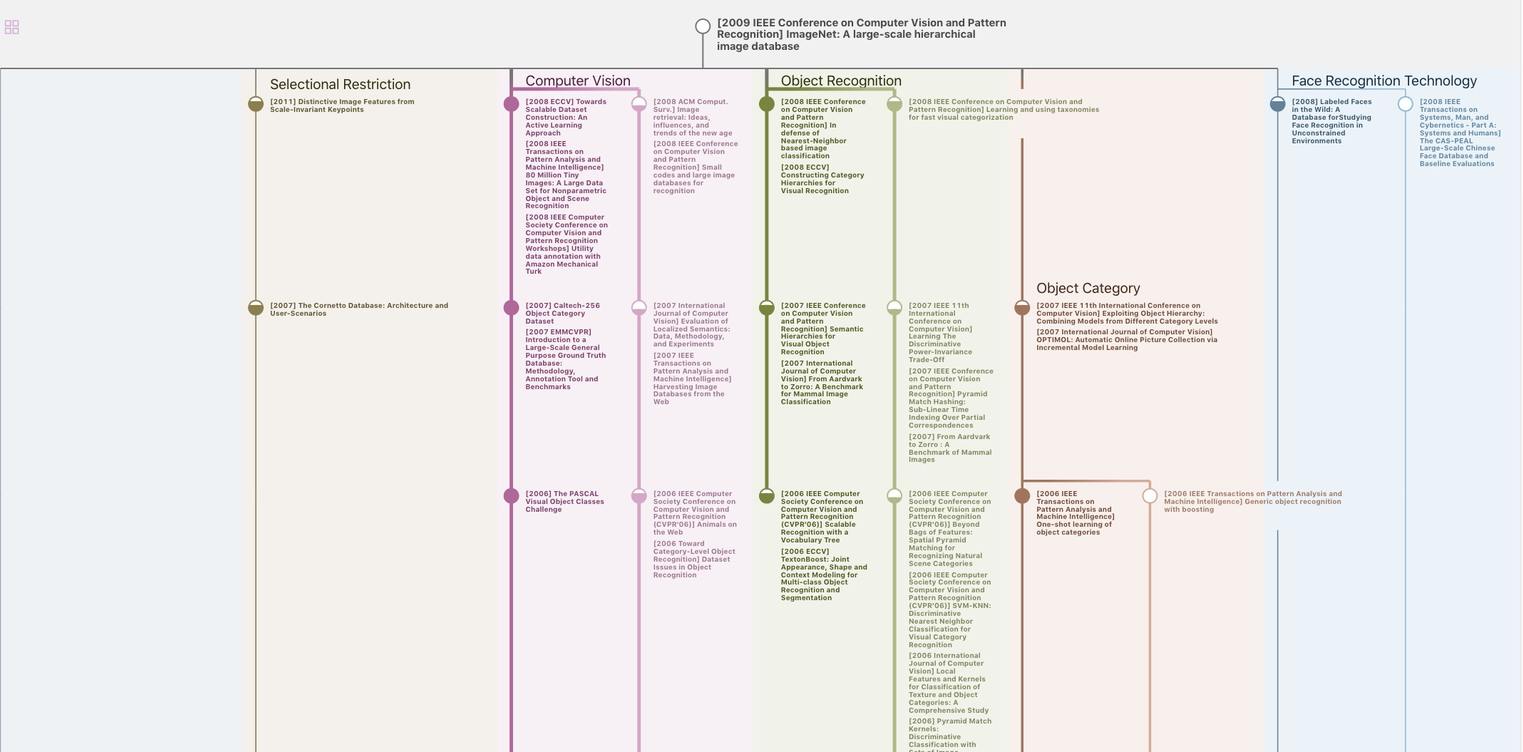
生成溯源树,研究论文发展脉络
Chat Paper
正在生成论文摘要