Deep learning-influenced joint vehicle-to-infrastructure and vehicle-to-vehicle communication approach for internet of vehicles
EXPERT SYSTEMS(2022)
摘要
The internet of vehicle (IoV) orchestration is an emerging technology in heterogeneous vehicles to contrivance diverse intelligent transportation applications. The roadside unit (RSU) plays a vital role during service provisioning. Vehicle-to-vehicle and vehicle-to-infrastructure communications have consistently accomplished the services in a vehicular network. However, persisting the increased vehicles' quality of experience and network vendors' utilities and which RSUs have to select for effective, reliable service are critical open research challenges to consolidate RSU services to enhance network service utility rate. In this article, we design a deep learning-inspired RSU Service Consolidation Approach based on two-models to enhance the service reliability by formulating the RSU coverage issue with the RSU Migration model and content delivery issue with Linear Programming-based Multicast model. Adaptive Packet-Error measurement system to optimize service reliability rate at the edge of cooperative vehicular network based on content correlation. The performance and efficiency are examined based on MATLAB. The simulation outcome shows RSC approach has low execution cost by 39%, service reliability rate by 71% than the state-of-art approaches.
更多查看译文
关键词
deep learning, edge computing, performance metrics, road side unit consolidation, service-centric architecture design, vehicle-to-infrastructure, vehicle-to-vehicle
AI 理解论文
溯源树
样例
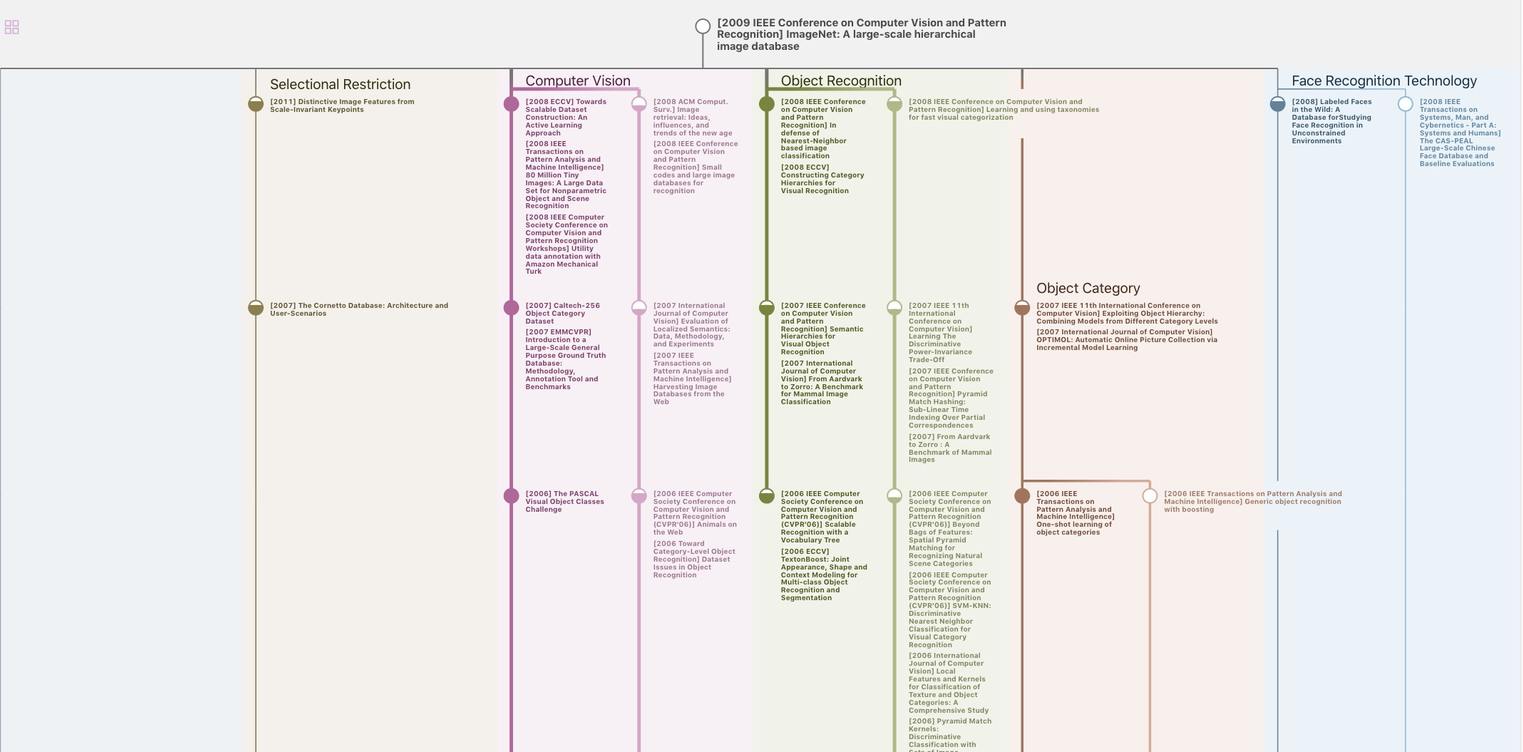
生成溯源树,研究论文发展脉络
Chat Paper
正在生成论文摘要