Lagrangian Integro-Difference Equation Model for Precipitation Nowcasting
JOURNAL OF ATMOSPHERIC AND OCEANIC TECHNOLOGY(2021)
摘要
Delivering reliable nowcasts (short-range forecasts) of severe rainfall and the resulting flash floods is important in densely populated urban areas. The conventional method is advection-based extrapolation of radar echoes. However, during rapidly evolving convective rainfall this so-called Lagrangian persistence (LP) approach is limited to deterministic and very short-range nowcasts. To address these limitations in the 1-h time range, a novel extension of LP, called Lagrangian Integro-Difference equation model with Autoregression (LINDA), is proposed. The model consists of five components: 1) identification of rain cells, 2) advection, 3) autoregressive process describing growth and decay of the cells, 4) convolution describing loss of predictability at small scales, and 5) stochastic perturbations to simulate forecast uncertainty. Advection is separated from the other components that are applied in the Lagrangian coordinates. The reliability of LINDA is evaluated using the NEXRAD WSR-88D radar that covers the Dallas-Fort Worth metropolitan area, as well as the NEXRAD mosaic covering the continental United States. This is done with two different configurations: LINDA-D for deterministic and LINDA-P for probabilistic nowcasts. The validation dataset consists of 11 rainfall events during 2018-20. For predicting moderate to heavy rainfall (5-20 mm h(-1)), LINDA outperforms the previously proposed LP-based approaches. The most significant improvement is seen for the ETS and POD statistics with the 5 mm h(-1) threshold. For 30-min nowcasts, they show 15% and 16% increases, respectively, to the second-best method and 48% and 34% increases compared to LP. For the 5 mm h(-1) threshold, the increase in the relative operating characteristic (ROC) skill score of 30-min nowcasts from the second-best method is 10%.
更多查看译文
关键词
Rainfall, Radars/Radar observations, Nowcasting, Probabilistic Quantitative Precipitation Forecasting(PQPF), Stochastic models, Urban meteorology
AI 理解论文
溯源树
样例
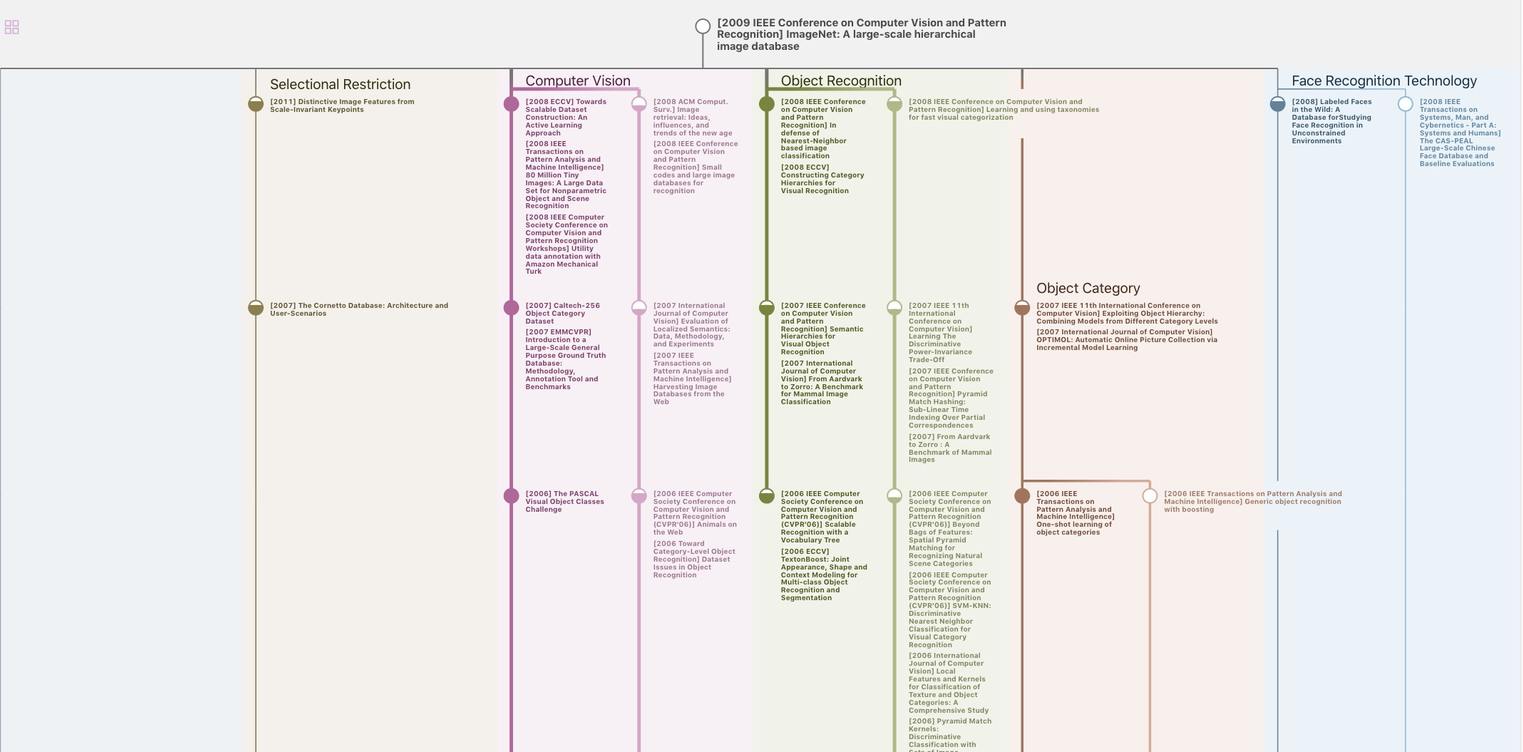
生成溯源树,研究论文发展脉络
Chat Paper
正在生成论文摘要