An extended gained and lost dominance score method based risk prioritization for Fine-Kinney model with interval type-2 fuzzy information
HUMAN AND ECOLOGICAL RISK ASSESSMENT(2022)
摘要
The Fine-Kinney model is one of the most extensively adopted classical occupational risk analysis techniques which has been regarded as a helpful means for addressing the risk identification and evaluation problems in various fields. However, current extended Fine-Kinney models have limited capacity to cope with occupational risk evaluation and prioritization problems considering interactive relationships among multi-risk parameters under inter-uncertain and intra-uncertain environments. To overcome the limitations, this paper proposes a hybrid risk prioritization approach for the Fine-Kinney model by integrating GLDS (gained and lost dominance score) method, interval type-2 fuzzy sets (IT2FSs), and Maclaurin symmetric mean (MSM) operator. First, the trapezoidal interval type-2 fuzzy numbers (TrIT2FNs) are introduced to depict the inter-uncertain and intra-uncertain risk evaluation information. Then, an ordered weighted averaging (OWA) operator is constructed to aggregate the complex and uncertain risk evaluation information from heterogeneous decision-makers in which the similarity-measure-based weighting method is incorporated. Next, the GLDS method combined with the weighted MSM operator is developed to address the risk prioritization problem in the Fine-Kinney model by considering the inter-dependencies among multi-risk parameters. Finally, a numerical example of occupational risk evaluation for potential hazards in construction excavation is presented within IT2FSs to demonstrate the application and availability of the extended Fine-Kinney model. After that, the comparison and sensitivity analyses are conducted to further illustrate the superiority and rationality of the proposed approach.
更多查看译文
关键词
Fine-Kinney, risk prioritization, gained and lost dominance score method, interval type-2 fuzzy set
AI 理解论文
溯源树
样例
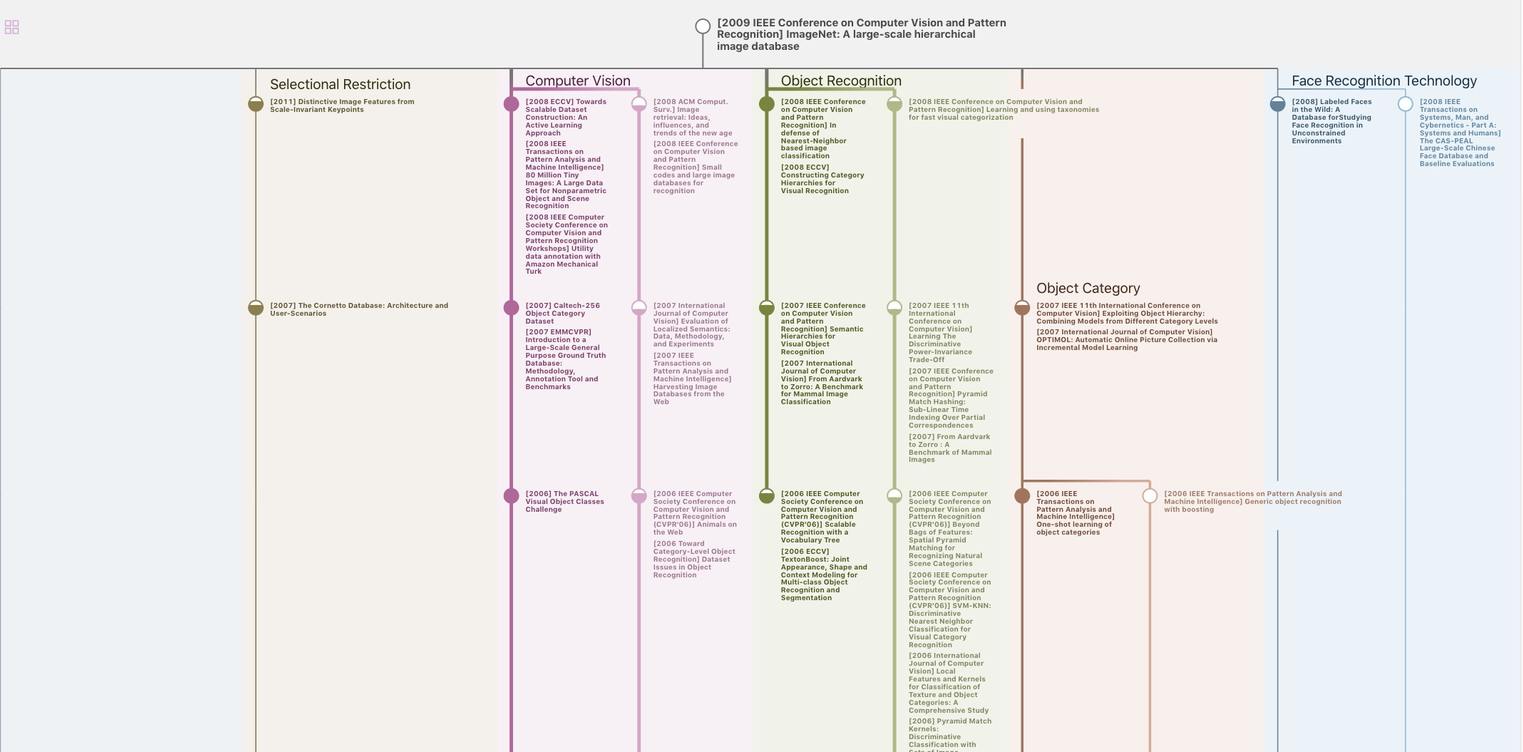
生成溯源树,研究论文发展脉络
Chat Paper
正在生成论文摘要