Modified Error Bounds for Matrix Completion and Application to RL
IEEE CONTROL SYSTEMS LETTERS(2022)
摘要
In matrix completion under noisy measurements, most available results assume that there is an a priori bound on the Frobenius norm of the noise, and derive bounds on the Frobenius norm of the residual error. In this letter, we obtain "component-wise" bounds on the residual error, based on a similar bound on the noise. This among the first such results. As in our earlier paper, choose the locations of the samples to correspond to the edge set of a Ramanujan bigraph. One recent application is to deriving nearly optimal action-value functions in Reinforcement Learning (RL), which is illustrated through examples. The results presented here are only sufficient conditions. Then we illustrate through numerical simulations that there is considerable room for improvement in the sufficient conditions derived here. This is a problem for future research.
更多查看译文
关键词
Noise measurement,Reinforcement learning,Sparse matrices,Minimization,Matrix decomposition,Upper bound,Standards,Statistical learning,machine learning,iterative learning control
AI 理解论文
溯源树
样例
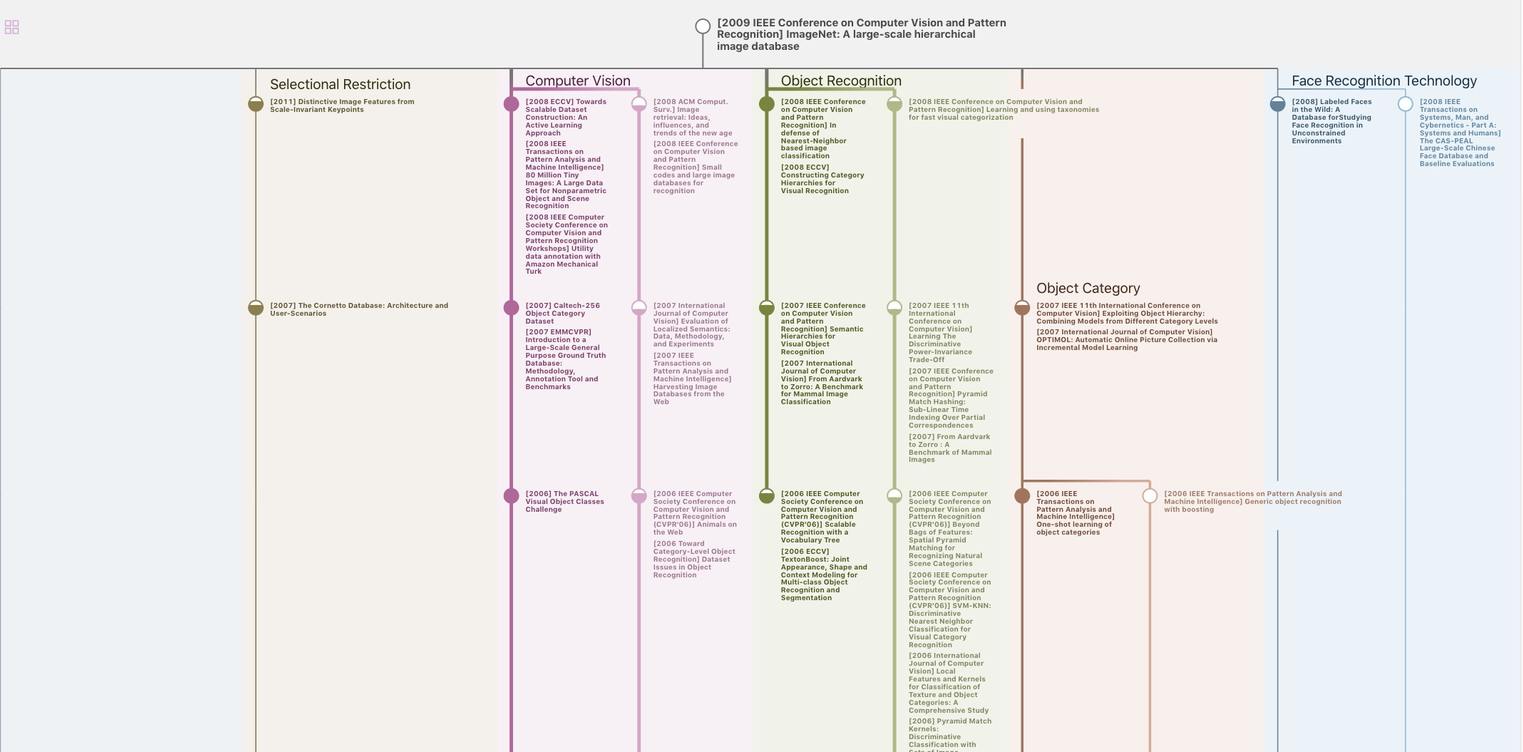
生成溯源树,研究论文发展脉络
Chat Paper
正在生成论文摘要