Enhance text-to-SQL model performance with information sharing and reweight loss
Multimedia Tools and Applications(2022)
摘要
The goal of Text-to-SQL task is to map natural language queries into equivalent structured query languages(NL2SQL). On the WikiSQL dataset, the method used by the state-of-the-art models is to decouple the NL2SQL task into subtasks and then build a dedicated decoder for each subtask. There are some problems in this method, such as the model is too complicated, and the ability to learn the dependency between different subtasks is limited. To solve these problems, this paper innovatively introduces the sharing mechanism of multi-task learning into the NL2SQL task and realizes sharing by letting different subtasks share the same decoder. Firstly, sharing decoders for different subtasks can effectively reduce the complexity of the model, and at the same time, allows different subtasks to share knowledge during the training process so that the model can better learn the dependencies between different subtasks. This paper also designed a re-weighted loss to balance the complexity of the SELECT clause and the WHERE clause. We have evaluated the method in this article on the WikiSQL dataset. The experimental results show that the accuracy of the proposed model is better than state-of-the-art on the WikiSQL without execution guided decoding.
更多查看译文
关键词
Text-to-SQL,Multi-task learning,Re-weighted loss
AI 理解论文
溯源树
样例
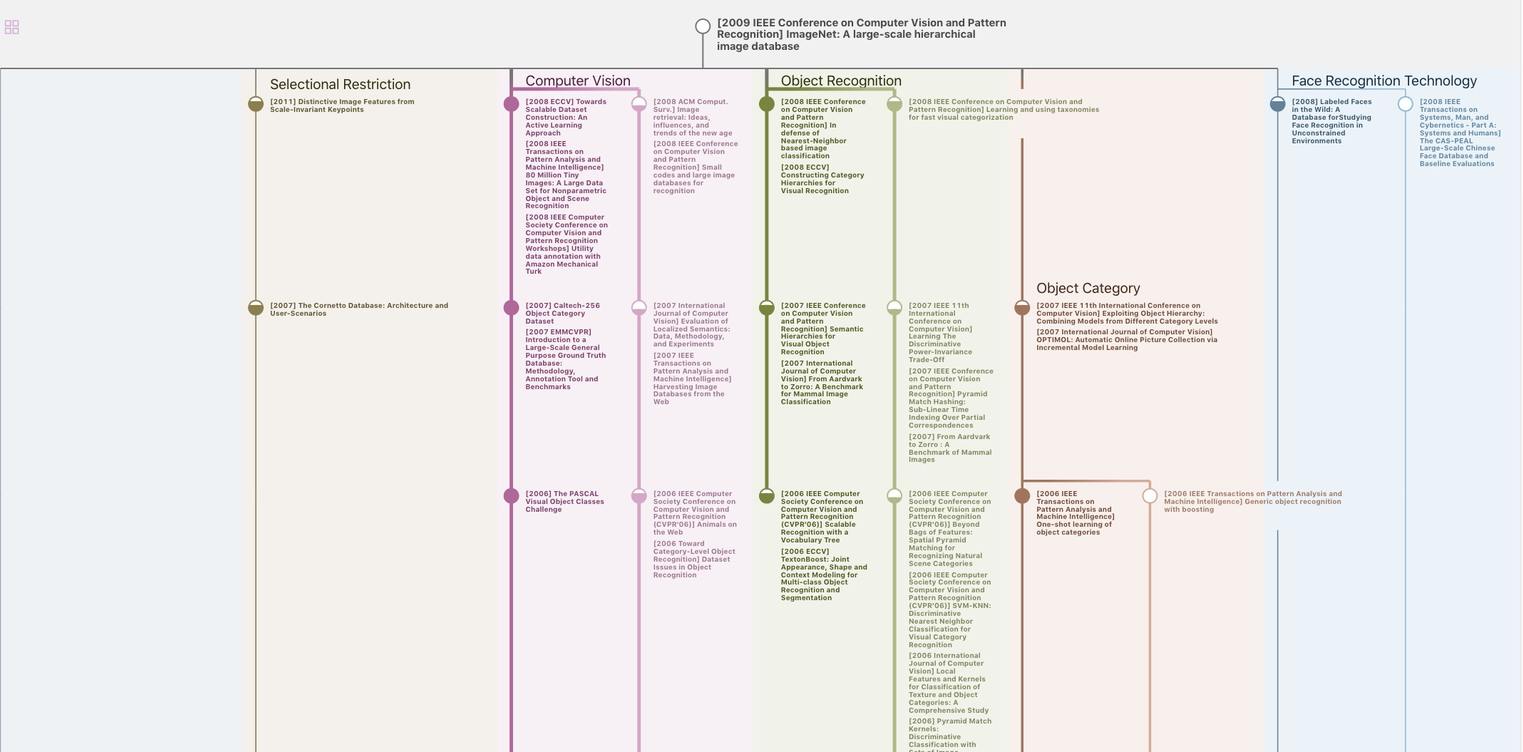
生成溯源树,研究论文发展脉络
Chat Paper
正在生成论文摘要