An Engineering Domain Knowledge-Based Framework for Modelling Highly Incomplete Industrial Data
INTERNATIONAL JOURNAL OF DATA WAREHOUSING AND MINING(2021)
摘要
The missing values in industrial data restrict the applications. Although this incomplete data contains enough information for engineers to support subsequent development, there are still too many missing values for algorithms to establish precise models. This is because the engineering domain knowledge is not considered, and valuable information is not fully captured. Therefore, this article proposes an engineering domain knowledge-based framework for modelling incomplete industrial data. The raw datasets are partitioned and processed at different scales. Firstly, the hierarchical features are combined to decrease the missing ratio. In order to fill the missing values in special data, which is identified for classifying the samples, samples with only part of the features presented are fully utilized instead of being removed to establish local imputation model. Then samples are divided into different groups to transfer the information. A series of industrial data is analyzed for verifying the feasibility of the proposed method.
更多查看译文
关键词
Data Mining, Data-Driven Engineering, Feature Combination, Feature Extraction, Industrial Data, Local Imputation Model, Missing Data Imputation, Neural Network Applications, Occupant Protection
AI 理解论文
溯源树
样例
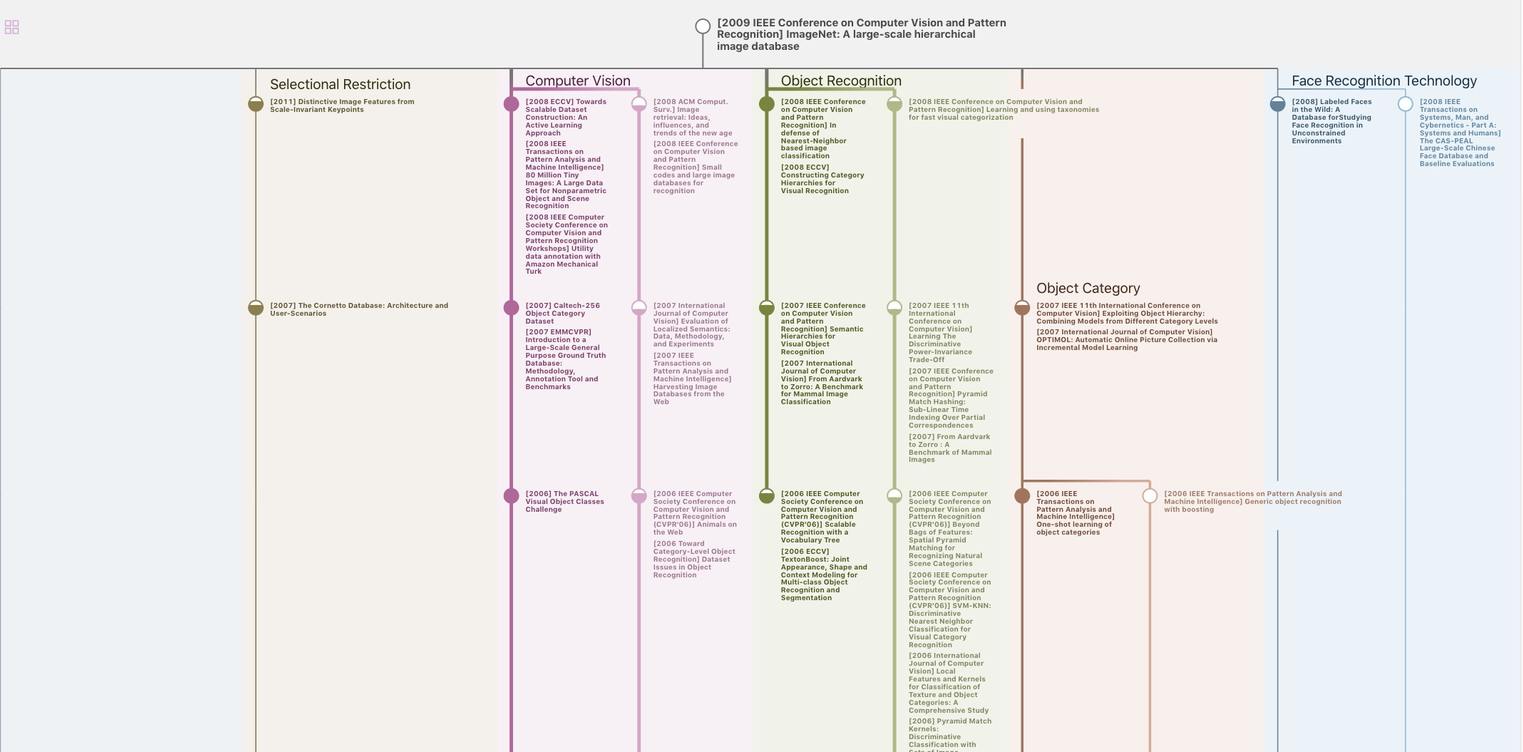
生成溯源树,研究论文发展脉络
Chat Paper
正在生成论文摘要