Research on maintenance spare parts requirement prediction based on LSTM recurrent neural network
OPEN PHYSICS(2021)
摘要
The aim of this study was to improve the low accuracy of equipment spare parts requirement predicting, which affects the quality and efficiency of maintenance support, based on the summary and analysis of the existing spare parts requirement predicting research. This article introduces the current latest popular long short-term memory (LSTM) algorithm which has the best effect on time series data processing to equipment spare parts require-ment predicting, according to the time series characteristics of spare parts consumption data. A method for predicting the requirement for maintenance spare parts based on the LSTM recurrent neural network is proposed, and the net-work structure is designed in detail, the realization of network training and network prediction is given. The advantages of particle swarm algorithm are introduced to optimize the network parameters, and actual data of three types of equipment spare parts consumption are used for experiments. The performance comparison of predictive models such as BP neural network, generalized regression neural network, wavelet neural network, and squeeze-and-excitation network prove that the new method is effective and provides an effective method for scientifically predicting the requirement for maintenance spare parts and improving the quality of equipment maintenance.
更多查看译文
关键词
LSTM, spare parts prediction, neural network, particle swarm
AI 理解论文
溯源树
样例
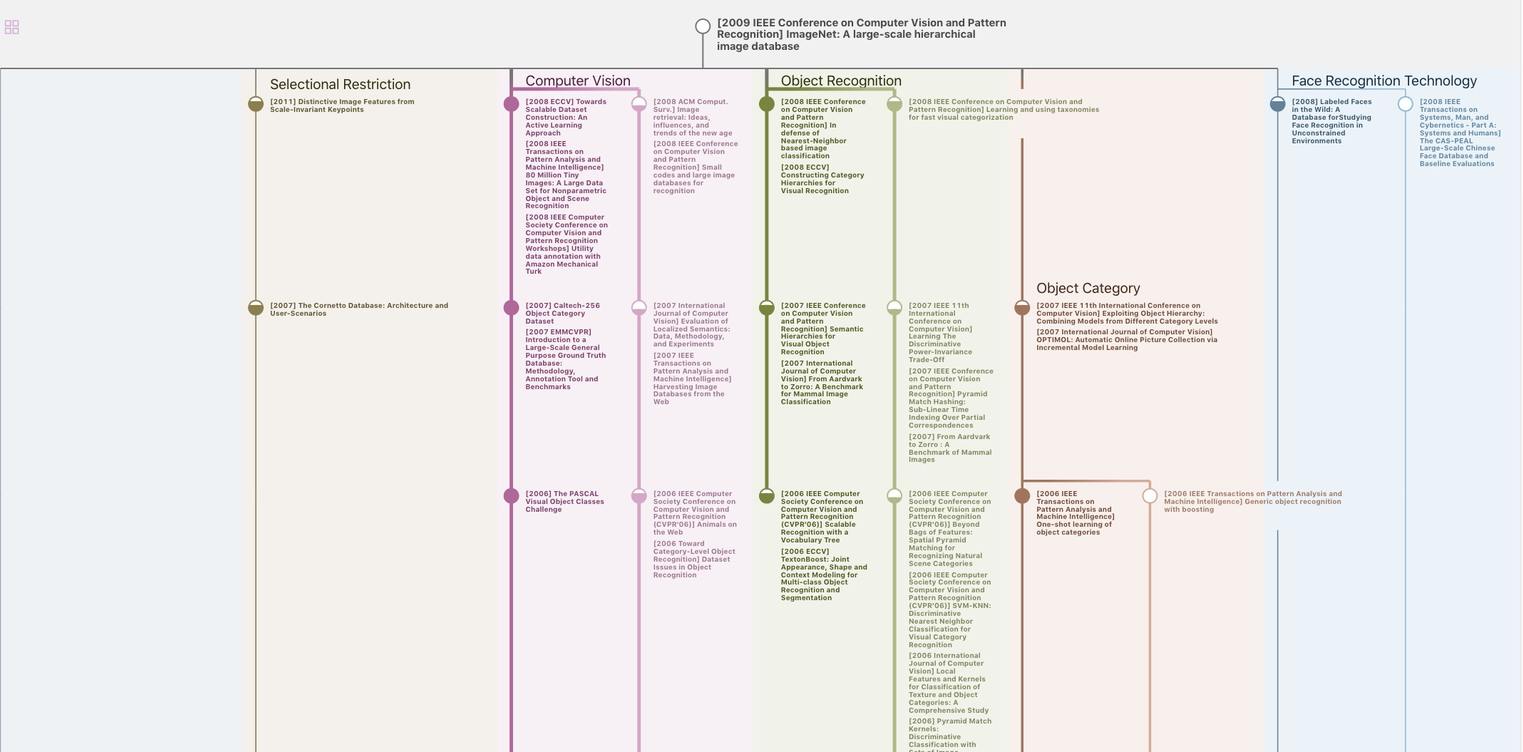
生成溯源树,研究论文发展脉络
Chat Paper
正在生成论文摘要