Aberration analysis and compensate method of fully connected neural network in deep ultraviolet lithography
OPTICAL ENGINEERING(2021)
摘要
Thermal aberration of a projection lens is caused by lens heating and leads to degradation of image quality. Two tasks have been undertaken in this article: first, analysis of the impact of single aberration on imaging quality, and second, use of machine learning to calculate the combination of Zernike aberration coefficients to reduce the influence of aberration on the process window. The impact of aberration on deep ultraviolet (DUV) lithography imaging has been analyzed separately for 16 Zernike terms. The influence of single Zernike coefficient on depth of focus, mask error enhancement factor, and image log slope is comprehensively analyzed by source mask optimization. However, more than one Zernike term is present in the aberration of a real DUV optics. A fully connected neural network (FCNN) model based on machine learning is widely used in the screening of multivariate experiments and can solve the problem of the large number of input variables and the random values of the input data. Its network structure is simple to debug, with fast screening speed and high precision. To make reasonable budget for aberration, an FCNN model is used to find some combinations of Zernike coefficients for different lithographic performance indicators. This implies that the FCNN model has the ability to select a better Zernike combination while each individual Zernike term value falls in the range of -30 to 30 m lambda, which plays a guiding role in DUV optics design. (C) 2021 Society of Photo-Optical Instrumentation Engineers (SPIE)
更多查看译文
关键词
lithography, aberration, machine learning, fully connected neural network, source mask optimization
AI 理解论文
溯源树
样例
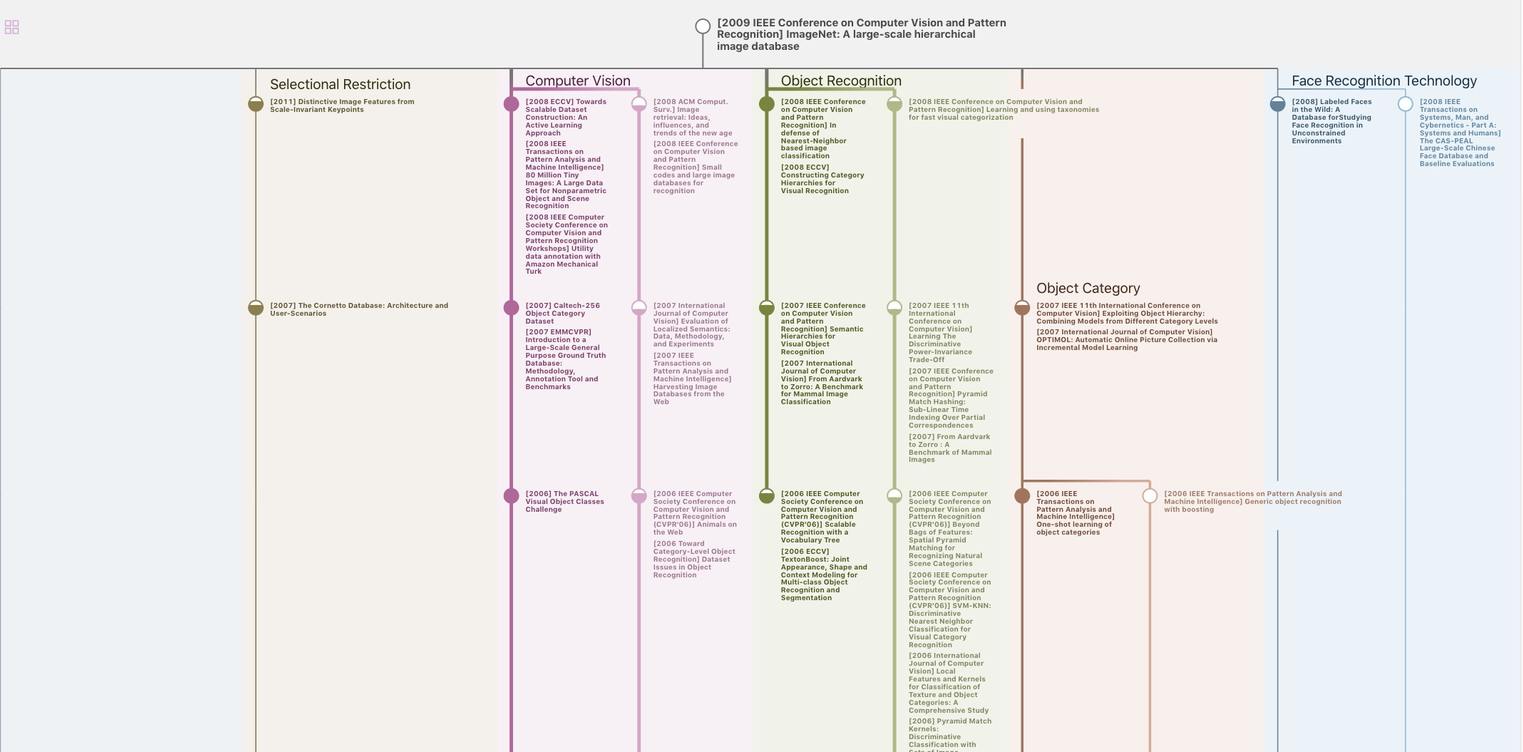
生成溯源树,研究论文发展脉络
Chat Paper
正在生成论文摘要