Mutual Information Measure for Image Segmentation Using Few Labels
MACHINE LEARNING AND KNOWLEDGE DISCOVERY IN DATABASES: APPLIED DATA SCIENCE TRACK, ECML PKDD 2020, PT IV(2021)
摘要
Recently several models have been developed to reduce the annotation effort which is required to perform semantic segmentation. Instead of learning from pixel-level annotations, these models learn from cheaper annotations, e.g. image-level labels, scribbles or bounding boxes. However, most of these models cannot easily be adapted to new annotations e.g. new classes since it requires retraining the model. In this paper, we propose a similarity measure between pixels based on a mutual information objective to determine whether these pixels belong to the same class. The mutual information objective is learned in a fully unsupervised manner while the annotations (e.g. points or scribbles) are only used during test time. For a given image, the unlabeled pixels are classified by computing their nearest-neighbors in terms of mutual information from the set of labeled pixels. Experimental results are reported on the Potsdam dataset and Sentinel-2 data is used to provide a real world use case where a large amount of unlabeled satellite images is available but only a few pixels can be labeled. On the Potsdam dataset, our model achieves 70.22% mIoU and 87.17% accuracy outperforming the state-of-the-art weakly-supervised methods.
更多查看译文
关键词
Mutual information maximization, Weakly supervised learning, Similarity measure, Image segmentation, Satellite datasets
AI 理解论文
溯源树
样例
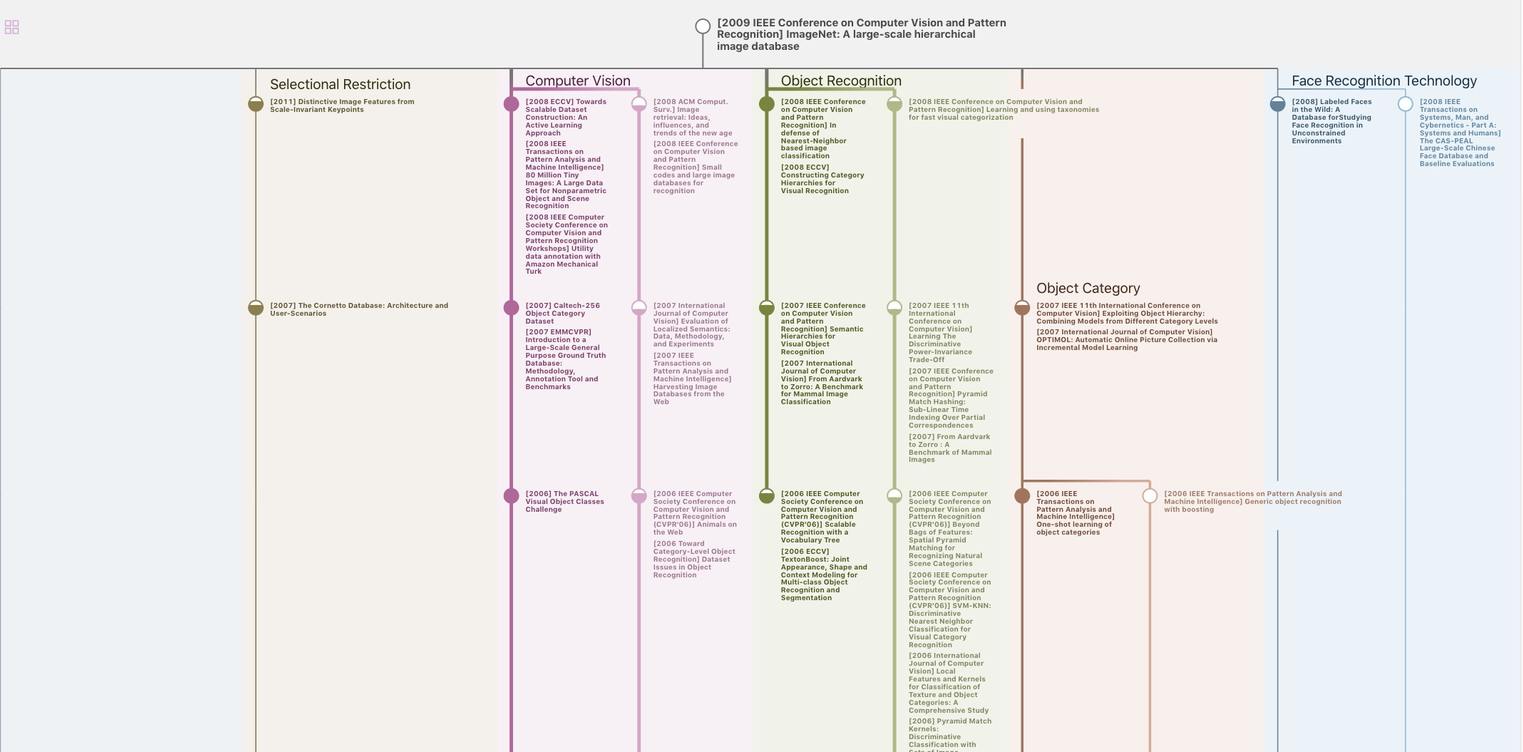
生成溯源树,研究论文发展脉络
Chat Paper
正在生成论文摘要