A Comparative Study of Bot Detection Techniques With an Application in Twitter Covid-19 Discourse
SOCIAL SCIENCE COMPUTER REVIEW(2023)
摘要
Bot Detection is crucial in a world where Online Social Networks (OSNs) play a pivotal role in our lives as public communication channels. This task becomes highly relevant in crises like the Covid-19 pandemic when there is a growing risk of proliferation of automated accounts designed to produce misinformation content. To address this issue, we first introduce a comparison between supervised Bot Detection models using Data Selection. The techniques used to develop the bot detection models use features such as the tweets' metadata or accounts' Digital Fingerprint. The techniques implemented in this work proved effective in detecting bots with different behaviors. Social Fingerprint-based methods have been found to be effective with bots that behave in a coordinated manner. Furthermore, all these approaches have produced excellent results compared to the Botometer v3. Second, we present and discuss a case study related to the Covid-19 pandemic that analyses the differences in the discourse between bots and humans on Twitter, a platform used worldwide to express opinions and engage in dialogue in a public arena. While bots and humans generally express themselves alike, the tweets' content and sentiment analysis reveal some dissimilitudes, especially in tweets concerning President Trump. When the discourse switches to pandemic management by Trump, sentiment-related values display a drastic difference, showing that tweets generated by bots have a predominantly negative attitude. However, according to our findings, while automated accounts are numerous and active in discussing controversial issues, they usually do not seem to increase exposure to negative and inflammatory content for human users.
更多查看译文
关键词
bot detection, Covid-19, Twitter, discourse analysis, data selection, sentiment
AI 理解论文
溯源树
样例
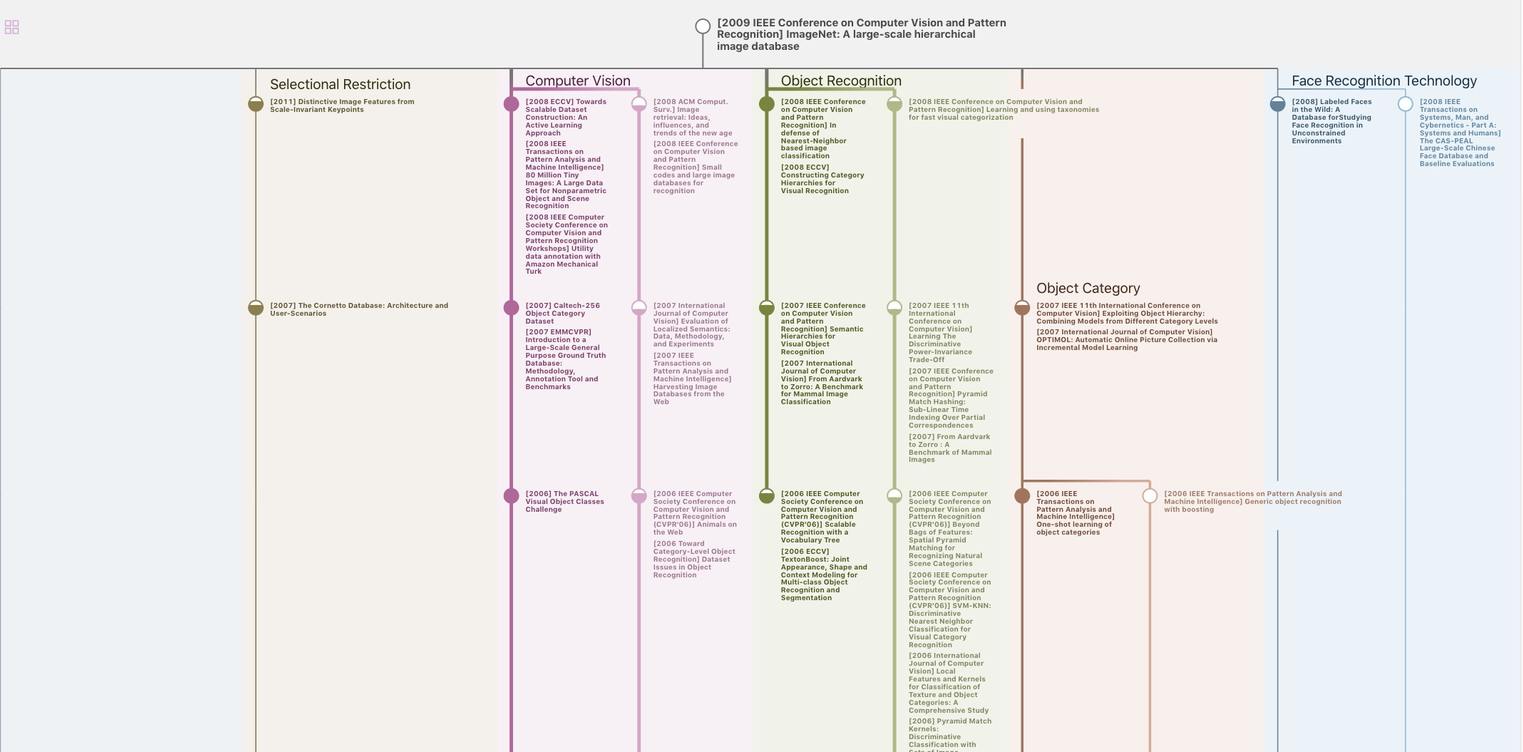
生成溯源树,研究论文发展脉络
Chat Paper
正在生成论文摘要