A population-based SHM methodology for heterogeneous structures: Transferring damage localisation knowledge between different aircraft wings
Mechanical Systems and Signal Processing(2022)
摘要
Population-based structural health monitoring (PBSHM) offers a new viewpoint for structural health monitoring (SHM), allowing diagnostic information to be shared across populations of structures. By extending the set of available damage observations, a population-based approach can diagnose damage previously unseen on a structure of interest by leveraging damage information from other structures in the population. These technologies therefore provide significant benefits for making SHM practicable in a variety of industrial settings. It is proposed that PBSHM methodologies must be comprised of tools to assess similarity coupled with algorithms that perform knowledge transfer. The similarity tools are important for identifying whether an SHM task should be attempted for a given population by assessing both the structural similarities between members of a population and similarities in their data spaces. An abstract representation of structures in a graphical domain is presented as an objective way of assessing structural similarity, with distance metrics utilised for assessing data-space similarities. Knowledge transfer is performed using a branch of transfer learning called domain adaptation. By determining if members of a population are similar in a structural and data-space sense, the risk of negative transfer can be reduced; whereby domain adaptation reduces classification performance. This paper demonstrates a PBSHM methodology for transferring knowledge within a heterogeneous population (a group of non-identical structures). Specifically, the PBSHM methodology is shown to transfer localisation labels from a Gnat aircraft wing to an unlabelled Piper Tomahawk aircraft wing dataset, resulting in 100% classification accuracy.
更多查看译文
关键词
Population-based structural health monitoring,Irreducible elements,Attributed graphs,Transfer learning,Domain adaptation
AI 理解论文
溯源树
样例
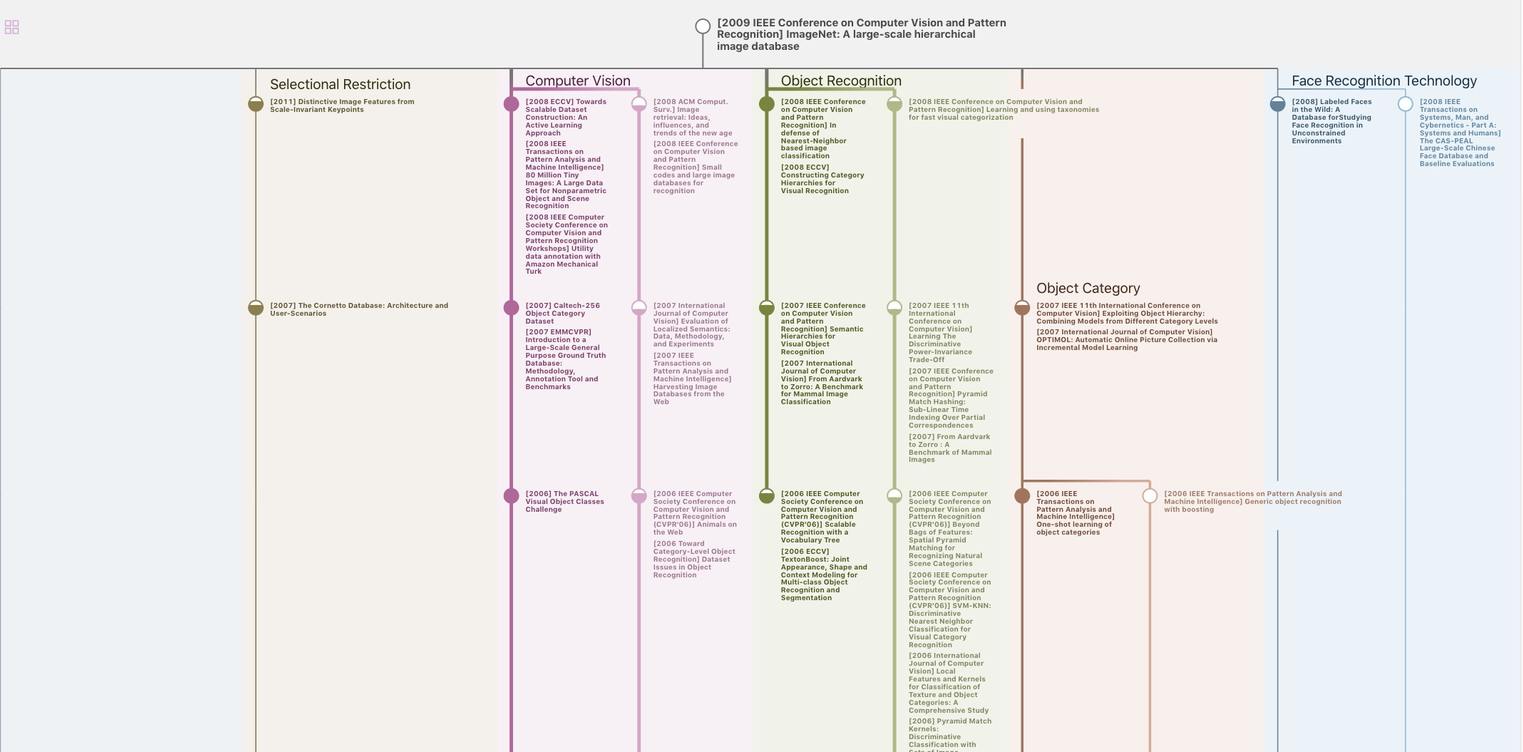
生成溯源树,研究论文发展脉络
Chat Paper
正在生成论文摘要