A Deep 1-D CNN and Bidirectional LSTM Ensemble Model With Arbitration Mechanism for LDDoS Attack Detection
IEEE TRANSACTIONS ON EMERGING TOPICS IN COMPUTATIONAL INTELLIGENCE(2022)
摘要
Low-rate Distributed Denial of Service (LDDoS) attacks are complex and common security issues, which disrupt the essential services of the varied and emerging networks. However, the traditional detection models are normally in a dilemma condition since LDDoS attacks have the characteristics of concealment and persistence in space and time dimensions. In this paper, we propose a novel, practical and fast ensemble model (FastCBLA-EM) to detect the LDDoS attacks. In the FastCBLA-EM, a dilated padding-based dual sliding method is worked out for producing fixed-length chronological order samples from flows per well-tuned packet step. Next, a competitive network composed of 1-D Convolutional Neural Network (1-D CNN) and a bidirectional Long Short Term Memory (bi-LSTM) network is employed to learn both spatial and temporal representations of samples, parallelly. Finally, an arbitration mechanism based on the enhanced attention method is used to assemble and weigh these representations for detecting LDDoS attacks. We compare the performances of the FastCBLA-EM with other models by obtaining a group of well-tuned hyperparameters in the revised ISCX-2016-SlowDos flow-based dataset. Experimental results demonstrate that the detection accuracy of the FastCBLA-EM can be up to 99.7% and the time complexity is $O(n)$.
更多查看译文
关键词
Feature extraction, Convolutional neural networks, Data models, Computational modeling, Support vector machines, Time-domain analysis, Time complexity, 1-D CNN, arbitration mechanism, bidirectional LSTM, ensemble model, low-rate distributed denial of service
AI 理解论文
溯源树
样例
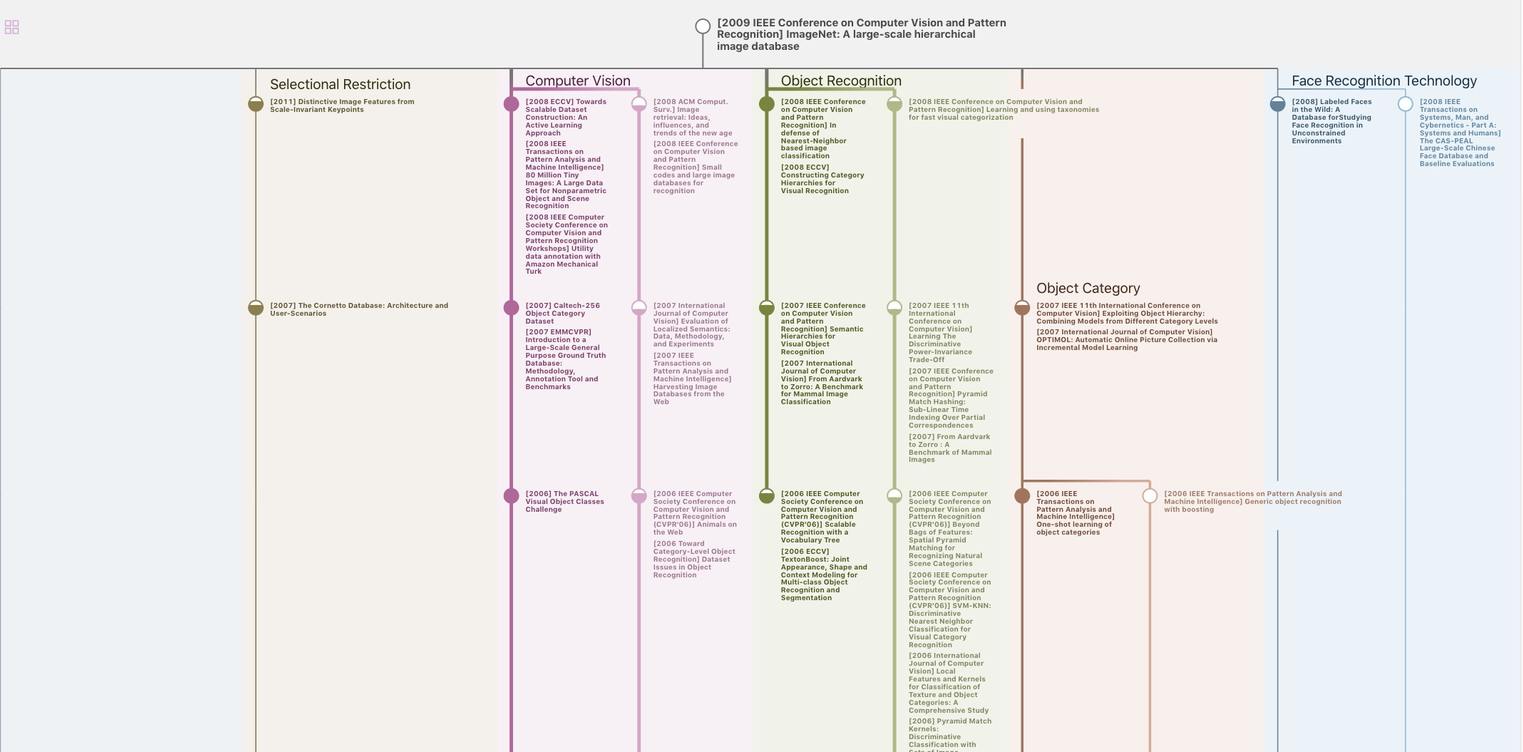
生成溯源树,研究论文发展脉络
Chat Paper
正在生成论文摘要