Attention Mechanism-Based Convolutional Long Short-Term Memory Neural Networks to Electrocardiogram-Based Blood Pressure Estimation
APPLIED SCIENCES-BASEL(2021)
摘要
Monitoring people's blood pressure can effectively prevent blood pressure-related diseases. Therefore, providing a convenient and comfortable approach can effectively help patients in monitoring blood pressure. In this study, an attention mechanism-based convolutional long short-term memory (LSTM) neural network is proposed to easily estimate blood pressure. To easily and comfortably estimate blood pressure, electrocardiogram (ECG) and photoplethysmography (PPG) signals are acquired. To precisely represent the characteristics of ECG and PPG signals, the signals in the time and frequency domain are selected as the inputs of the proposed NN structure. To automatically extract the features, the convolutional neural networks (CNNs) are adopted as the first part of neural networks. To identify the meaningful features, the attention mechanism is used in the second part of neural networks. To model the characteristic of time series, the long short-term memory (LSTM) is adopted in the third part of neural networks. To integrate the information of previous neural networks, the fully connected networks are used to estimate blood pressure. The experimental results show that the proposed approach outperforms CNN and CNN-LSTM and complies with the Association for the Advancement of Medical Instrumentation standard.
更多查看译文
关键词
blood pressure estimation, electrocardiogram, attention mechanism, CNN-LSTM
AI 理解论文
溯源树
样例
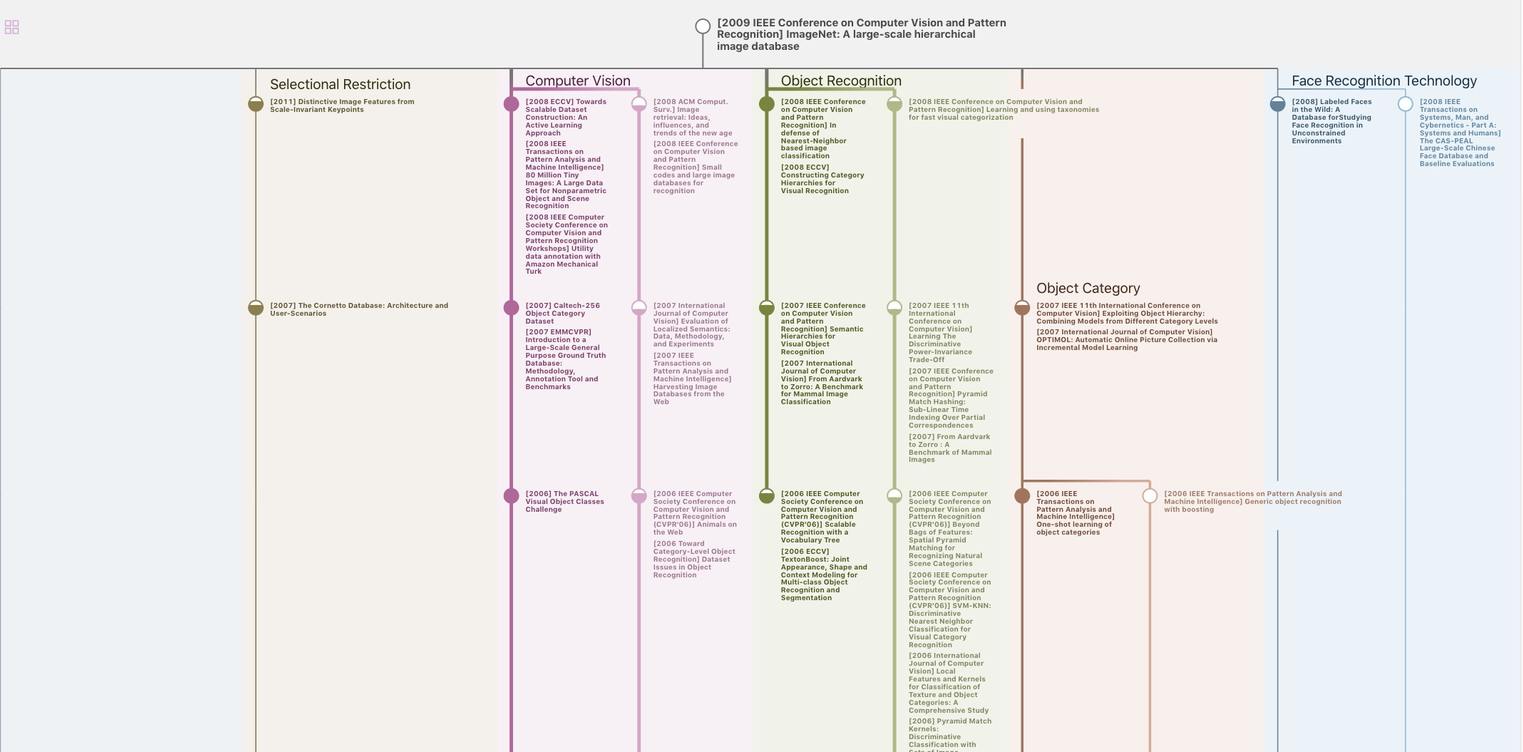
生成溯源树,研究论文发展脉络
Chat Paper
正在生成论文摘要