Enhanced decision making in multi-scenarios for autonomous vehicles using alternative bidirectional Q network
Neural Computing and Applications(2022)
摘要
To further enhance decision making in autonomous vehicles field, grant more safety, comfort, reduce traffic, and accidents, learning approaches were adopted, mainly reinforcement learning. However, possibility in upgrading these algorithms is still available due to many limitations including : convergence rate, stability, handling multiple dynamic environments, raw performance, robustness, and complexity of algorithms. To tackle these problems, we propose a novel extension of the well-known deep Q network called “alternative bidirectional Q network” that aims mainly to enhance stability and performance with improving exploration and Q values update policies, to overcome the literature gap that generally focuses on only one policy to handle decision making in multiple scenarios (avoiding obstacles, goal-oriented environments, etc.). In “alternative bidirectional Q network,” data about previous, current, and upcoming states are used to update the Q values where the actions are selected according to the relation between these data to handle several scenarios: highways, merges, roundabouts, and parking. This concept provides reinforcement learning agents with more balance between exploration and exploitation and enhances stability during learning. A gym simulator was adopted for training and testing the proposed algorithm’s outcome, while various state-of-the-art algorithms were used as benchmark models. The performance of the proposed extension was evaluated using several metrics being: loss, accuracy, speed, and reward values, where the results of comparison showed the superiority of the novel extension in all scenarios for most of the exploited metrics. The experiment results were confirmed using the complexity and the robustness aspects.
更多查看译文
关键词
Decision making, Reinforcement learning, Deep learning, Autonomous vehicles
AI 理解论文
溯源树
样例
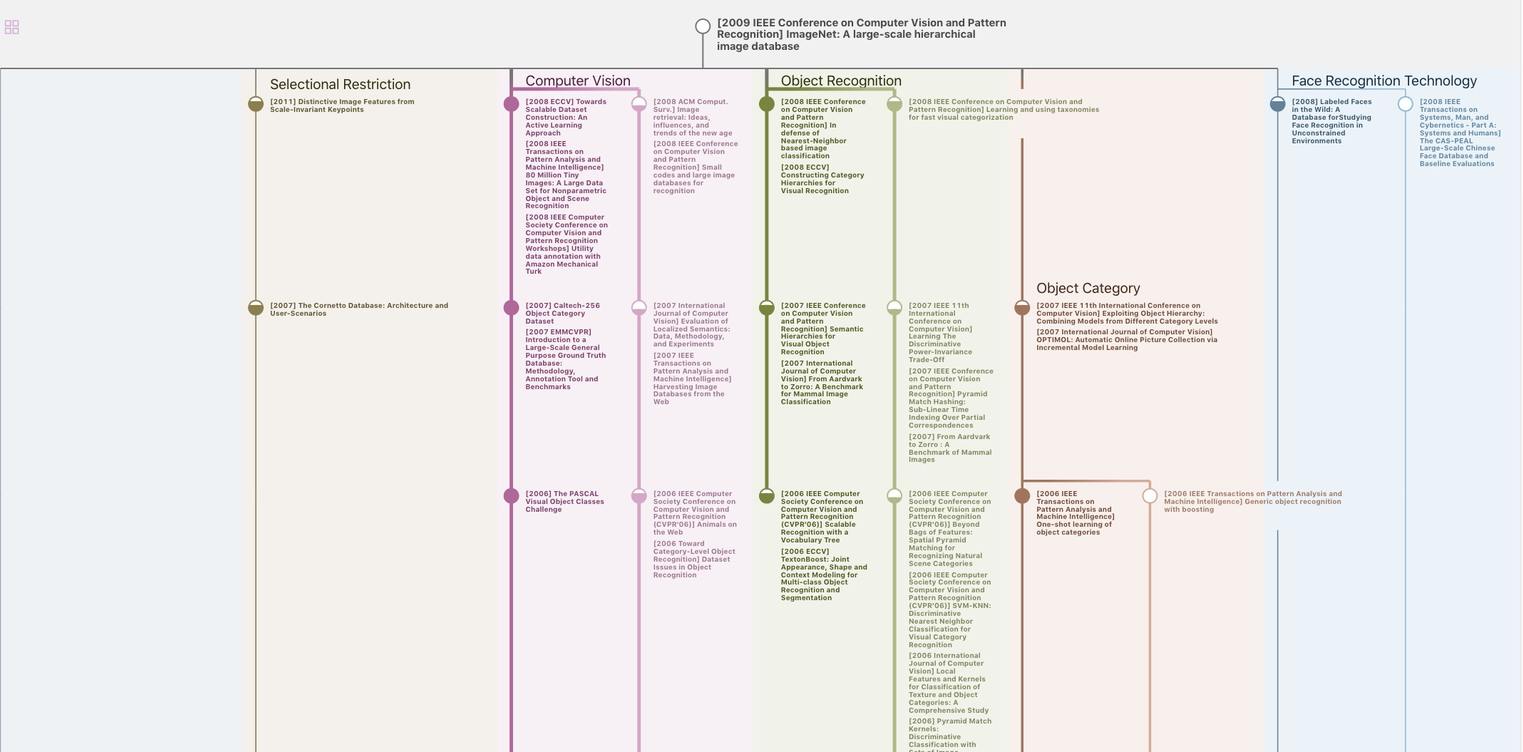
生成溯源树,研究论文发展脉络
Chat Paper
正在生成论文摘要