Feature attenuation reinforced recurrent neural network for diffusion prediction
Applied Intelligence(2022)
摘要
In recent years, prediction models based on deep learning have become more popular owing to their good prediction performance. Of them, the recurrent neural network (RNN) model has shown excellent learning and prediction ability in processing sequence data. However, in the field of information transmission, the existing models only treat the cascade process as a numerical sequence without considering the temporal characteristics of information diffusion and the difference of neighbor influence, making the prediction model unable to capture the characteristics of cascade data. We propose an information diffusion prediction approach based on feature attenuation reinforced recurrent network called Feature Deep Diffusion (FADD) to solve this problem. Firstly, a multi-order neighbor influence mechanism is introduced to distinguish the influence weights of neighbors of different classes, and the user feature representation is updated with the network representation method. Then, combining with the time attenuation effect, the neural network model based on feature attenuation enhancement is constructed. Finally, the model is used to predict information forwarding and information heat. A large set of experiments on two real social networks shows that the performance of the proposed method is better than that of the mainstream propagation prediction method based on an end-to-end neural network.
更多查看译文
关键词
Information diffusion, Activation users prediction, Cascade scale prediction, Feature attenuation, Deep learning
AI 理解论文
溯源树
样例
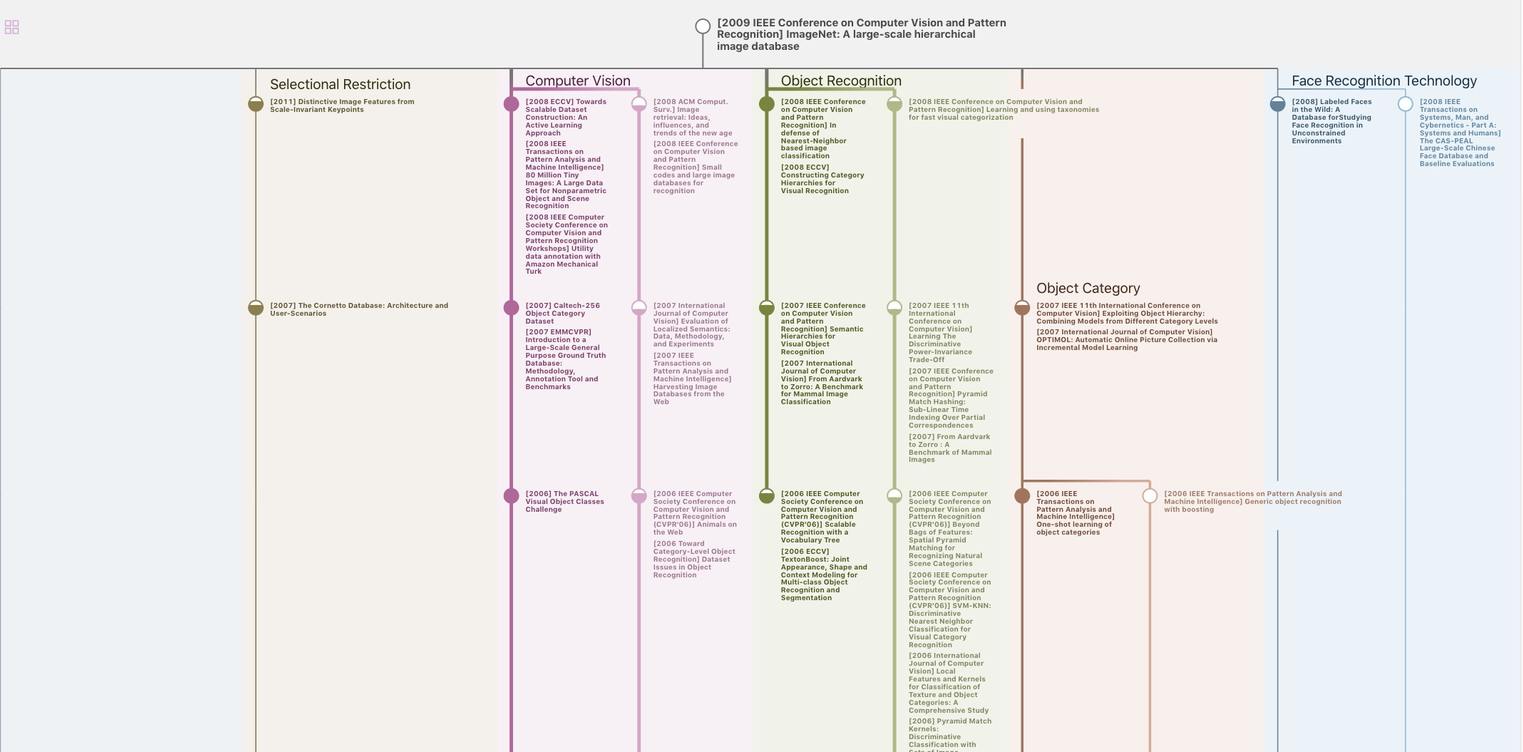
生成溯源树,研究论文发展脉络
Chat Paper
正在生成论文摘要