Recognition-oriented Facial Depth Estimation from a Single Image.
Applied intelligence(2022)
摘要
Deep learning algorithms have dramatically improved 2D face recognition, which makes 3D face recognition more promising, as 3D faces are well acknowledged to be more discriminative than 2D faces. Most deep learning algorithms for 3D face recognition are based on depth information. However, it is difficult to acquire enough depth data to meet the requirements by deep learning. There are many approaches used to reconstruct 3D faces from 2D faces, but depths derived from these 3D faces are too coarse to ensure a satisfying recognition. Therefore, this paper uses real 3D depth data to search for an optimal mapping from 2D faces to facial depths by a well-trained CycleGAN network. This can greatly assist 3D face recognition. To make the CycleGAN network more recognition-oriented, an identical cycle consistency loss is employed instead of the cycle consistency loss in CycleGAN, which typically takes the form of pixel losses. With two perceptual losses enforcing at both ends, CycleGAN is asked to preserve as much identity information as possible in both the forward and backward cycles. Furthermore, another perceptual loss is incorporated to ensure that the mapped depth can preserve the same identity as the corresponding real 3D depth. To increase the generalizability of the model in cases where the data are insufficient, this paper uses massive reconstructed depth data to pre-train the U-Nets therein. Then, the modified CycleGAN network is further trained with the ND-2006 dataset(13450 images) to finally obtain the optimal U-Net network. Extensive experiments are conducted on multiple datasets to show that the proposed method is practicable and effective.
更多查看译文
关键词
Facial depth estimation,Parameter pretraining,Identical cycle consistency loss,Identity preserving loss
AI 理解论文
溯源树
样例
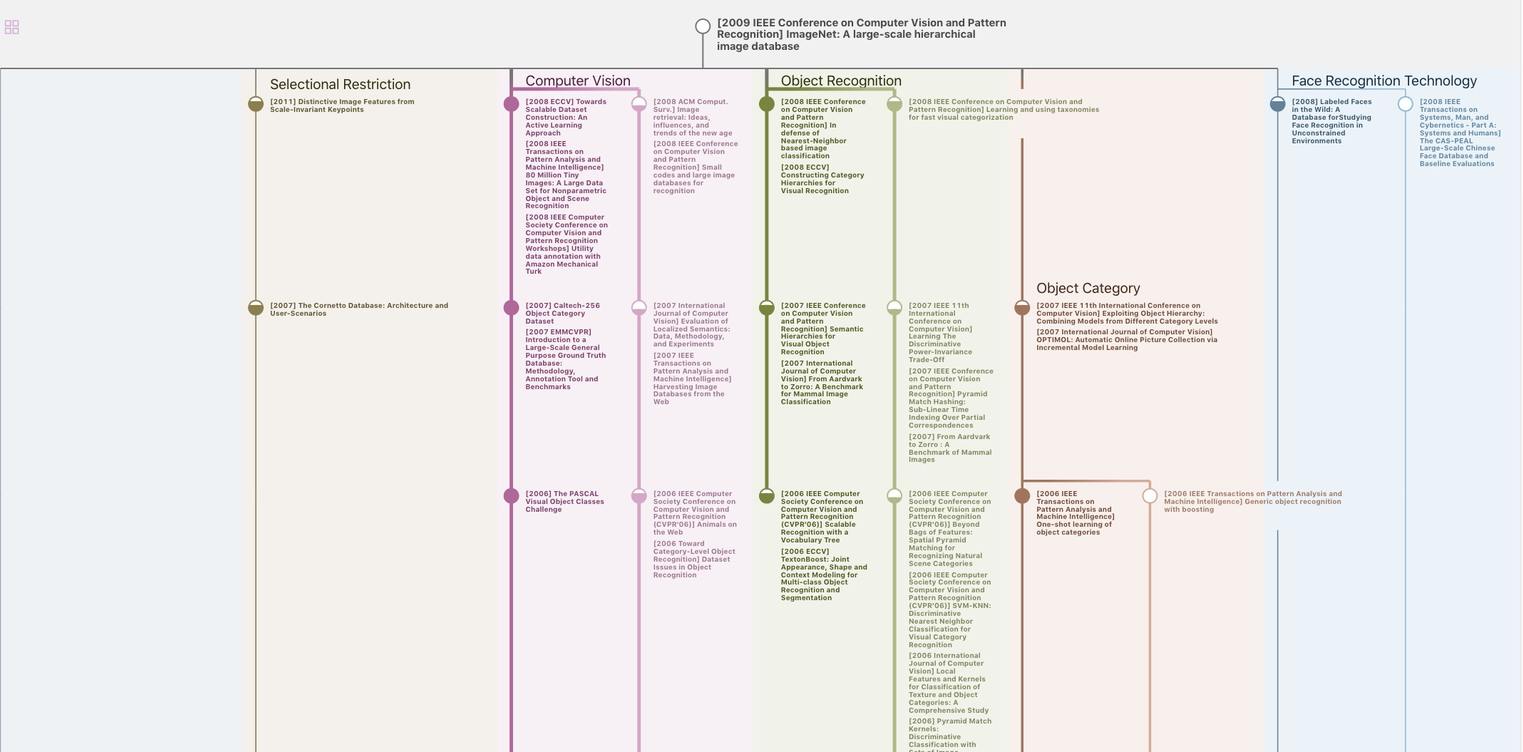
生成溯源树,研究论文发展脉络
Chat Paper
正在生成论文摘要