Data-driven model-based calibration for optimizing electrically boosted diesel engine performance
INTERNATIONAL JOURNAL OF ENGINE RESEARCH(2023)
摘要
Engine calibration is a process of optimizing its control variables, which is a crucial step to achieve the desired performance from the engine system. However, its complexity has increased dramatically due to the constant evolution of the engine architecture with multiple control variables interacting with each other, making it difficult to calibrate its control variables with significantly increased experimental costs. System complexity also affects the physics-based modeling process as it makes the system model highly non-linear and difficult to capture system dynamics under all engine operating conditions. To overcome these challenges, the current work proposes a machine learning-based framework to reduce the required calibration effort. The work implements a stochastic surrogate assisted optimization approach on an actual engine experimental test setup, which efficiently looks for the global optimal solution based on only the input-output data set. This work performs a steady-state calibration of a turbocharged diesel engine with an additional external electric-assisted boosting system (eBoost). Three control parameters are considered for this study: Variable Geometry Turbocharger (VGT) vane position, Exhaust Gas Recirculation (EGR) valve position, and eBoost speed to identify the optimal trade-off between engine efficiency (Brake Specific Fuel Consumption) and emissions (NO x ). The addition of eBoost makes the calibration problem difficult because the two control parameters, VGT and EGR, also depend on eBoost speed. Using the proposed data-driven calibration approach, the experimental results showed enhanced engine performance in terms of efficiency and emissions using the eBoost compared with the case without eBoost. At the same time, the experimental cost was reduced significantly due to the reduced number of testing points required to achieve the optimum.
更多查看译文
关键词
Engine calibration, stochastic optimization, surrogate model, constrained multi-objective optimization, data-driven control
AI 理解论文
溯源树
样例
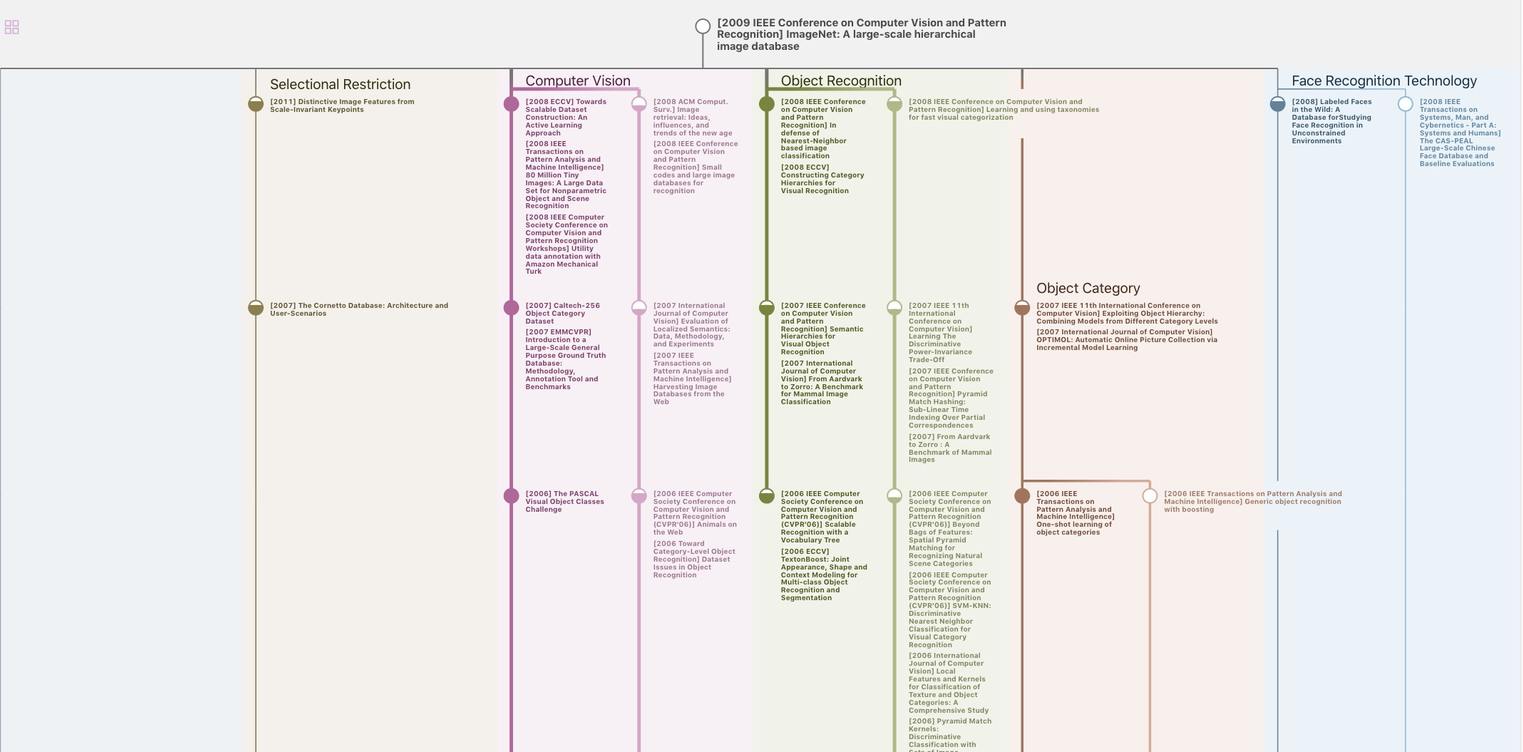
生成溯源树,研究论文发展脉络
Chat Paper
正在生成论文摘要