Prediction of electron ionization mass spectra based on graph convolutional networks
International Journal of Mass Spectrometry(2022)
摘要
Putative identification of metabolites is comparing the observed mass spectrum of the sample to a reference library. However, the existing libraries cannot contain all the mass spectra due to the huge number of compounds. The identification process will fail if the target mass spectrum does not exist in the library. One solution is augmenting the library with synthetic spectra. Previous work uses neural networks to predict the electron ionization mass spectra (EI-MS) of small compounds from their extended-connectivity fingerprints (ECFPs). But some molecular structural information may be lost during the process of generating ECFPs. In this paper, we proposed a deep learning model that uses graph convolutional networks to extract molecular features and predict EI-MS. This model is an end-to-end method that operates directly on the graphs of molecules, so it is an excellent way to avoid the loss of structural information. The experimental results show that the performance of the proposed model beats the previously reported methods on the task of EI-MS prediction.
更多查看译文
关键词
Mass Spectrometry、Graph convolutional network(GCN)、Compound identification、Deep learning、Features extraction
AI 理解论文
溯源树
样例
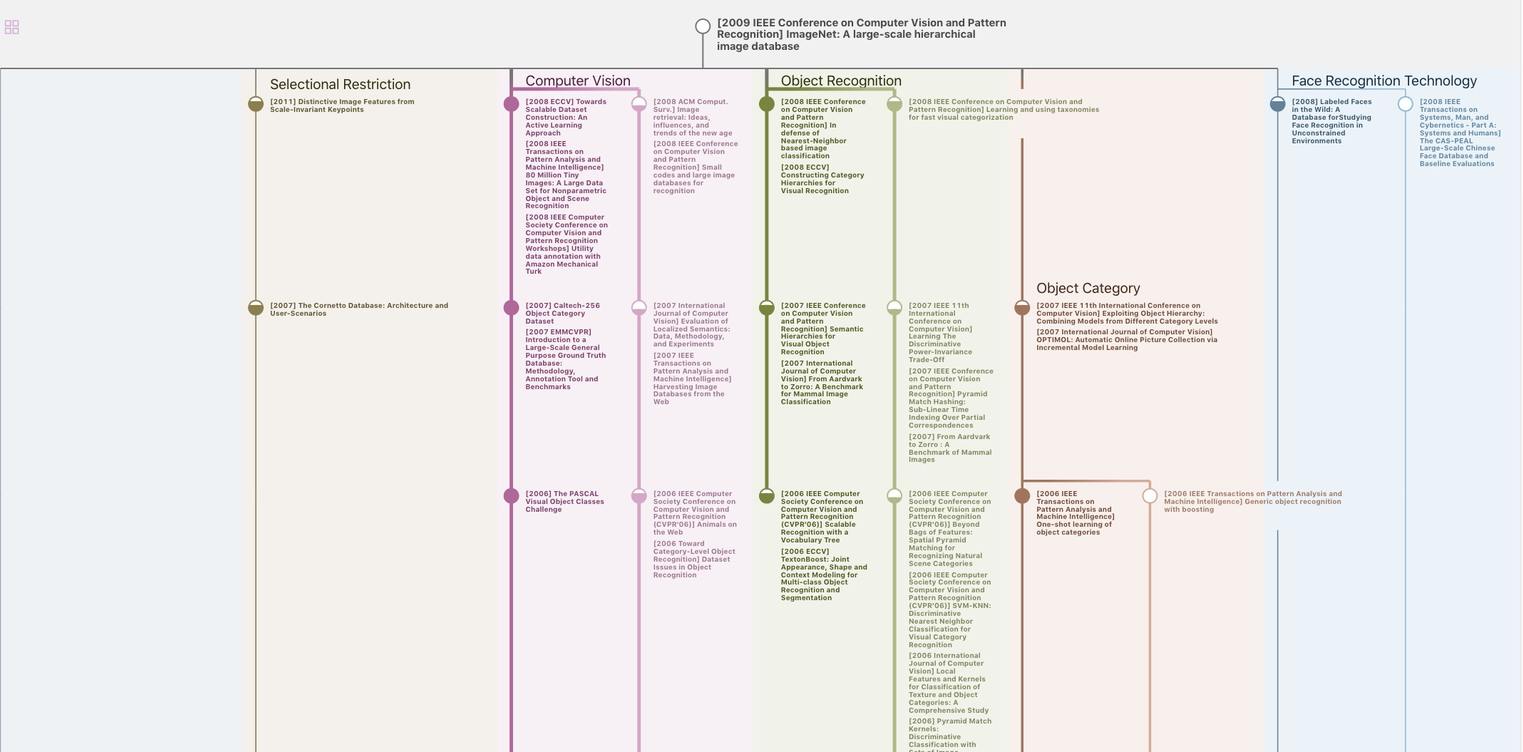
生成溯源树,研究论文发展脉络
Chat Paper
正在生成论文摘要