Sparsifying the least-squares approach to PCA: comparison of lasso and cardinality constraint
ADVANCES IN DATA ANALYSIS AND CLASSIFICATION(2022)
摘要
Sparse PCA methods are used to overcome the difficulty of interpreting the solution obtained from PCA. However, constraining PCA to obtain sparse solutions is an intractable problem, especially in a high-dimensional setting. Penalized methods are used to obtain sparse solutions due to their computational tractability. Nevertheless, recent developments permit efficiently obtaining good solutions of cardinality-constrained PCA problems allowing comparison between these approaches. Here, we conduct a comparison between a penalized PCA method with its cardinality-constrained counterpart for the least-squares formulation of PCA imposing sparseness on the component weights. We compare the penalized and cardinality-constrained methods through a simulation study that estimates the sparse structure’s recovery, mean absolute bias, mean variance, and mean squared error. Additionally, we use a high-dimensional data set to illustrate the methods in practice. Results suggest that using cardinality-constrained methods leads to better recovery of the sparse structure.
更多查看译文
关键词
Cardinality constraint,Sparse PCA,Penalized linear regression
AI 理解论文
溯源树
样例
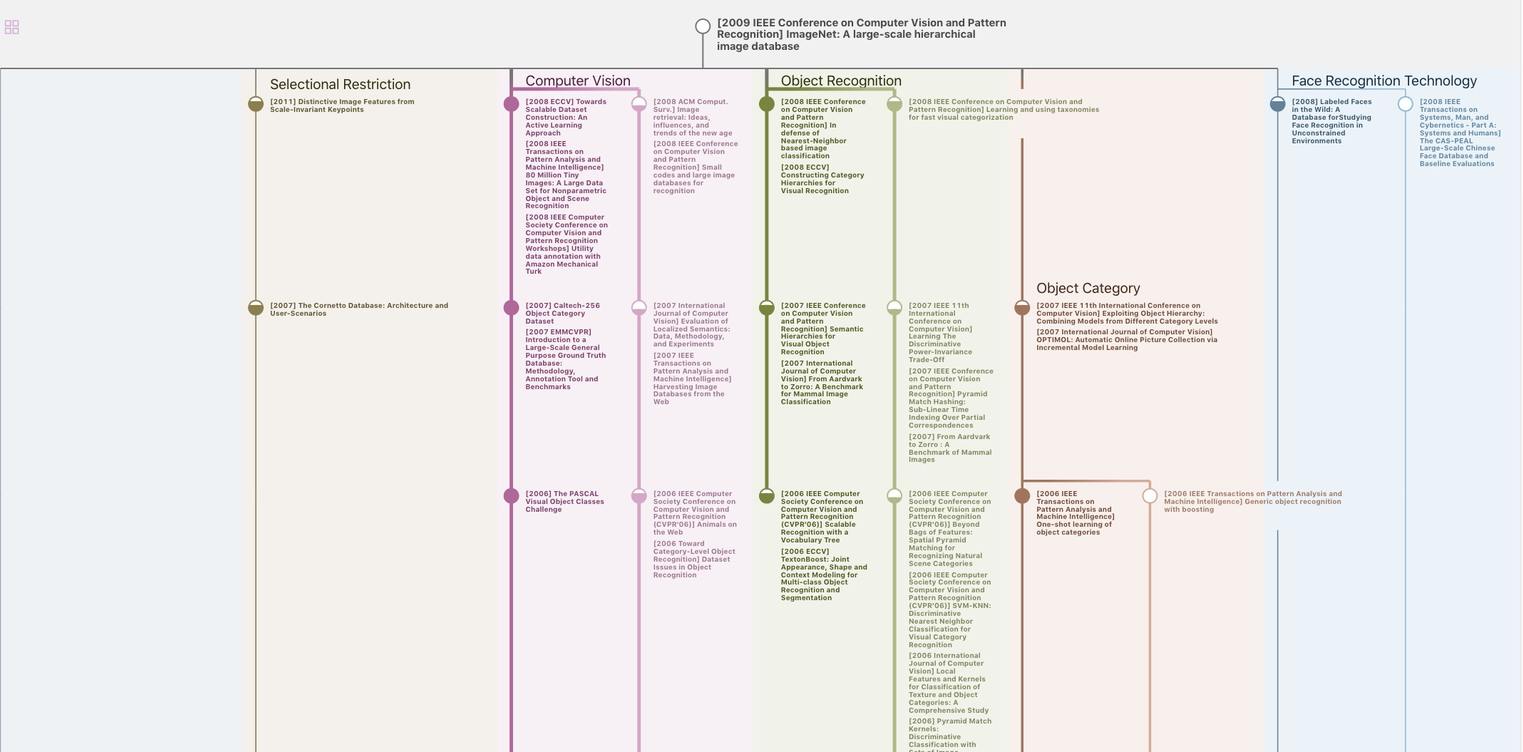
生成溯源树,研究论文发展脉络
Chat Paper
正在生成论文摘要