Transfer Learning Assisted GPHH for Dynamic Multi-Workflow Scheduling in Cloud Computing
AI 2021: ADVANCES IN ARTIFICIAL INTELLIGENCE(2022)
摘要
Dynamic Multi-Workflow Scheduling (DWS) in cloud is a challenging optimisation due to the dynamic nature of the problem. It requires complex mapping decisions to be made under unknown dynamic events. Genetic Programming Hyper-Heuristic (GPHH) has been successfully applied to generate scheduling heuristics for DWS due to its flexible representation. However, the simulation-based evaluation is computationally expensive due to calculations required by individuals to make decisions in the simulation. To improve efficiency, this paper proposes a novel Transfer Learning (TL) Assisted GPHH (TL-GPHH). Specifically, TL-GPHH is designed and compared with Non-Assisted GPHH to generate effective heuristic rules in less time. The results show that the proposed algorithm generates heuristics that can minimise makespan in more scenarios than Non-Assisted GPHH and other state-of-the-art heuristics. Moreover, the proposed algorithm can reduce the computational costs of GP without sacrificing the performance.
更多查看译文
关键词
Genetic Programming Hyper Heuristic, Dynamic Multi-Workflow Scheduling, Cloud computing, Transfer Learning
AI 理解论文
溯源树
样例
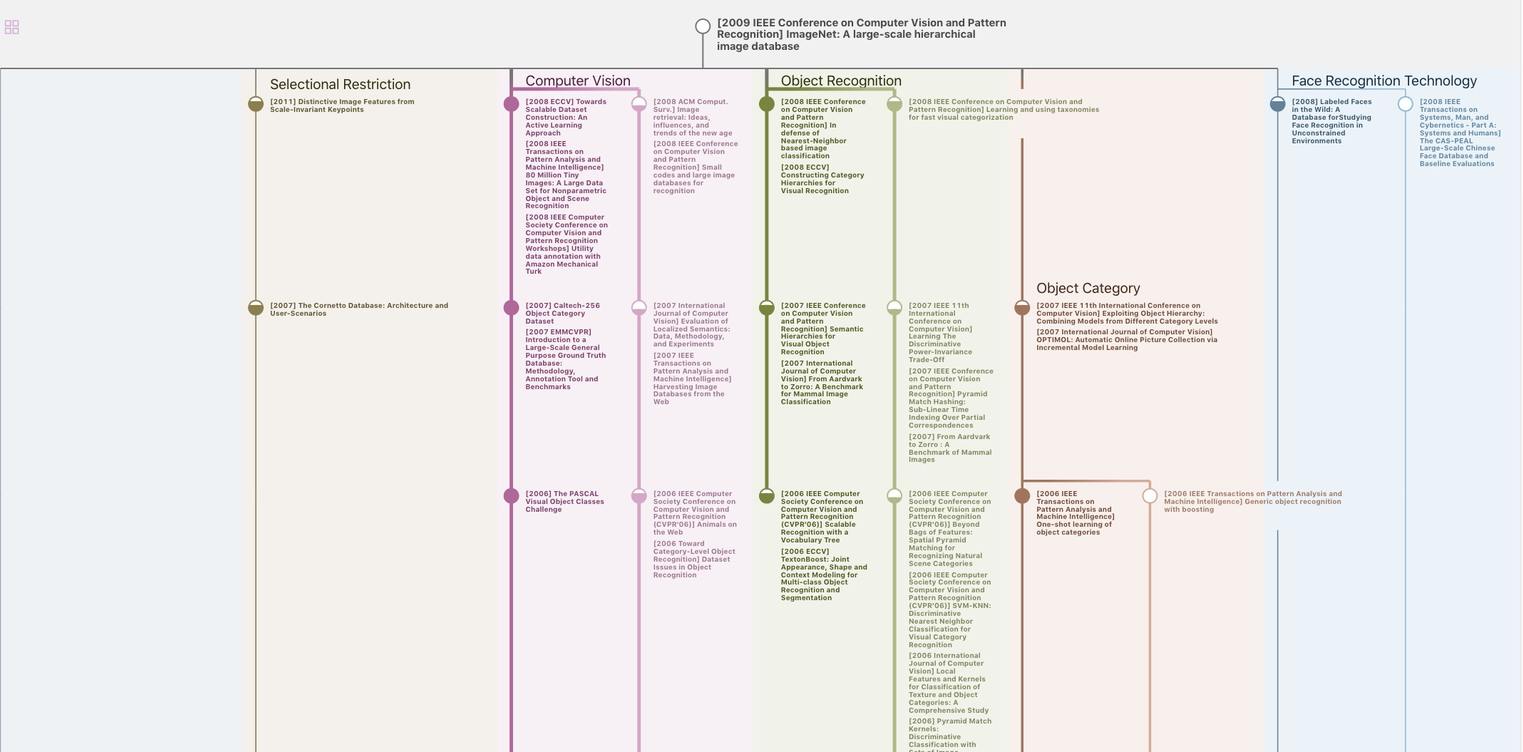
生成溯源树,研究论文发展脉络
Chat Paper
正在生成论文摘要