CDeRSNet: Towards High Performance Object Detection in Vietnamese Document Images
MultiMedia Modeling(2022)
摘要
In recent years, document image understanding (DIU) has received much attention from the research community. Localizing page objects (tables, figures, equations) in document images is an important problem in DIU, which is the foundation for extracting information from document images. However, it has remained many challenges due to the high degree of intra-class variability in page document. Especially, object detection in Vietnamese image documents has still limited. In this paper, we propose CDeRSNet: a novel end-to-end trainable deep learning network to solve object detection in Vietnamese documents. The proposed network consists of Cascade R-CNN with the deformable convolution backbone and Rank & Sort (RS) Loss. CDeRSNet detects objects varying in scale with high detection accuracy at a higher IoU threshold to localize objects that differ in scale with detection accuracy at high quality. We empirically evaluate CDeRSNet on the Vietnamese image document dataset - UIT-DODV with four classes of objects: table, figure, caption, and formula. We achieved the best performance on the UIT-DODV dataset with 79.9% in terms of mAP, which is higher 5.4% than current results. In addition, we also provide a comprehensive evaluation and insightful analysis of CDeRSNet. Finally, we demonstrate CDeRSNet outperformance over state-of-the-arts models in object detection such as GFocal, GFocalV2, VFNet, DetectoRS on the UIT-DODV dataset. Code can be available at:
https://github.com/trongthuan205/CDeRSNet.git
.
更多查看译文
关键词
Object detection, CDeRSNet, RSLoss
AI 理解论文
溯源树
样例
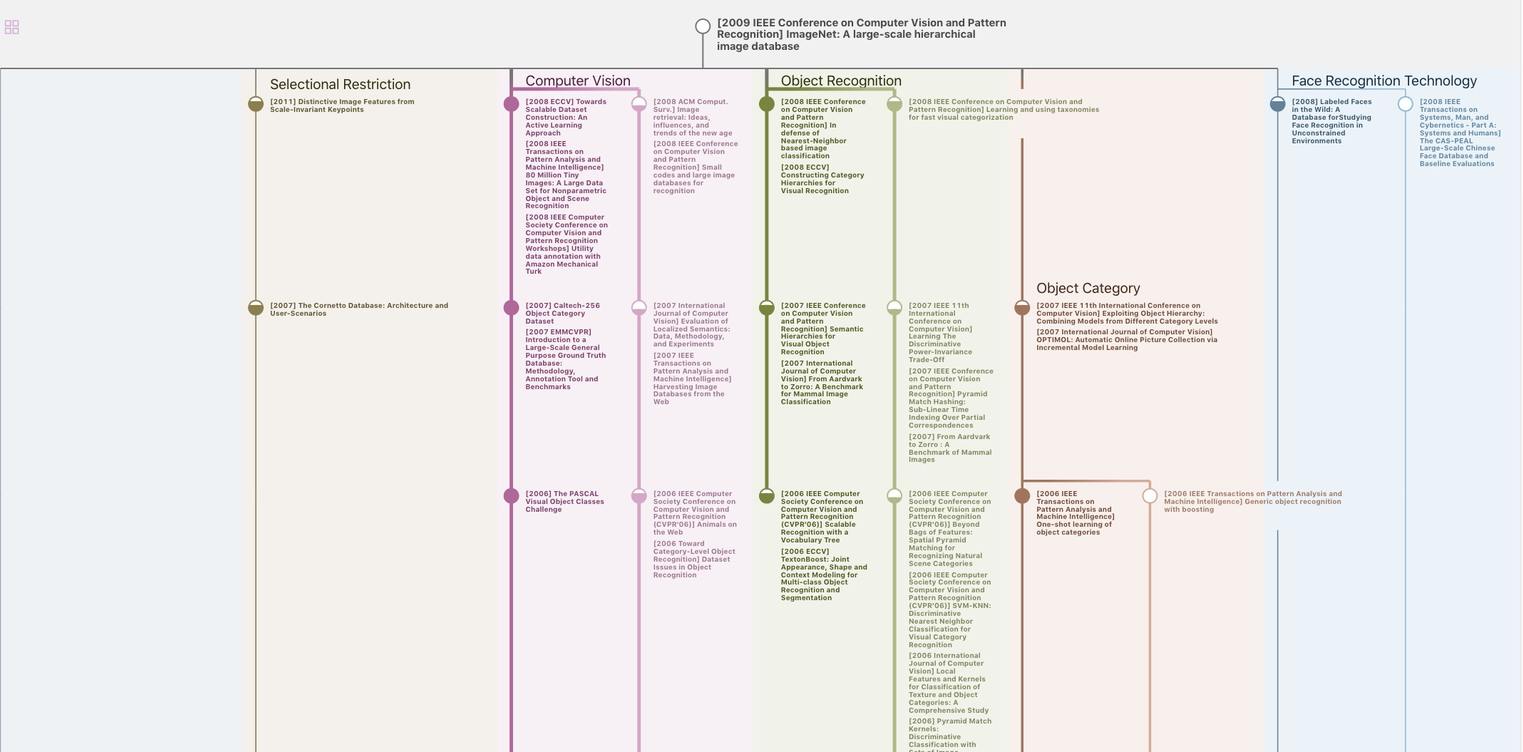
生成溯源树,研究论文发展脉络
Chat Paper
正在生成论文摘要