DIG: A Data-Driven Impact-Based Grouping Method for Video Rebuffering Optimization
MULTIMEDIA MODELING, MMM 2022, PT II(2022)
摘要
Rebuffering is known to be the dominant metric that affects the user experience of video streaming applications. In this paper, we propose a data-driven impact-based grouping (DIG) method for video rebuffering optimization. By analyzing data of 74 5 million video sessions collected in a real video streaming system, several key features with most significant and temporally persistent impact on video rebuffering are identified. Based on the values of these features, similar video sessions are grouped together. Within each group, we forecast future rebuffering events via a simple and efficient model, exploiting the insight that all video sessions in the same group face a similar risk of rebuffering. If rebuffering is predicted to happen in a coming session, we try to avoid it by selecting a better content distribution network (CDN) for this video. Experimental results show that our method can successfully predict 46% of the rebuffering sessions, and reduce the average rebuffering rate by 18.4%.
更多查看译文
关键词
Video rebuffering, Feature ranking, CDN selection
AI 理解论文
溯源树
样例
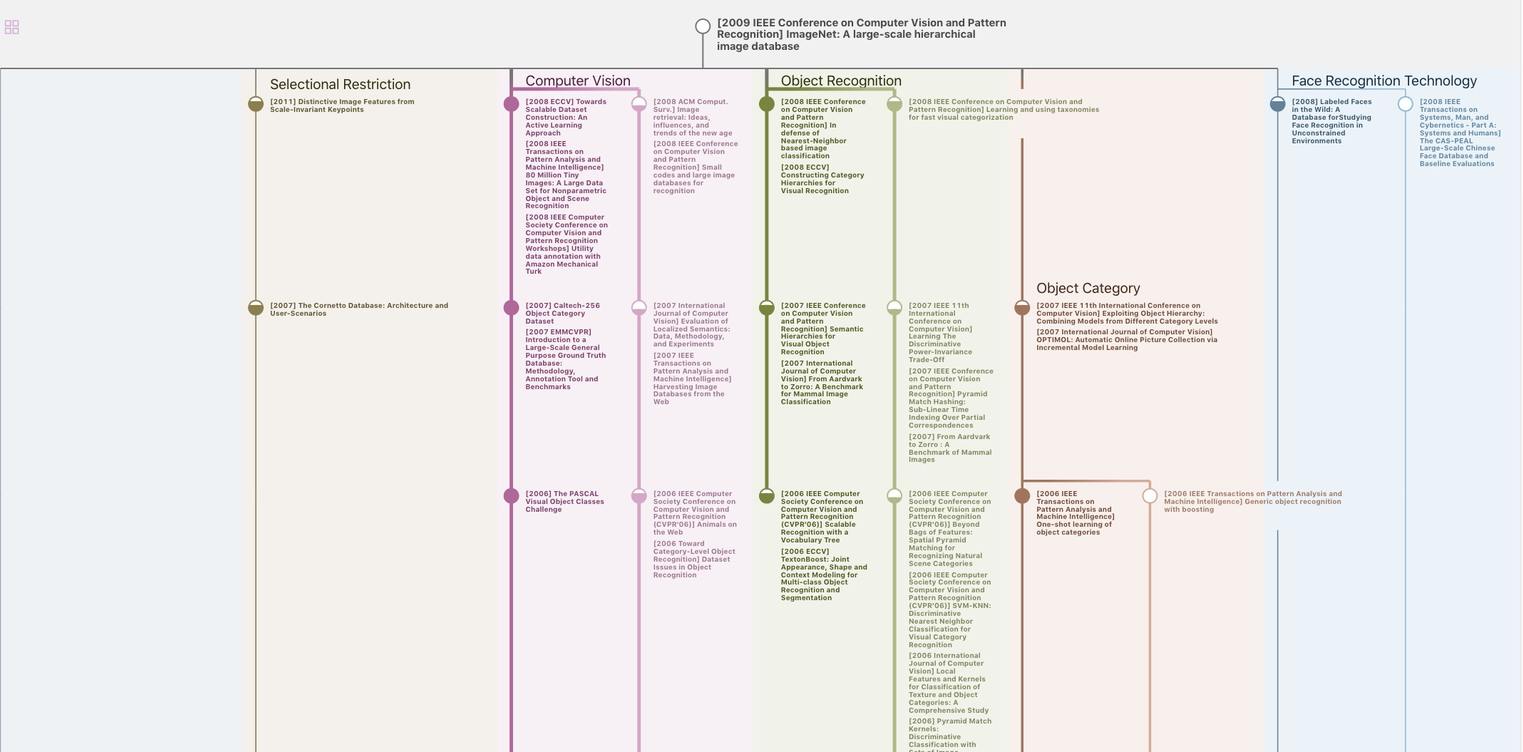
生成溯源树,研究论文发展脉络
Chat Paper
正在生成论文摘要