Fault prediction of combine harvesters based on stacked denoising autoencoders
INTERNATIONAL JOURNAL OF AGRICULTURAL AND BIOLOGICAL ENGINEERING(2022)
摘要
Accurate fault prediction is essential to ensure the safety and reliability of combine harvester operation. In this study, a combine harvester fault prediction method based on a combination of stacked denoising autoencoders (SDAE) and multi-classification support vector machines (SVM) is proposed to predict combine harvester faults by extracting operational features of key combine components. In general, SDAE contains autoencoders and uses a deep network architecture to learn complex non-linear input-output relationships in a hierarchical manner. Selected features are fed into the SDAE network, deep-level features of the input parameters are extracted by SDAE, and an SVM classifier is then added to its top layer to achieve combine harvester fault prediction. The experimental results show that the method can achieve accurate and efficient combine harvester fault prediction. In particular, the experiments used Gaussian noise with a distribution center of 0.05 to corrupt the test data samples obtained by random sampling of the whole population, and the results showed that the prediction accuracy of the method was 95.31%, which has better robustness and generalization ability compared to SVM (77.03%), BP (74.61%), and SAE (90.86%).
更多查看译文
关键词
fault prediction, combine harvester, stacked denoising autoencoders, support vector machines
AI 理解论文
溯源树
样例
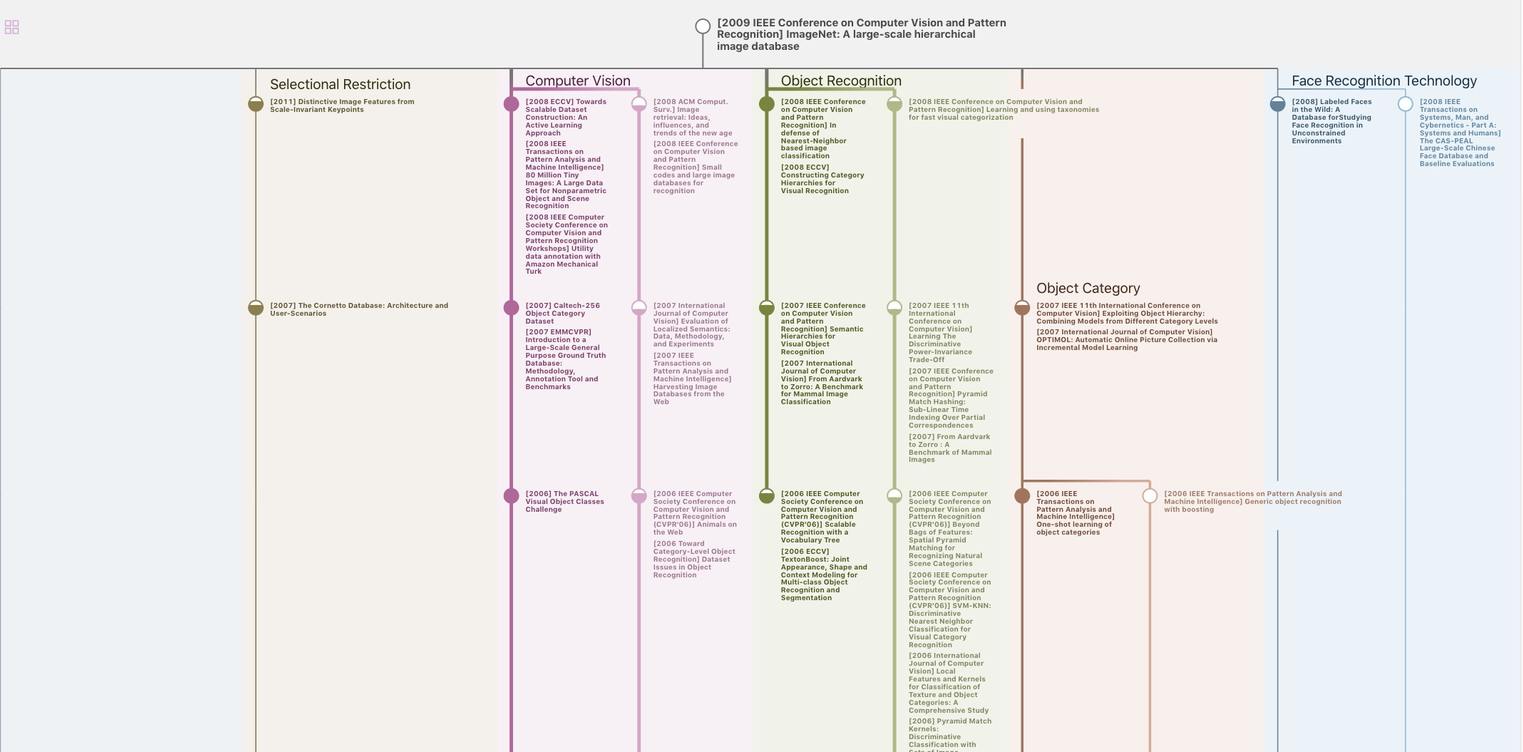
生成溯源树,研究论文发展脉络
Chat Paper
正在生成论文摘要