A Complementary Fusion Strategy for RGB-D Face Recognition
MultiMedia Modeling(2022)
摘要
RGB-D Face Recognition (FR) with low-quality depth maps recently plays an important role in biometric identification. Intrinsic geometry properties and shape clues reflected by depth information significantly promote the FR robustness to light and pose variations. However, the existing multi-modal fusion methods mostly lack the ability of complementary feature learning and establishing correlated relationships between different facial features. In this paper, we propose a Complementary Multi-Modal Fusion Transformer (CMMF-Trans) network which is able to complement the fusion while preserving the modal-specific properties. In addition, the proposed novel tokenization and self-attention modules stimulate the Transformer to capture long-range dependencies supplementary to local representations of face areas. We test our model on two public datasets: Lock3DFace and IIIT-D which contain challenging variations in pose, occlusion, expression and illumination. Our strategy achieves the state-of-the-art performance on them. Another meaningful contribution in our work is that we have created a challenging RGB-D FR dataset which contains more kinds of difficult scenarios, such as, mask occlusion, backlight shadow, etc.
更多查看译文
关键词
RGB-D face recognition, Complementary multi-modal fusion, Transformer, Long-range dependencies
AI 理解论文
溯源树
样例
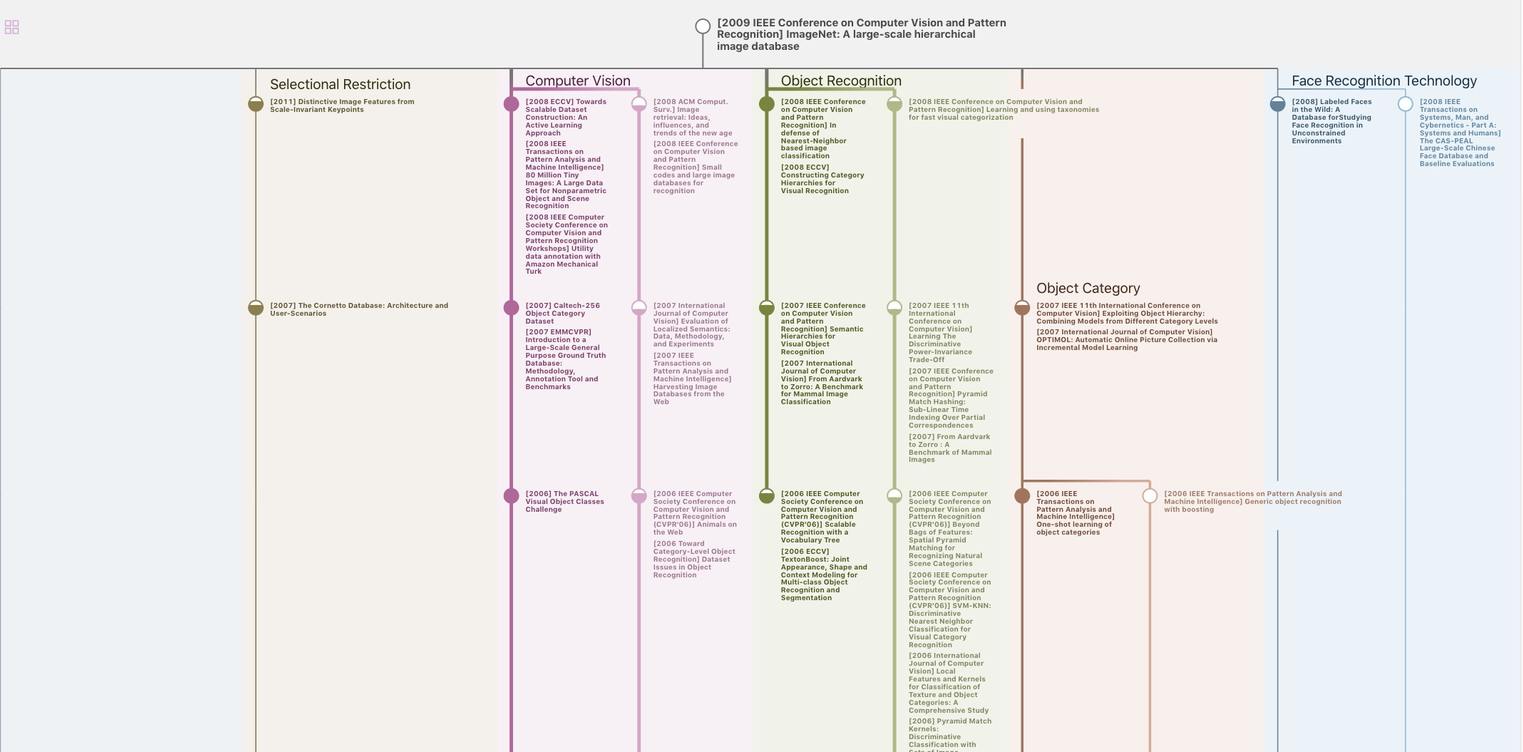
生成溯源树,研究论文发展脉络
Chat Paper
正在生成论文摘要