Borderline-margin loss based deep metric learning framework for imbalanced data
Applied Intelligence(2022)
摘要
The imbalanced data suffer the problem where minority class is under-represented compared with majority ones. Traditional imbalanced learning algorithms only consider the class imbalance while ignoring the class overlap, which leads to an undesirable accuracy for minority samples in overlapping regions. Considering the above issue, we propose a deep metric framework with borderline-margin loss (DMFBML) for improving the intra-class coherence and inter-class difference in overlapping regions. Firstly, a flexible borderline margin is designed for each minority sample, which is adaptively adjusted according to the neighborhood’s label. The proposed margin enables to discriminate minority samples with varying overlap degrees, which significantly preserves the valuable information of classification boundary. The input data is then reconstructed into training triplets set to generate more metric constraints for minority samples, thereby increasing the difference in overlapping regions. Finally, a neural network with DMFBML is presented to achieve a better classifier performance on imbalanced data. The proposed method is verified by the comparative experiments on six synthetic datasets and eleven actual datasets.
更多查看译文
关键词
Imbalanced classification, Class imbalance, Class overlap, Deep metric framework, Borderline-margin loss
AI 理解论文
溯源树
样例
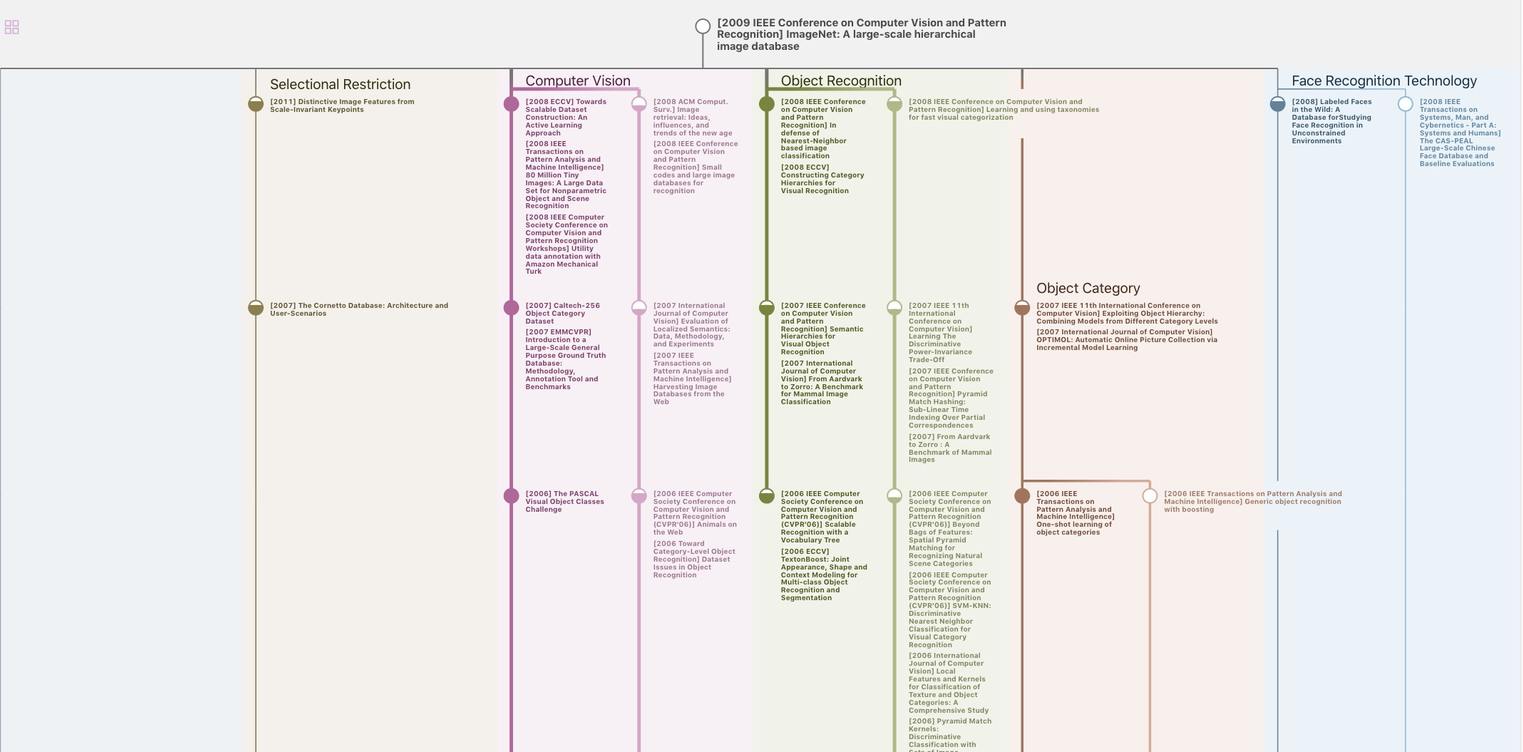
生成溯源树,研究论文发展脉络
Chat Paper
正在生成论文摘要