Low Cloud Detection in Multilayer Scenes Using Satellite Imagery with Machine Learning Methods
JOURNAL OF ATMOSPHERIC AND OCEANIC TECHNOLOGY(2022)
摘要
The detection of multilayer clouds in the atmosphere can be particularly challenging from passive visible and infrared imaging radiometers since cloud boundary information is limited primarily to the topmost cloud layer. Yet detection of low clouds in the atmosphere is important for a number of applications, including aviation nowcasting and general weather forecasting. In this work, we develop pixel-based machine learning-based methods of detecting low clouds, with a focus on improving detection in multilayer cloud situations and specific attention given to improving the Cloud Cover Layers (CCL) product, which assigns cloudiness in a scene into vertical bins. The random forest (RF) and neural network (NN) implementations use inputs from a variety of sources, including GOES Advanced Baseline Imager (ABI) visible radiances, infrared brightness temperatures, auxiliary information about the underlying surface, and relative humidity (which holds some utility as a cloud proxy). Training and independent validation enlists near-global, actively sensed cloud boundaries from the radar and lidar systems on board the CloudSat and CALIPSO satellites. We find that the RF and NN models have similar performances. The probability of detection (PoD) of low cloud increases from 0.685 to 0.815 when using the RF technique instead of the CCL methodology, while the false alarm ratio decreases. The improved PoD of low cloud is particularly notable for scenes that appear to be cirrus from an ABI perspective, increasing from 0.183 to 0.686. Various extensions of the model are discussed, including a nighttime-only algorithm and expansion to other satellite sensors.& nbsp;
更多查看译文
关键词
Clouds, Cloud retrieval, <p>Lidars/Lidar observations</p>, <p>Radars/Radar observations</p>, Remote sensing, Satellite observations, Nowcasting, Machine learning
AI 理解论文
溯源树
样例
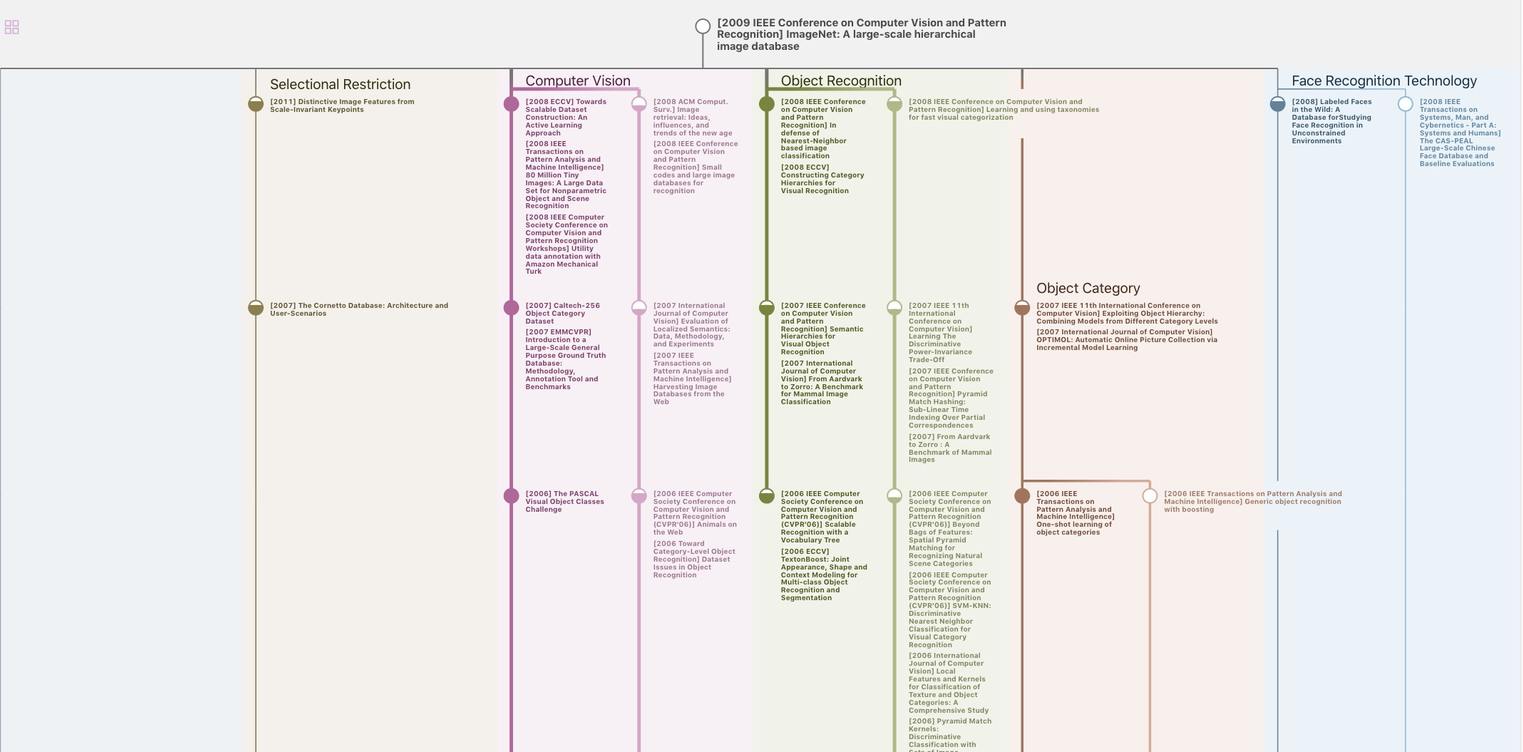
生成溯源树,研究论文发展脉络
Chat Paper
正在生成论文摘要