Using online student interactions to predict performance in a first-year computing science course
TECHNOLOGY PEDAGOGY AND EDUCATION(2022)
摘要
Student performance is a critical factor in determining a university's reputation because it has a negative effect on student retention. Students who do not perform well in a course are more likely to drop out from their programmes before graduating. Many students who enrol in Computing Science programmes struggle to find success because it is considered a difficult discipline. In this study, a sample of 918 observations were selected containing demographic and academic information about students enrolled in a first-year undergraduate Computing Science course at a university. Classification algorithms such as Decision Tree, Random Forest, Naive Bayes and Support Vector Machine were used to build predictive models to determine whether a student will pass or fail the course. The results showed the Random Forest algorithms are capable of producing better predictive performance compared with traditional Decision Tree algorithms.
更多查看译文
关键词
Predictive analytics, data mining, classification algorithms, random forest algorithm, decision trees
AI 理解论文
溯源树
样例
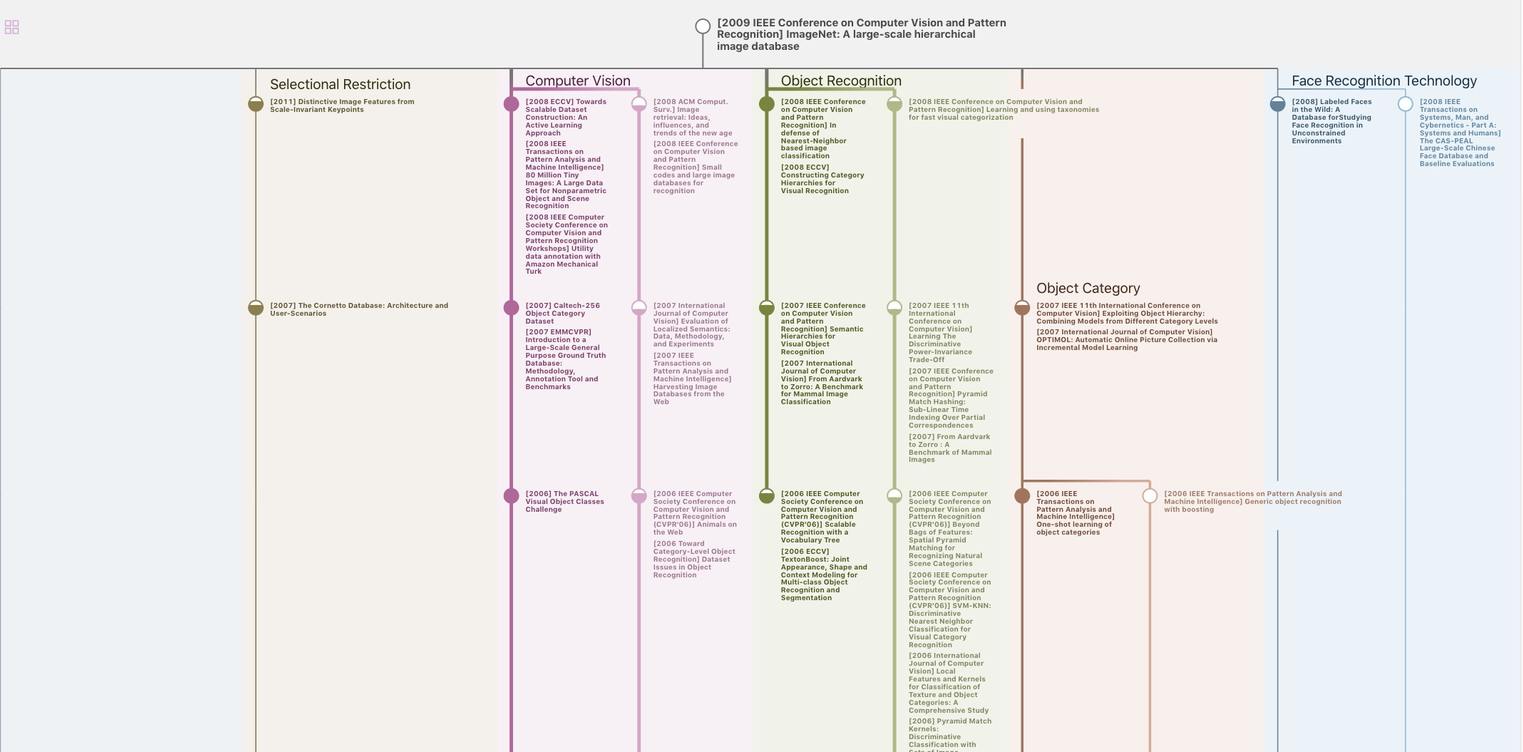
生成溯源树,研究论文发展脉络
Chat Paper
正在生成论文摘要