HASIC-Net: Hybrid Attentional Convolutional Neural Network With Structure Information Consistency for Spectral Super-Resolution of RGB Images
IEEE TRANSACTIONS ON GEOSCIENCE AND REMOTE SENSING(2022)
摘要
Spectral super-resolution (SSR), referring to the recovery of a reasonable hyperspectral image (HSI) from a single RGB image, has achieved satisfactory performance as part of the continued development of a convolutional neural network (CNN) in remote sensing image processing. However, the majority of existing algorithms focus on the pursuit of networks with deeper or broader architecture. Such algorithms have a poor channel or band feature extraction and fusing performance, and fail to fully leverage the input RGB images. To overcome these issues, we present a novel hybrid attentional CNN with structure information consistency (HASIC-net) that uses a two-pathway architecture. Specifically, both sides are stacked with several 2-D residual groups (2-DRGs) and residual groups (1-DRGs) equipped with channel or band attention (BA) modules, which mainly focuses on extracting channel statistics and bandwise features, respectively, by a parallel pooling architecture. We introduce several transversal connections from 2-DRG to 1-DRG to realize the interaction of information flow between both sides. In addition, we take the structure information of both RGB images and HSI into consideration and devise a structure information consistency (SIC) module to merge the structure tensor prior to the RGB images with the input of each 2-DRG. We then combine spectral gradient constraint loss with mean relative absolute error as a novel loss function to further restrain the spectral distortion and smooth the reconstructed spectral response curves. Experimental results on four benchmark datasets (i.e., NTIRE 2020, NTIRE 2018, CAVE, and Harvard) demonstrate that our proposed HASIC-net achieves state-of-the-art performance.
更多查看译文
关键词
Image reconstruction, Convolutional neural networks, Superresolution, Encoding, Feature extraction, Silicon carbide, Hyperspectral imaging, Channel or band attention (BA) modules (CBAMs), spectral gradient constraint, spectral super-resolution (SSR), structure information consistency (SIC)
AI 理解论文
溯源树
样例
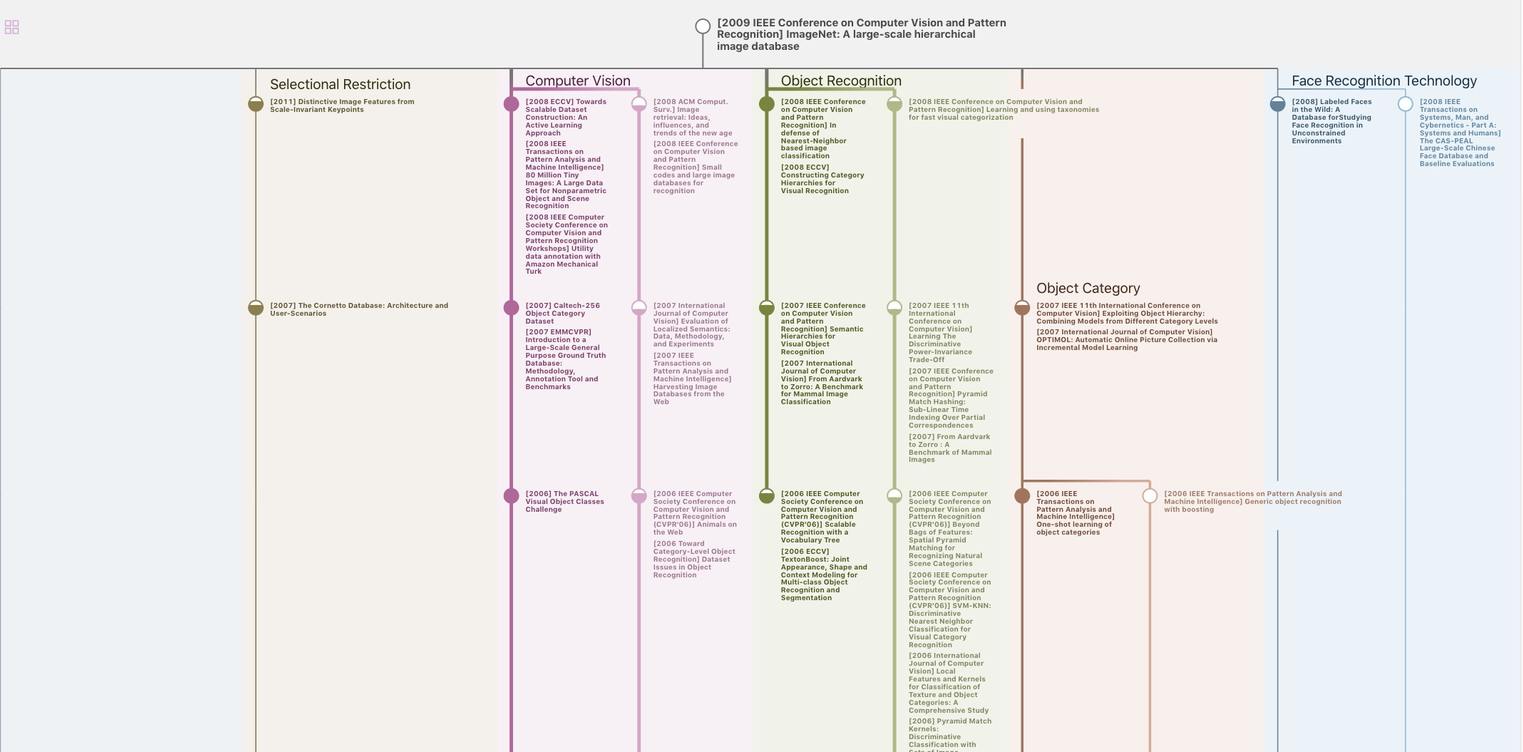
生成溯源树,研究论文发展脉络
Chat Paper
正在生成论文摘要