Why Did My Consumer Shop? Learning an Efficient Distance Metric for Retailer Transaction Data
MACHINE LEARNING AND KNOWLEDGE DISCOVERY IN DATABASES APPLIED DATA SCIENCE AND DEMO TRACK, ECML PKDD 2020, PT V(2021)
摘要
Transaction analysis is an important part in studies aiming to understand consumer behaviour. The first step is defining a proper measure of similarity, or more specifically a distance metric, between transactions. Existing distance metrics on transactional data are built on retailer specific information, such as extensive product hierarchies or a large product catalogue. In this paper we propose a new distance metric that is retailer independent by design, allowing cross-retailer and cross-country analysis. The metric comes with a novel method of finding the importance of categories of products, alternating between unsupervised learning techniques and importance calibration. We test our methodology on a real-world dataset and show how we can identify clusters of consumer behaviour.
更多查看译文
关键词
Distance metric,Transaction categorization,Clustering,Optimization
AI 理解论文
溯源树
样例
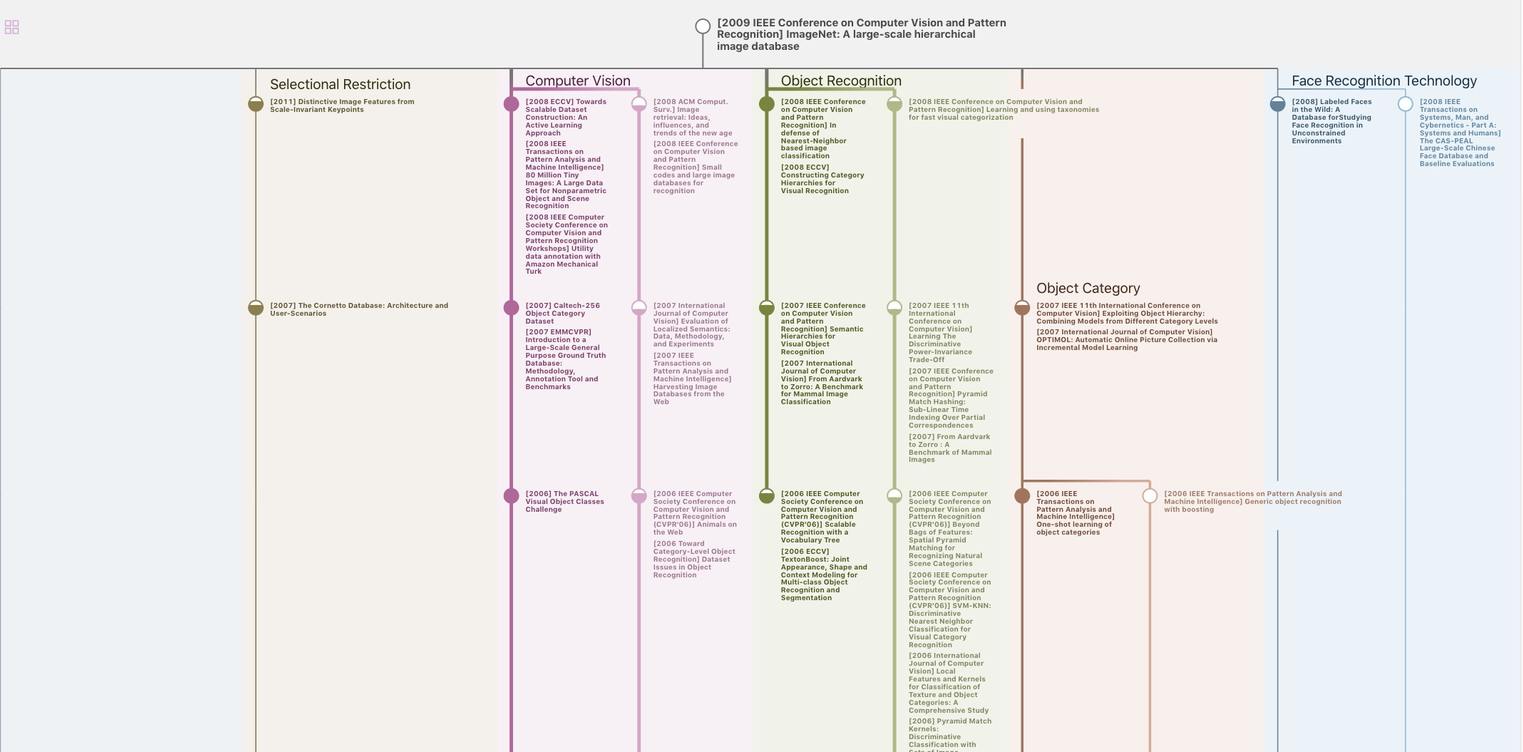
生成溯源树,研究论文发展脉络
Chat Paper
正在生成论文摘要