Farmland Parcel-Based Crop Classification in Cloudy/rainy Mountains Using Sentinel-1 and Sentinel-2 Based Deep Learning
International journal of remote sensing(2022)
摘要
Multitemporal remote sensing data, especially those for key phenological periods, play an important role in crop classification. However, cloudy/rainy climate conditions can easily lead to a lack of valid optical data, leading to crop classification difficulties. A general solution is taking advantage of all-weather synthetic aperture radar (SAR) datasets. In practice, SAR and optical datasets are often applied in the agricultural field by the method of image fusion, but it is difficult to apply when the number of optical images is too small. To solve this problem, this research proposes a data-transfer and feature-optimize-based method, which deploy an RNN-based encoding-decoding network to add additional data to the 'optical' temporal features at the farmland parcel scale and improve the utilization of optical fragments. On the basis of this method, we mitigate inconsistencies in spatial scale among different datasets and optimize the time-series parameters without expert knowledge in the crop classification procedure. The experimental results illustrate the crop classification accuracy of this method, which achieves a 4.1% improvement over the traditional approach and is especially effective for dryland crops (e.g. corn and rapeseed). Thus, this research demonstrates the effectiveness of the combined use of optical and SAR data for similar applications in cloudy/rainy mountainous areas.
更多查看译文
关键词
cloudy,rainy mountainous area,crop classification,multitemporal data,combined utilization,feature transfer,optimization
AI 理解论文
溯源树
样例
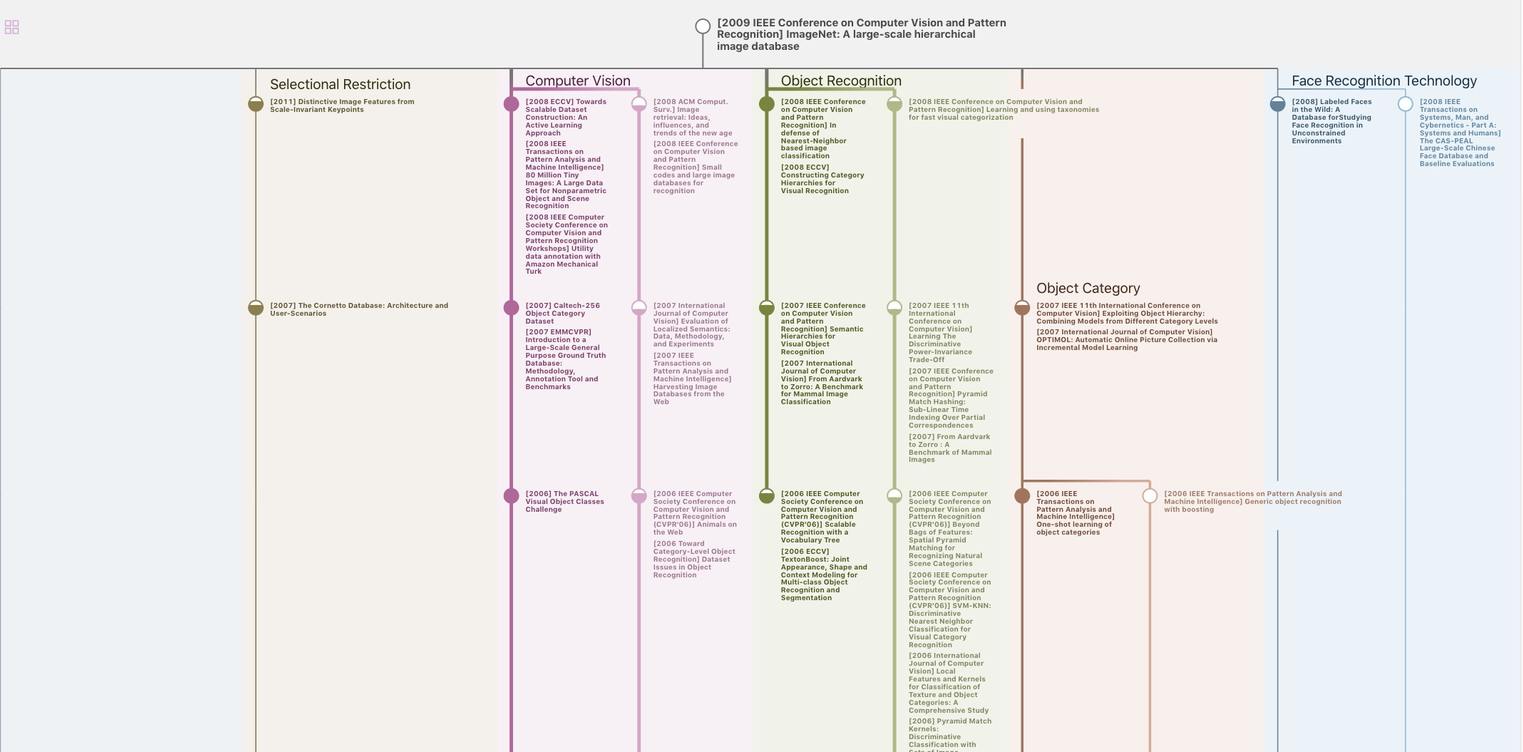
生成溯源树,研究论文发展脉络
Chat Paper
正在生成论文摘要