Ionosphere Tomographic Model Based on Neural Network with Balance Cost and Dynamic Correction Using Multi-Constraints
Atmosphere(2022)
摘要
A Neural network (NN) is a promising tool for the tomographic inversion of the ionosphere. However, existing research has adopted an unbalanced cost function for training purposes and a preset image for constraint purposes, resulting in the output image being dominated by measurements. To address these problems, we proposed an NN-based tomographic model with a balance cost function and a dynamic correction process (BCDC) for ionosphere inversion. The cost function is composed of two balance terms corresponding to the measurements and the selected constraints, respectively. The produced image in the forward process of the NN is corrected dynamically by fitting each vertical profile with orthogonal basis functions (EOFs) and the Chapman function and then by smoothing the voxels of each layer with a moving window approach horizontally. The corrected image is then used to calculate the slant total electron content (STEC) parameter, which is further translated into the term of the cost for the vertical and horizontal constraints. Experiments were carried out to validate the BCDC method and compared with a recently developed tomographic method and the international reference ionosphere (IRI) model. Results show that the parameters derived from the BCDC model demonstrate good consistency with the observations. Comparing with the reference methods, the BCDC method performs better in the validations of vertical profiles, F2 layer peak density (NmF2), STEC parameter and vertical total electron content map. Further analysis also shows that a balance cost function is of benefit to achieve an image of better quality.
更多查看译文
关键词
ionospheric tomography,neural network,empirical orthogonal function,Chapman function,smoothing constraint
AI 理解论文
溯源树
样例
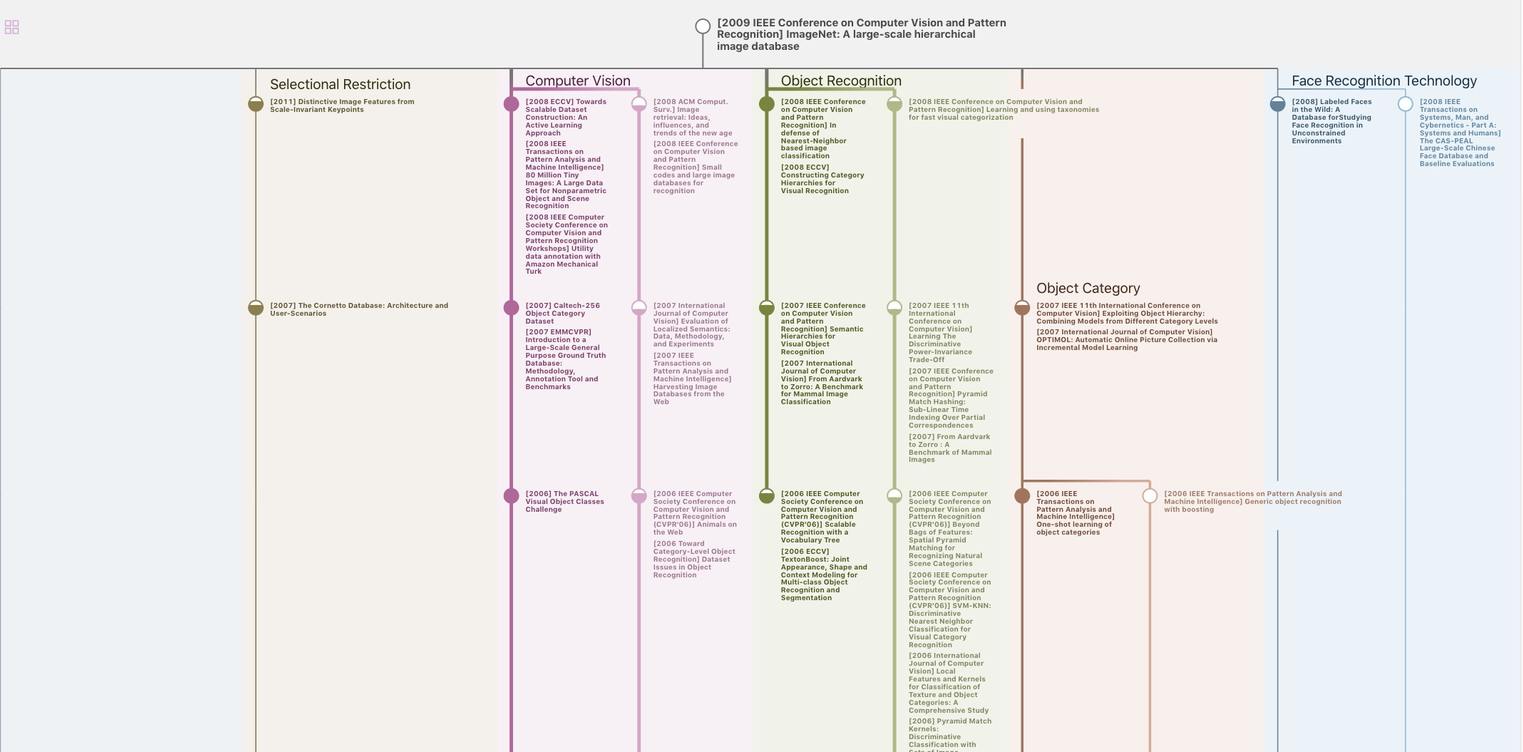
生成溯源树,研究论文发展脉络
Chat Paper
正在生成论文摘要