Using logistic regression model selection towards interpretable machine learning in mineral prospectivity modeling
Geochemistry(2021)
摘要
Regression or regression-like models are often employed in mineral prospectivity modeling, i.e., for the targeting of resources, either based on 2D map images or 3D geomodels both in raster mode or based on spatial point processes. Machine learning techniques like artificial neural networks are often applied and give decent results in the prediction of target events. However, they typically provide little insight into the problem regarding the importance, or relevance, of covariables. On the other hand, logistic regression has a well understood statistical foundation and uses an explicit model from which knowledge can be gained about the underlying phenomenon. Establishing such an explicit model is rather difficult for real world problems. In the context of mineral prospectivity modeling additional challenges arise, such as rare events, i.e. only a small fraction of data instances describes a positive target event, which is the event of interest.
更多查看译文
关键词
Mineral prospectivity modeling,Logistic regression,Model selection,Machine learning
AI 理解论文
溯源树
样例
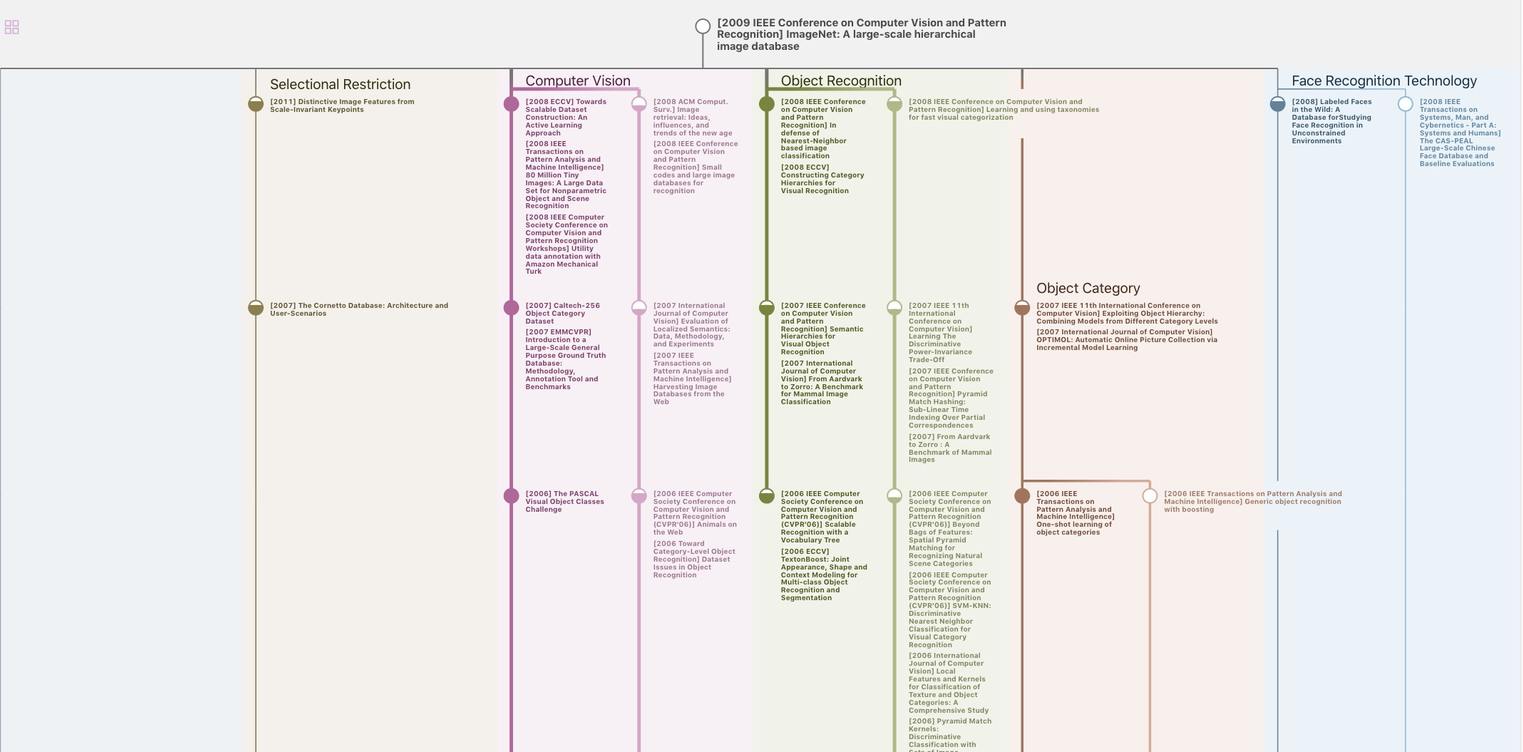
生成溯源树,研究论文发展脉络
Chat Paper
正在生成论文摘要