Predicting Temperature Field in Powder-Bed Fusion (PBF) Additive Manufacturing Process Using Radial Basis Neural Network (RBNN)
THERMOMECHANICS & INFRARED IMAGING, INVERSE PROBLEM METHODOLOGIES, MECHANICS OF ADDITIVE & ADVANCED MANUFACTURED MATERIALS, AND ADVANCEMENTS IN OPTICAL METHODS & DIGITAL IMAGE CORRELATION, VOL 4(2022)
摘要
Avoiding or eliminating thermal abnormalities in powder bed fusion (PBF) is critical since the abnormalities can lead to a higher failure rate of printing complex parts, a longer manufacturing lead time, and/or additional post-processing. Controlling the thermal evolution of the process can hinder or minimize some of the most frequently encountered thermal abnormalities. To achieve such an objective, the prediction and control of temperature distribution throughout an exposure layer is a crucial step. The generation of uniform temperature distribution throughout the printed layers and the avoidance of overheated zones are two primary sub-objectives for controlling the thermal evolution of the process. However, the complex and non-linear nature of the process has limited the ability to derive a universal analytical equation to correlate the process parameters with the thermal distribution of a printed layer. Laser specifications such as laser power and scanning speed are among the main process parameters that predominantly govern the temperature distribution throughout the layer. In this paper, we employ an artificial neural network (ANN) to correlate laser power with the temperature of the printed area around the melt pool in Inconel 718. In our first variant, we investigate the effectiveness of using the multilayer perceptron Radial Basis Neural Network (RBNN) to model the function for predicting the temperature distribution for various laser power. We use the Rosenthal equation to generate adequate inputs-outputs for training our function. We then compare the output with the simulation results for five different laser powers. The results show that the function was trained successfully with a low mean square root error of 9.7157 using 2000 samples, a wider gap exists between the trained function and the simulated data. In the second variant, we use a recurrent neural network (RNN), which enables temporal histories to be used for training. To fulfill such objective, we acquire real thermal data using a photon-counting IR camera for different printed layers. This step allows the training of a function to predict the temperature distribution precisely for different laser power and thermal history. As future work, we will employ the function to adjust the laser power to minimize the overheated zones and distribute the temperature uniformly throughout each exposure layer.
更多查看译文
关键词
Powder bed fusion process, Artificial neural network, FBFN neural network, Temperature prediction, Adjusting laser power
AI 理解论文
溯源树
样例
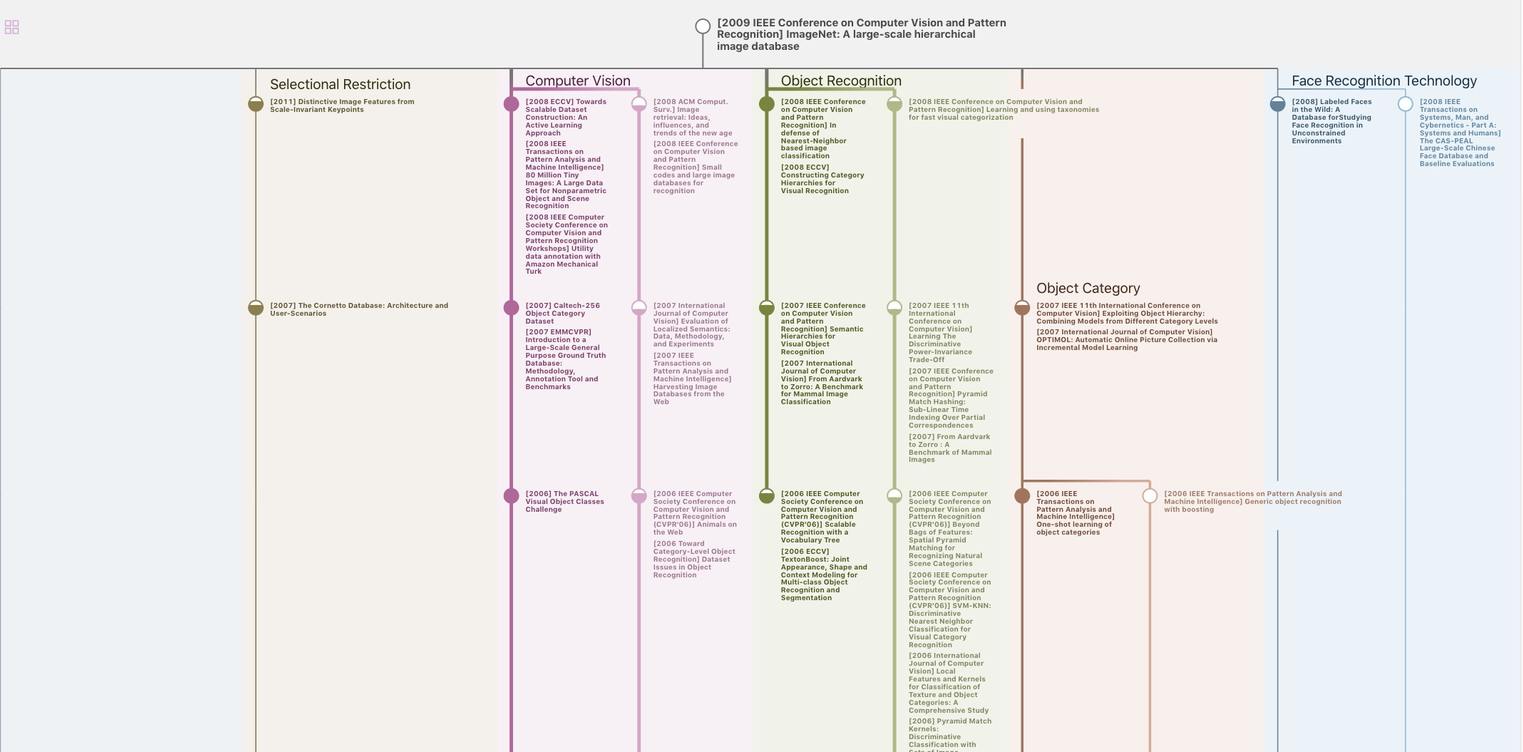
生成溯源树,研究论文发展脉络
Chat Paper
正在生成论文摘要