Cataract grading method based on deep convolutional neural networks and stacking ensemble learning
INTERNATIONAL JOURNAL OF IMAGING SYSTEMS AND TECHNOLOGY(2022)
摘要
The cataract is the most common cause of severe vision impairment or blindness worldwide. A periodical diagnosis is recommended in order to prevent cataract severity, where screening might be feasibly ensured through fundus images. In this paper, we propose a CAD system to efficiently grade the cataract from fundus images. For such a need, an ensemble learning framework is put forward where the knowledge of three convolutional deep neural networks is stacked in order to perform an efficient cataract prediction. The main contributions of this work are given as follows: (1) Preprocessing and data augmentation of fundus images are carried out to ensure the robustness of the cataract grading; (2) The well-known DL architectures (Inception-V3, MobileNet-V2 and NasNet-Mobile) are fine-tuned and learned as base classifiers; (3) An Extreme Learning Machine (ELM) is performed as a meta classifier to stack the predictions of base classifiers. The evaluation is conducted using a dataset of 590 fundus images selected from two public databases. The suggested framework achieves 94.07% accuracy, 94.37% sensitivity, 93.62% specificity, 95.71% precision and 95.04% F-measure for cataract grading. The experimentation proves that the proposed framework successfully grades fundus images into cataract severity. Moreover, stacking ensemble learning allows achieving a performance that significantly surpasses the ones realized by each DL architecture, applied separately.
更多查看译文
关键词
cataract, deep learning, ensemble learning, fundus images
AI 理解论文
溯源树
样例
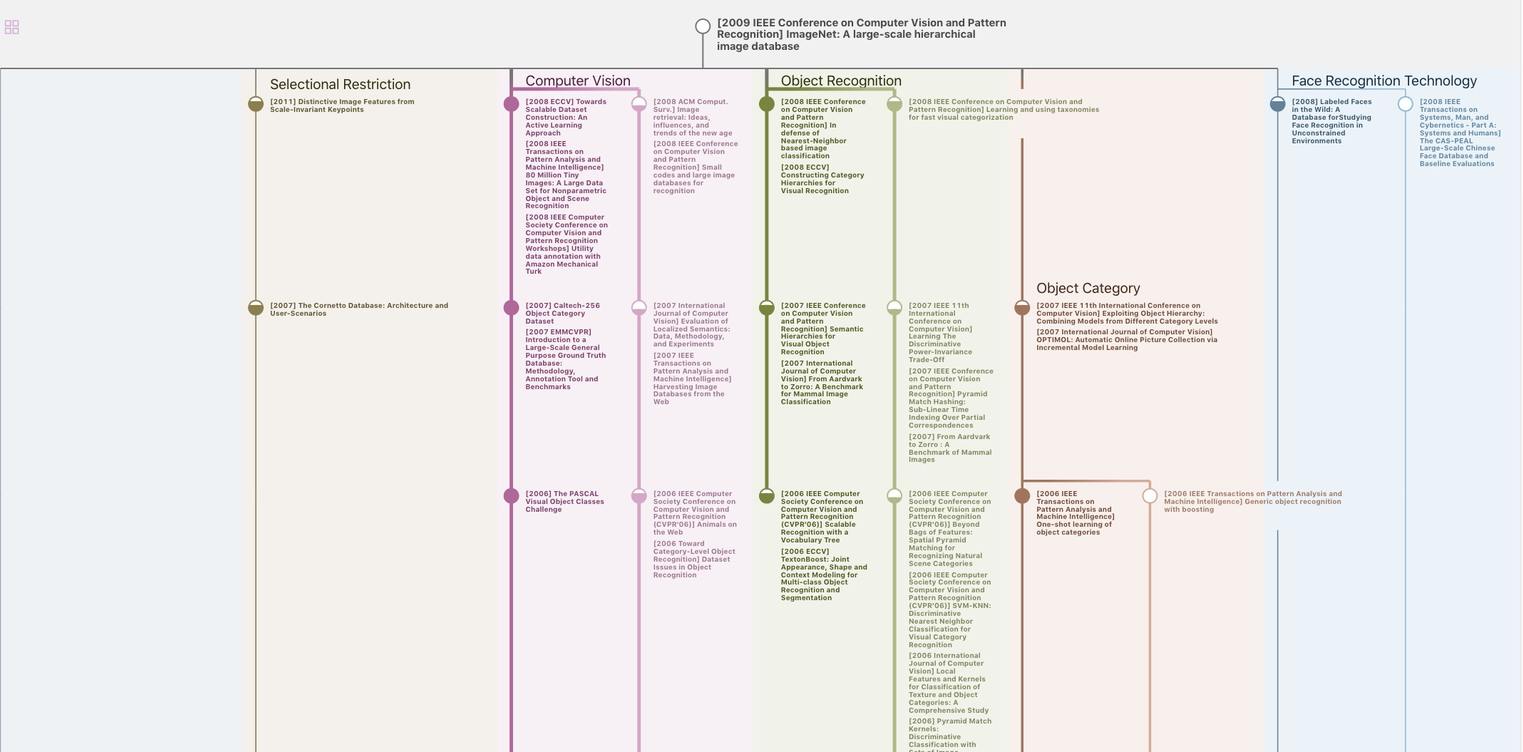
生成溯源树,研究论文发展脉络
Chat Paper
正在生成论文摘要