A comparative study of optical flow methods for fluid mechanics
EXPERIMENTS IN FLUIDS(2021)
摘要
We present a benchmark study of Optical Flow (OpF) methods for fluid mechanics applications. It is aimed at assessing the performance of three OpF methods, Lucas and Kanade (in: Proceedings of the 7th 1519 international joint conference on artificial intelligence, 1981), Horn and Schunck (AI 17:185–203, 1981) and Farnebäck (Two-frame motion estimation based on polynomial expansion, in: Bigun, Gustavsson (eds) Image analysis, Springer, 2003), combined or not with the Liu and Shen (JFM 614:253–291, 2008) algorithm. The performance of the OpF methods, evaluated exclusively as the difference between the values of the methods and of ground-truth (reference values), is benchmarked for deformation dominated, rotation-dominated and uniform flows. For each flow type, relative and absolute errors are computed for different tracer displacements, noise levels, pixel particle sizes, image bit-depths and particle concentrations. The accuracy of the OpF methods seems mainly affected by the magnitude of velocity gradients and convective accelerations. It does not seem to be affected by the relative preponderance of rotational and deformation components. The inner region of the Poiseuille flow and the saddle point in the Rankine vortex combined with uniform flow pose significant difficulties to all methods. The performance of the Lucas-Kanade/Liu-Shen combination is the best for all flow types, image conditions and image bit depths. The Farnebäck/Liu-Shen combination has a similar high performance but only for image depths of 10 bit or higher. These OpF methods maintain a high performance for tracer sizes and concentrations outside the PIV optimal range. Horn-Schunck is the worst performing method, due to high sensitiveness to particle concentration variations or particle sizes. These results can be used to plan new particle-based velocimetry experiments or to retrieve further information from existing PIV databases. Graphical abstract
更多查看译文
AI 理解论文
溯源树
样例
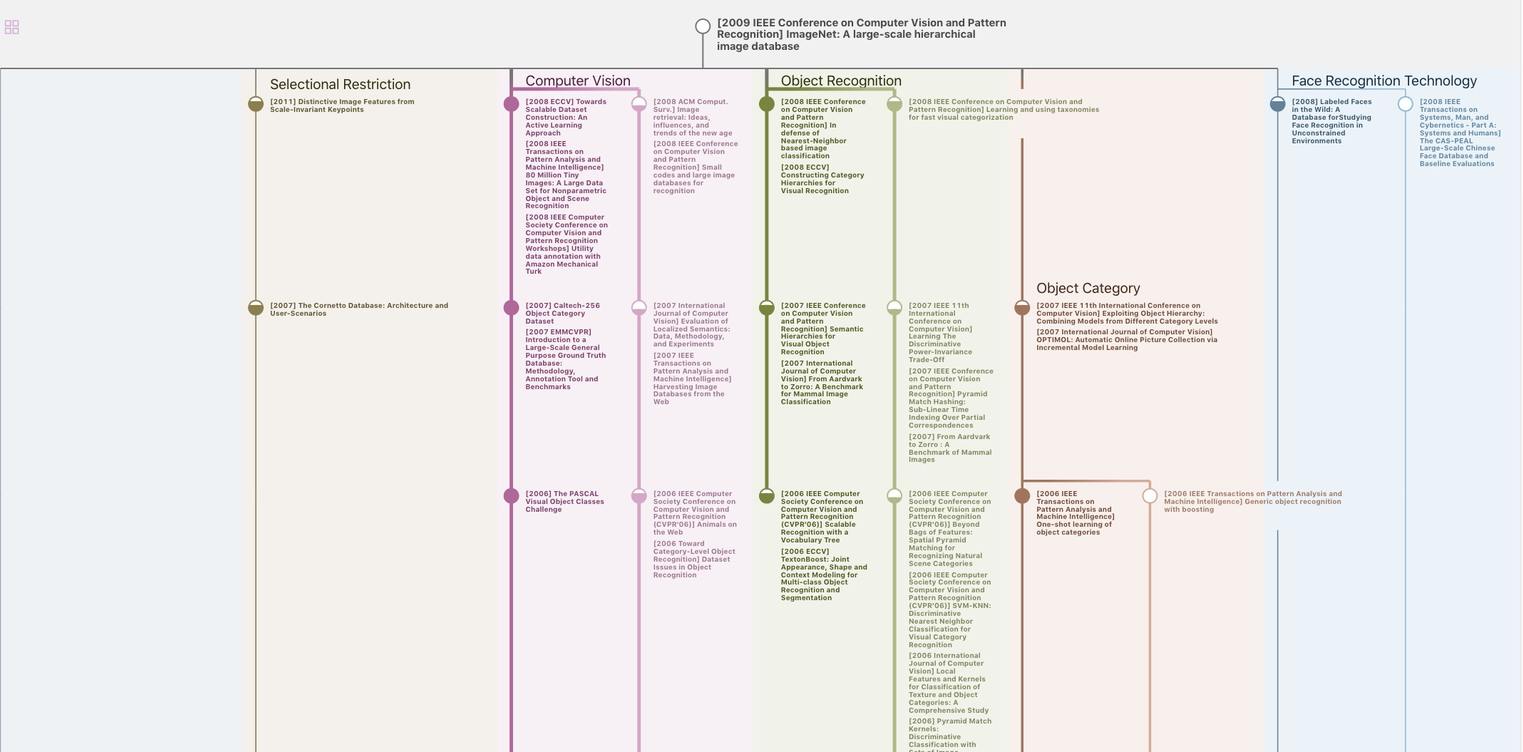
生成溯源树,研究论文发展脉络
Chat Paper
正在生成论文摘要