Application of machine learning in rapid analysis of solder joint geometry and type on thermomechanical useful lifetime of electronic components
MECHANICS OF ADVANCED MATERIALS AND STRUCTURES(2023)
摘要
Rapid reliability analysis of the solder joints in the electronic devices identifies as an appeared gap in the design stage. This paper demonstrates the feasibility of employing the neural network in the solder joint useful lifetime estimation. A dataset is prepared for training a deep neural network under different conditions using finite element simulations. The contributory parameters including thermal specifications, solder joint geometry and solder joint type are considered in 420 finite element simulations. While the proposed approach has a remarkably quick reliability assessment, the predicted results showed a maximum root mean square error of 3.2%. The results reveal that there exists an optimum solder joint volume in which the maximum useful lifetime under the certain thermal loading. Also, it is found that the hourglass type solder interconnection has better performance in comparison with barrel type solder interconnection as the reliability perspective owing to the more gentle triaxiality factor. Ability of rapid reliability analysis and effects consideration of key parameters on the solder joint reliability make this proposed method attractive in the design stage of electronic devices.
更多查看译文
关键词
Damage evolution, creep-fatigue failure mechanism, solder joint, neural network
AI 理解论文
溯源树
样例
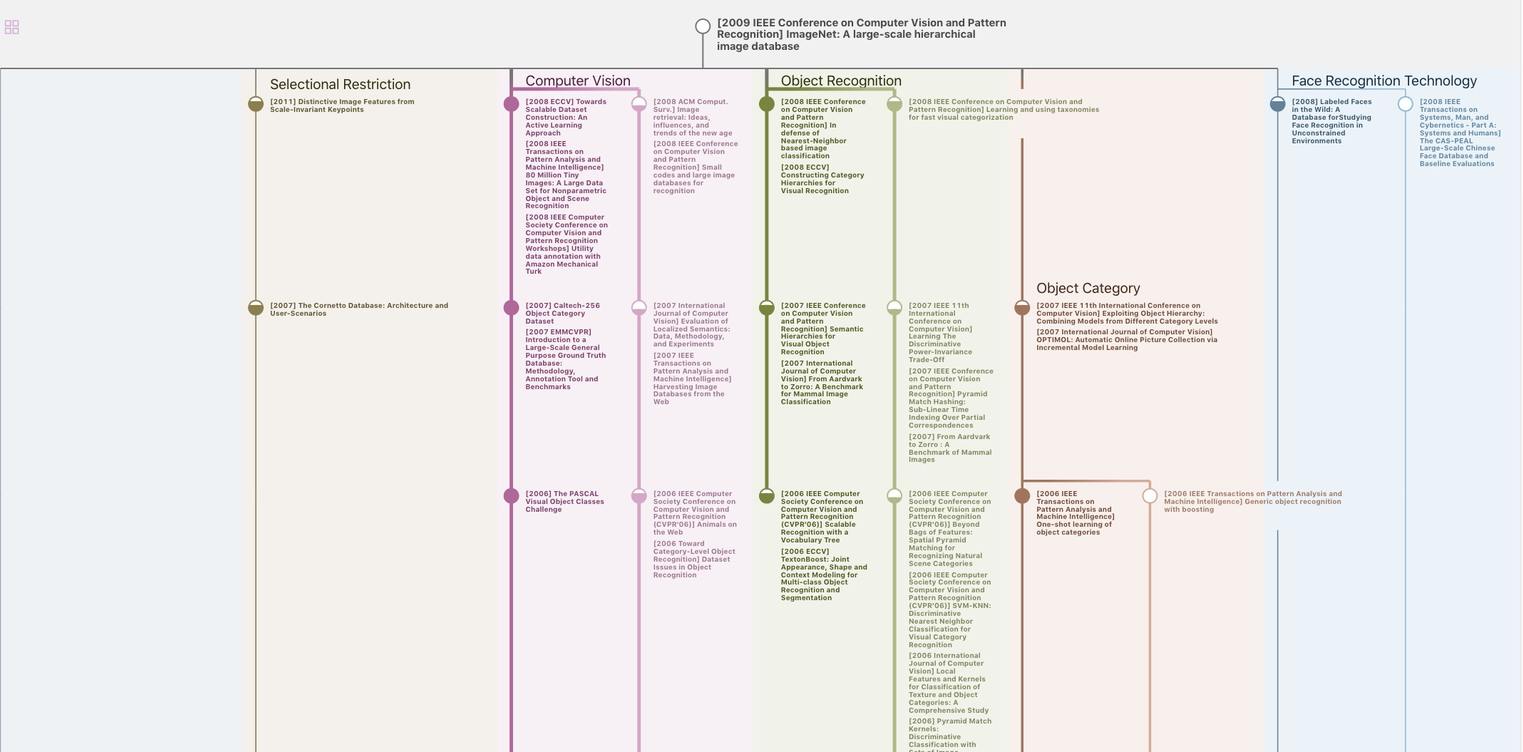
生成溯源树,研究论文发展脉络
Chat Paper
正在生成论文摘要