Multiscale deep network for compressive sensing image reconstruction
JOURNAL OF ELECTRONIC IMAGING(2022)
摘要
Deep learning-based image compressive sensing methods have received extensive attention in recent years due to their superior learning ability and fast processing speed. The majority of existing image compressive sensing neural networks use single-scale sampling, whereas multiscale sampling has demonstrated excellent performance compared to single-scale. We propose a multiscale deep network for compressive sensing image reconstruction that consists of a multiscale sampling network and a reconstruction network. First, we use convolution to mimic the linear decomposition of images, and the convolution is learned during the training process. Then a sampling network captures compressive measurements across multiple decomposed scales. The reconstruction network, which includes both the initial and enhanced reconstruction networks, learns an end-to-end mapping between the compressed sensing (CS) measurements and the recovered images of the network. Experimental results indicate that the proposed network framework outperforms the existing CS methods in terms of objective metrics, peak signal to noise ratio (PSNR), structural similarity index, and subjective visual quality. Specifically, at a 0.1 sampling rate, using 10 images for testing, and the average PSNR maximum (minimum) gain is 5.95 dB (0.25 dB). (C) 2022 SPIE and IS&T
更多查看译文
关键词
image compressive sensing, deep learning, multiscale, image decomposition, reconstruction network
AI 理解论文
溯源树
样例
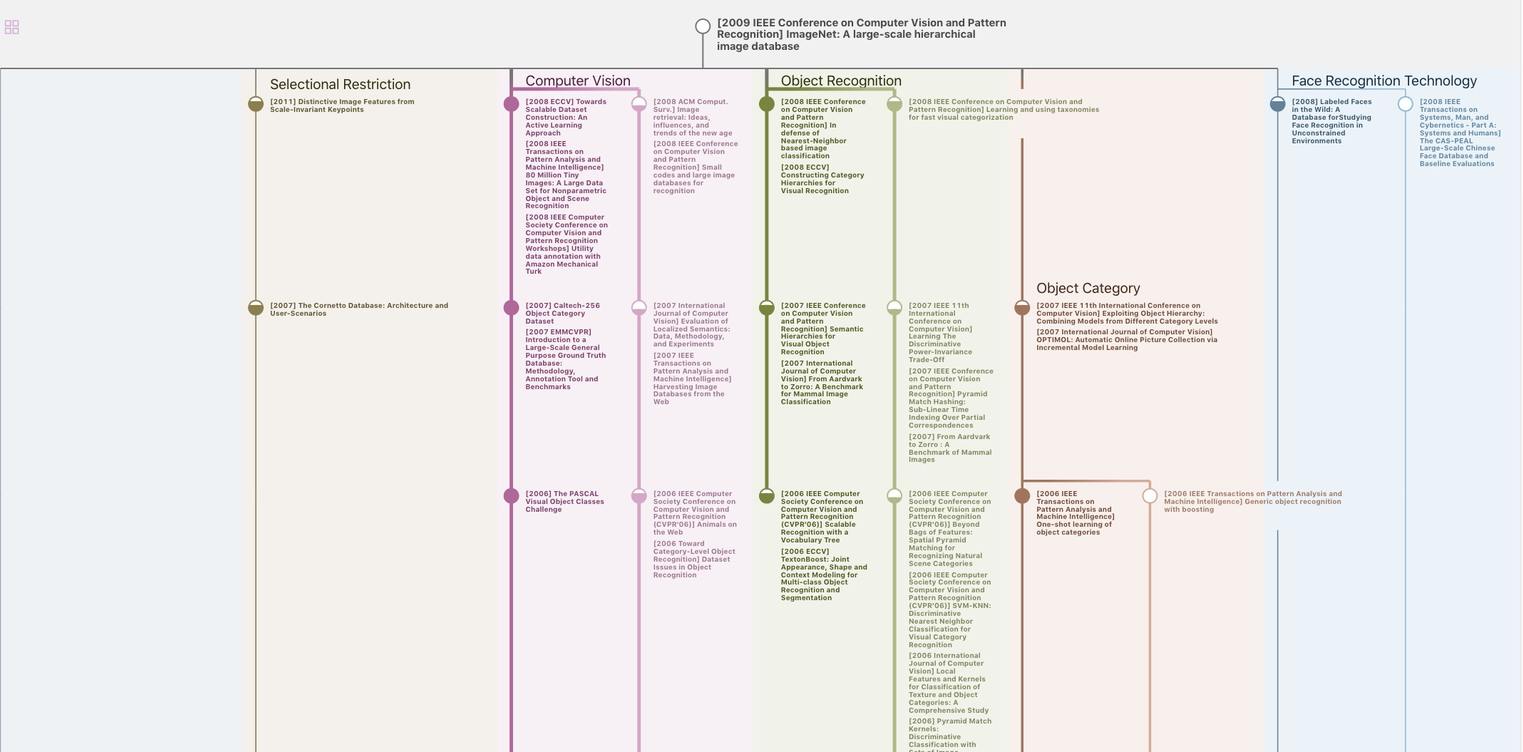
生成溯源树,研究论文发展脉络
Chat Paper
正在生成论文摘要