Predicting In-Field Flow Experiences Over Two Weeks from ECG Data: A Case Study
INFORMATION SYSTEMS AND NEUROSCIENCE (NEUROIS RETREAT 2021)(2021)
摘要
Predicting flow intensities from unobtrusively collected sensor data is considered an important yet challenging endeavor for NeuroIS scholars aiming to understand and support flow during IS use. In this direction, a limitation has been the focus on cross-subject models built on data collected in controlled laboratory settings. We investigate the potential of predicting flow in the field through personalized models by collecting report and ECG data from a clerical worker over the course of two weeks. Results indicate that a lack of variation in flow experiences during this time likely diminished these potentials. Through pre-training feature selection methods, model accuracies could be achieved that nonetheless approach related cross-subject flow prediction work. Novel recommendations are developed that could introduce more flow variation in future flow field studies to further investigate the within-subject predictability of flow based on wearable physiological sensor data.
更多查看译文
关键词
Flow experience, Field study, ECG, LASSO, Random forest
AI 理解论文
溯源树
样例
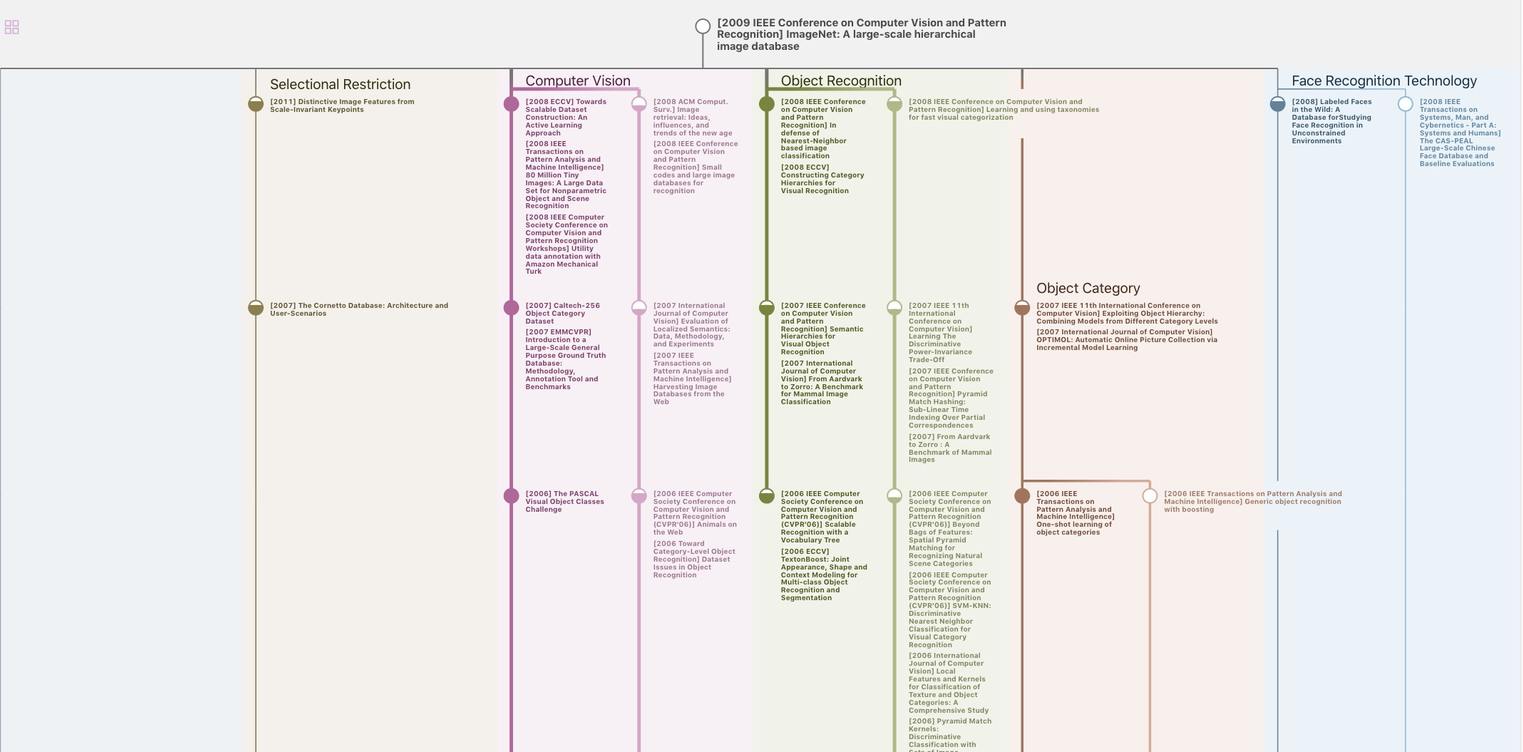
生成溯源树,研究论文发展脉络
Chat Paper
正在生成论文摘要