Spatio Temporal Sparsity in Homicide Prediction Models
IEEE ACCESS(2022)
摘要
Homicide prediction is a challenging task due to the spatio-temporal sparsity of these crime events. In this paper we report the results of using several approaches to mitigate this sparsity condition in machine learning models specially tailored towards modeling homicides events. Since spatial resolution is a direct determinant of sparsity, we focus on the performance of these models across different resolutions of interest to police authorities. We use a simple count model as benchmark and propose some enhancements of it directed towards improving prediction performance. We then compare the results to more complex models motivated by manifold learning and graph signal processing methods. We found that the simple benchmark models are as good as state of the art models for low resolution, but, as resolution increases, the performance of machine learning models outperform the benchmark. These results provide a rationality for the use of state of the art machine learning models for homicide prediction at the high resolution of interest for the deployment of police resources.
更多查看译文
关键词
Predictive models, Urban areas, Spatial resolution, Data models, Training, Signal resolution, Law enforcement, Crime prediction, homicides, signal processing, kernel warping, spatio-temporal sparsity
AI 理解论文
溯源树
样例
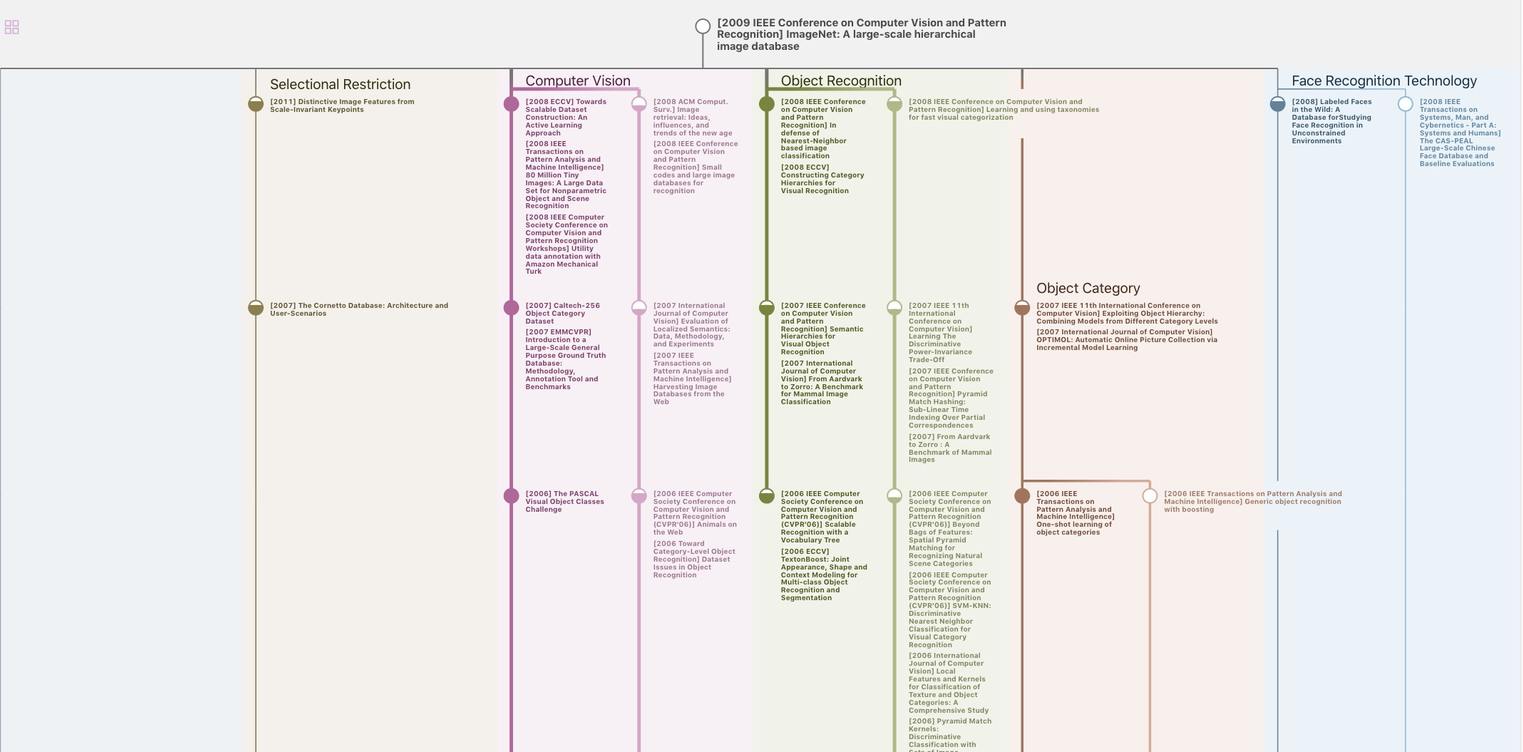
生成溯源树,研究论文发展脉络
Chat Paper
正在生成论文摘要