Physics-guided and Neural Network Learning-based Sliding Mode Control
IFAC PAPERSONLINE(2021)
摘要
This paper presents a physics-guided neural network learning fused with a sliding mode control design approach for closed-loop model identification of nonlinear systems. In particular, physical knowledge of Lagrangian for mechanical systems is incorporated into neural networks to facilitate the learning of equations of motion from data. A sliding mode controller design is considered that uses the identified model and further refines the model online by an updating law determined from the Lyapunov analysis. The proposed approach is shown to be more efficient and targeted for identification than existing Lagrangian-based neural network approaches. Simulation results on a two-joint manipulator and a three-joint manipulator demonstrate that the proposed learning-based approach can achieve better performance of model identification and improved tracking control with less computational cost compared to learningbased control without online fine-tuning. Copyright (C) 2021 The Authors.
更多查看译文
关键词
Physics-guided learning, Neural networks, Sliding mode control, Online learning
AI 理解论文
溯源树
样例
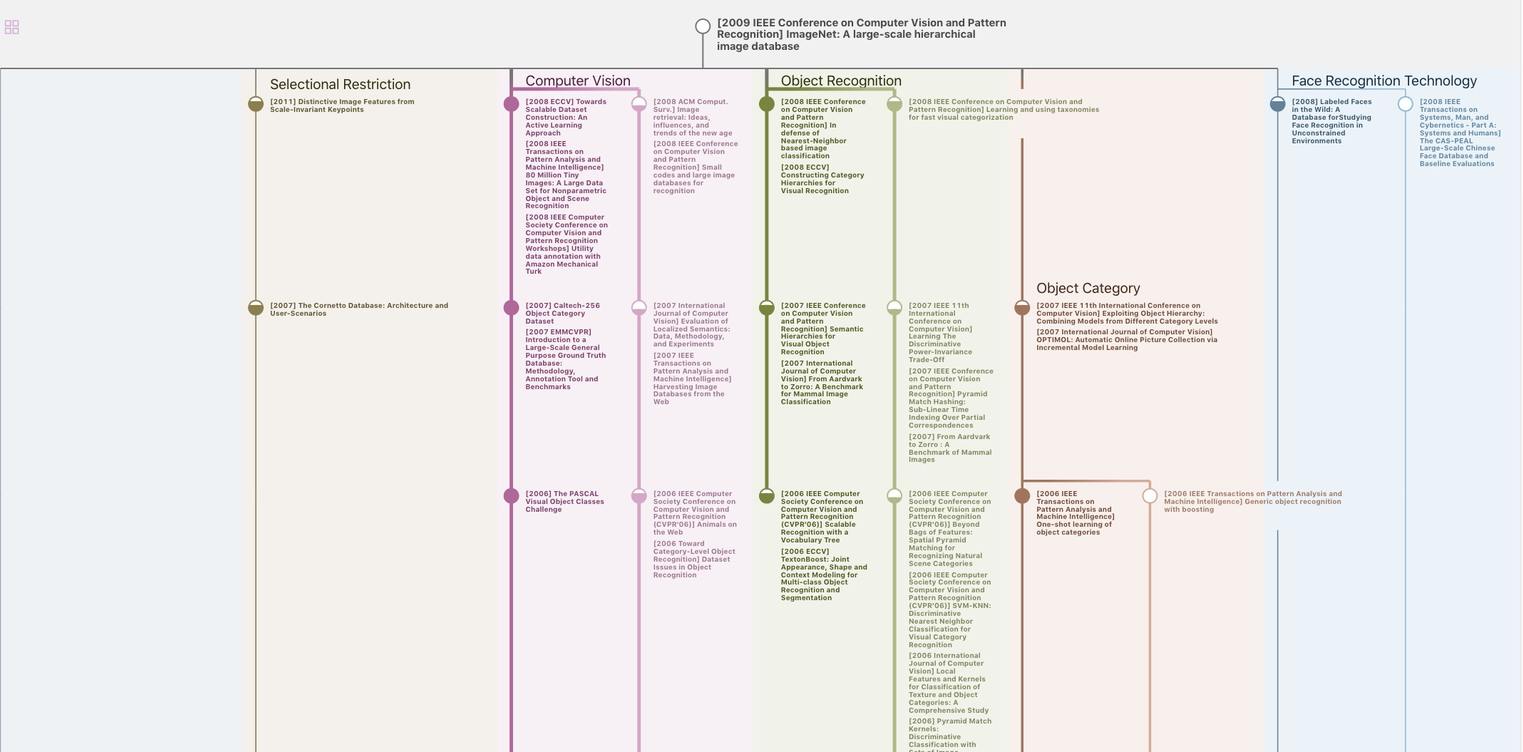
生成溯源树,研究论文发展脉络
Chat Paper
正在生成论文摘要