Actin Cytoskeleton Morphology Modeling Using Graph Embedding and Classification in Machine Learning
IFAC-PapersOnLine(2021)
摘要
Actin cytoskeleton modeling and quantification are essential in studying the dynamics of cellular mechanotransduction. However, current approaches to actin cytoskeleton quantification are limited in terms of both efficiency and accuracy. In this paper, we propose to model the cellular actin cytoskeleton morphology using the graph to vector embedding technique together with the neural network (NN) classification in machine learning. The proposed model consists of a skip-gram model followed by a fully connected classifier. The actin cytoskeleton morphology is modeled based on both the structure and node features extracted from the cytoskeleton images. Specifically, the embedding tool outputs the embedded vectors of the cytoskeleton graphs, and then the embedded vectors are used by the fully connected layer to perform cytoskeleton classification. In this work, we demonstrate the classification accuracy of the proposed framework using actin cytoskeleton images from cells treated by Latrunculin B (an actin depolymerizer) at different concentrations. The actin cytoskeleton morphology corresponding to each treatment concentration is defined as a class (e.g., actin depolymerization level). The final classification result is showed an accuracy of 85.3%.
更多查看译文
关键词
Graph to vector embedding,Skip-gram model,Classification,Machine learning,Actin cytoskeleton
AI 理解论文
溯源树
样例
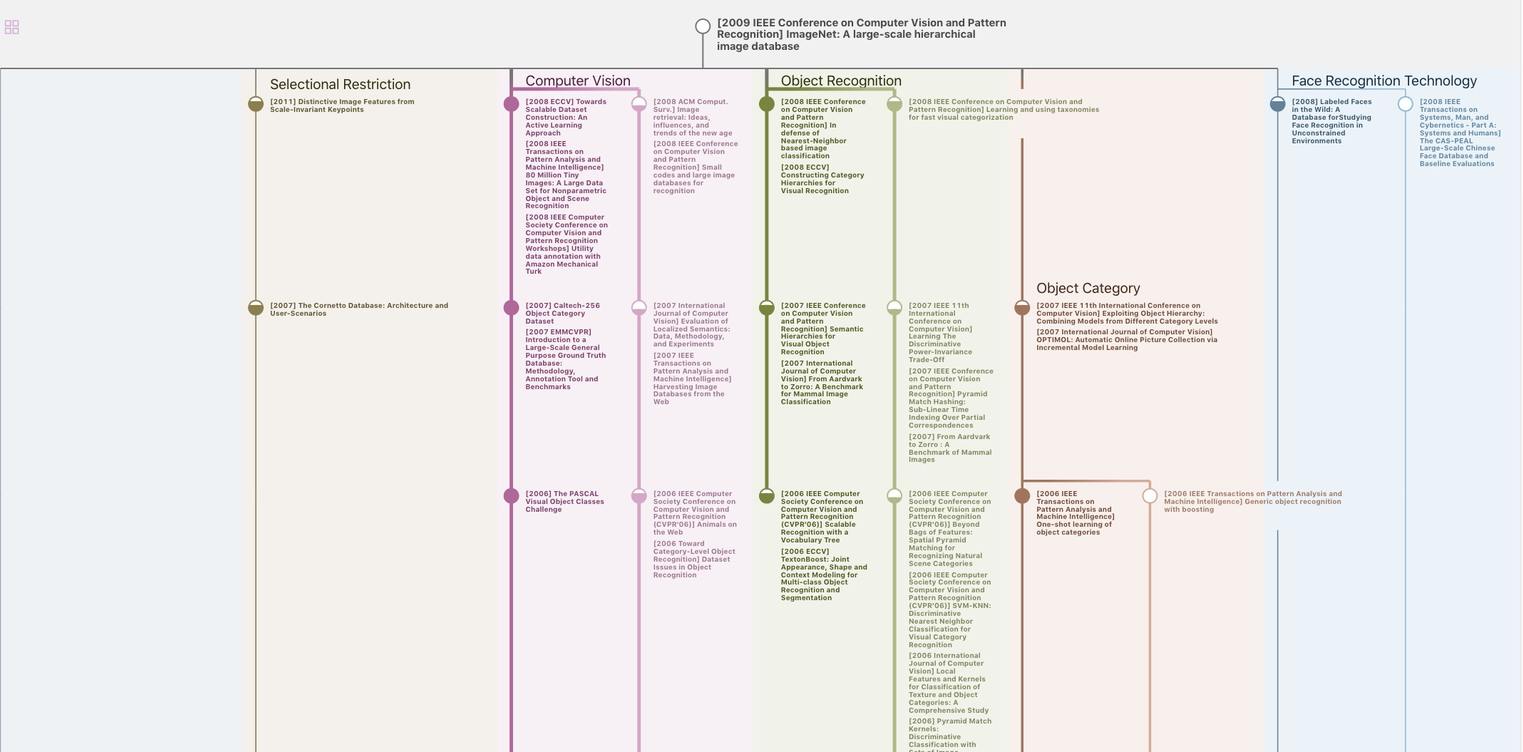
生成溯源树,研究论文发展脉络
Chat Paper
正在生成论文摘要