A Spatiotemporal and Multivariate Attribute Correlation Extraction Scheme for Detecting Abnormal Nodes in WSNs
IEEE access(2021)
摘要
Many heterogeneous sensors exhibit strong spatio-temporal correlations that can be used to enhance the abnormal node detection problem in a wireless sensor network (WSN). Corruption in these correlations has been shown effective in detecting false data injection attacks. In this paper, we adopt a new cross-correlation-based method to extract the sensor relationships. It utilizes the observed spatiotemporal (ST) and multivariate-attribute (MVA) sensor correlations to decide whether the sensor is subject to abnormalities or represents actual events. Based on the ST correlations, the cross-correlation is extracted in both space and time by conducting shape-based logical subclustering and two-phase analysis methods. In the first analysis phase, the system uses a variable-size sliding window and a median absolute deviation (MAD) measure. If the collected sensor data streams output a certain percentage of anomalous points, the MAD will flag these points as anomalous measurements, and all the sensor data and the window size will be passed to the second analysis phase. The latter performs both tumbling-window and sliding-window analyses to extract multicriteria cross-correlation measures. Finally, all the extracted sensor time-series features will be fed to the shape-based clustering to generate a sensor similarity-like graph. The latter reflects the similarity degree of the sensor with the other nodes. The nodes with a low similarity degree below the threshold will be identified as candidate abnormal nodes. Based on the observed MVA correlations, a set consisting of a few rules is introduced to check whether the detected candidate abnormal nodes represent actual events. Finally, if abnormal nodes exist, then such nodes are reported. Our experiments using two real-world datasets demonstrate that our proposed approach detects abnormal nodes with an average accuracy of 96.50%, an average precision of 88.69%, and a recall rate of 93.00%.
更多查看译文
关键词
Anomaly detection,Internet of Things,sensor data correlations,wireless sensor networks,false data injection attacks
AI 理解论文
溯源树
样例
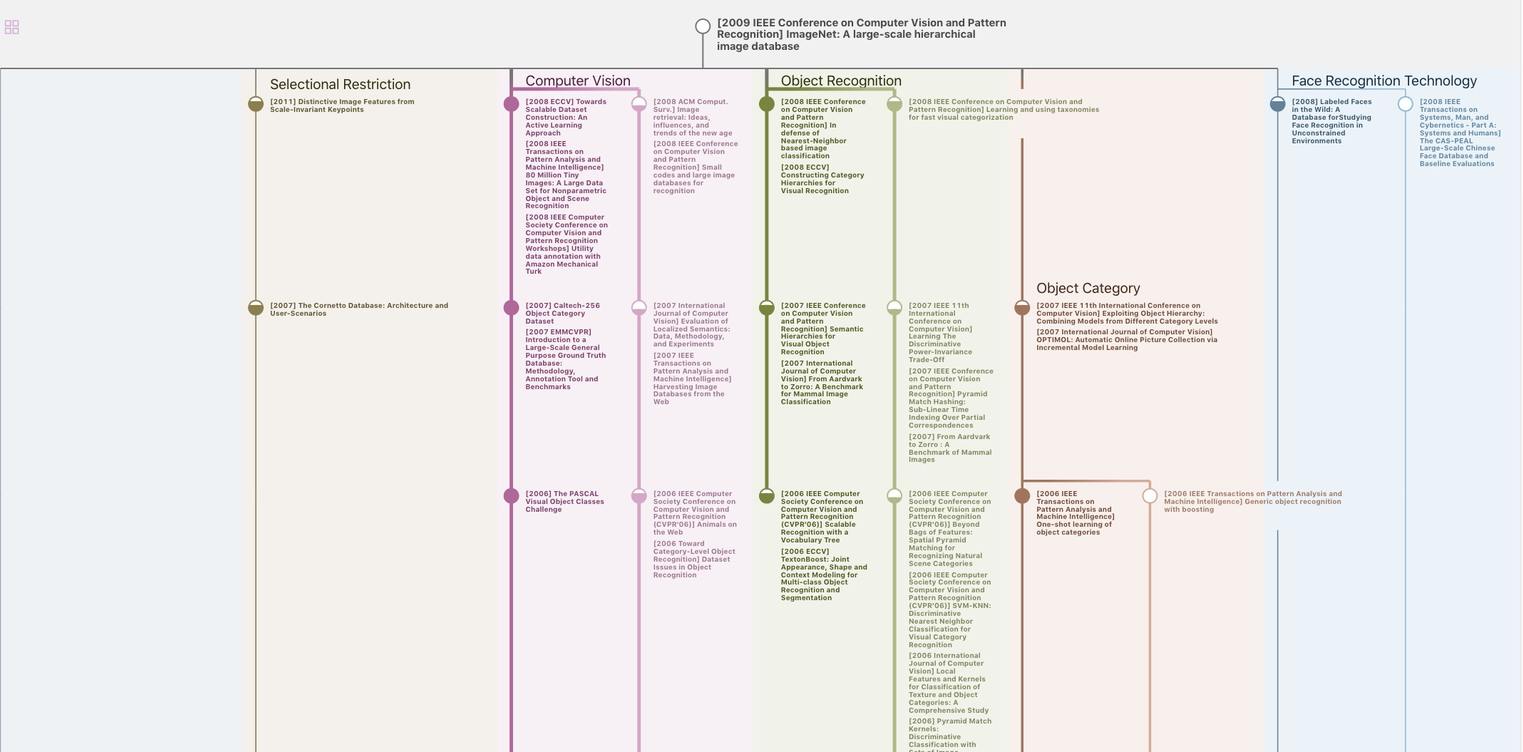
生成溯源树,研究论文发展脉络
Chat Paper
正在生成论文摘要