Yield and Quality Prediction of Winter Rapeseed-Artificial Neural Network and Random Forest Models
AGRONOMY-BASEL(2022)
摘要
As one of the greatest agricultural challenges, yield prediction is an important issue for producers, stakeholders, and the global trade market. Most of the variation in yield is attributed to environmental factors such as climate conditions, soil type and cultivation practices. Artificial neural networks (ANNs) and random forest regression (RFR) are machine learning tools that are used unambiguously for crop yield prediction. There is limited research regarding the application of these mathematical models for the prediction of rapeseed yield and quality. A four-year study (2015-2018) was carried out in the Republic of Serbia with 40 winter rapeseed genotypes. The field trial was designed as a randomized complete block design in three replications. ANN, based on the Broyden-Fletcher-Goldfarb-Shanno iterative algorithm, and RFR models were used for prediction of seed yield, oil and protein yield, oil and protein content, and 1000 seed weight, based on the year of production and genotype. The best production year for rapeseed cultivation was 2016, when the highest seed and oil yield were achieved, 2994 kg/ha and 1402 kg/ha, respectively. The RFR model showed better prediction capabilities compared to the ANN model (the r(2) values for prediction of output variables were 0.944, 0.935, 0.912, 0.886, 0.936 and 0.900, for oil and protein content, seed yield, 1000 seed weight, oil and protein yield, respectively).
更多查看译文
关键词
rapeseed, yield, oil content, mathematical modelling, artificial neural network, random forest
AI 理解论文
溯源树
样例
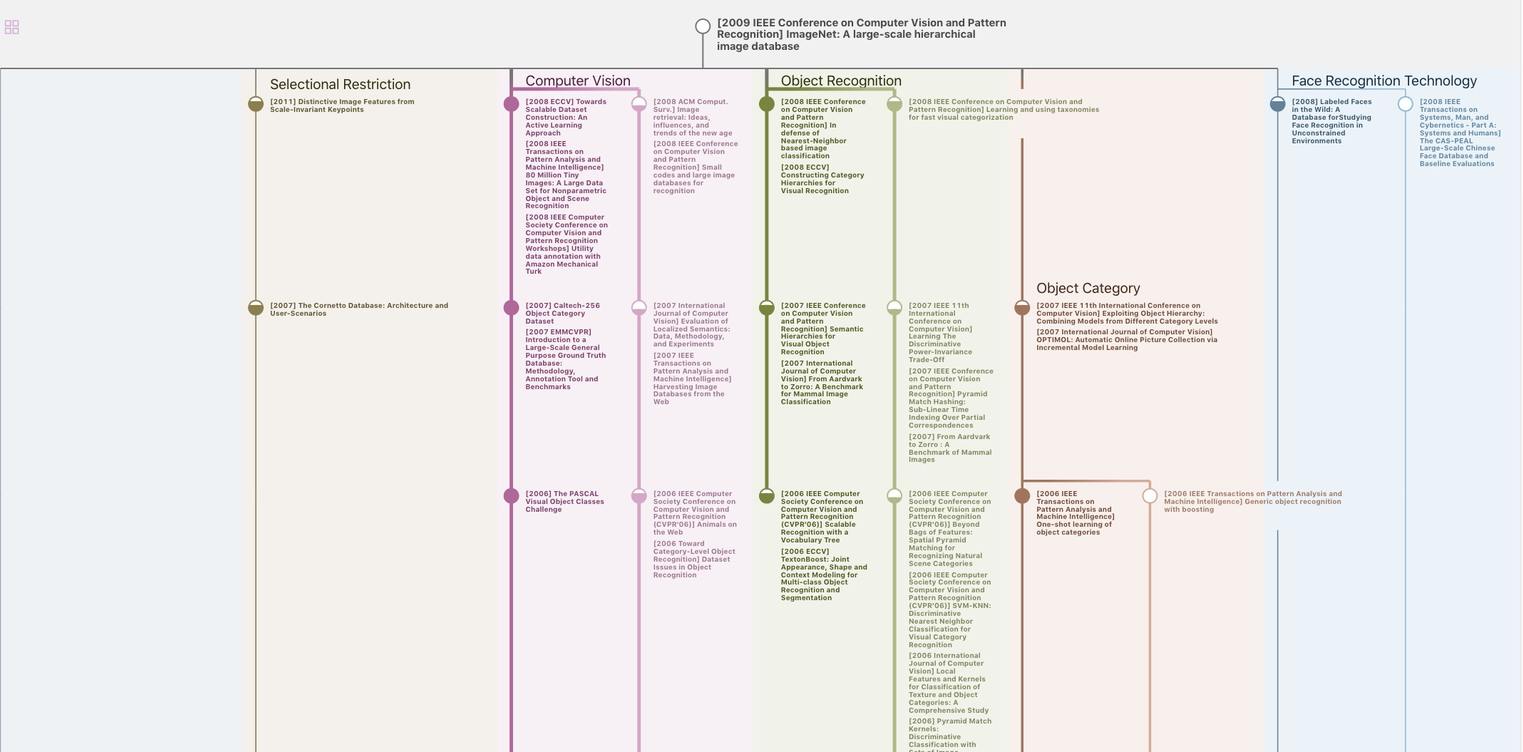
生成溯源树,研究论文发展脉络
Chat Paper
正在生成论文摘要